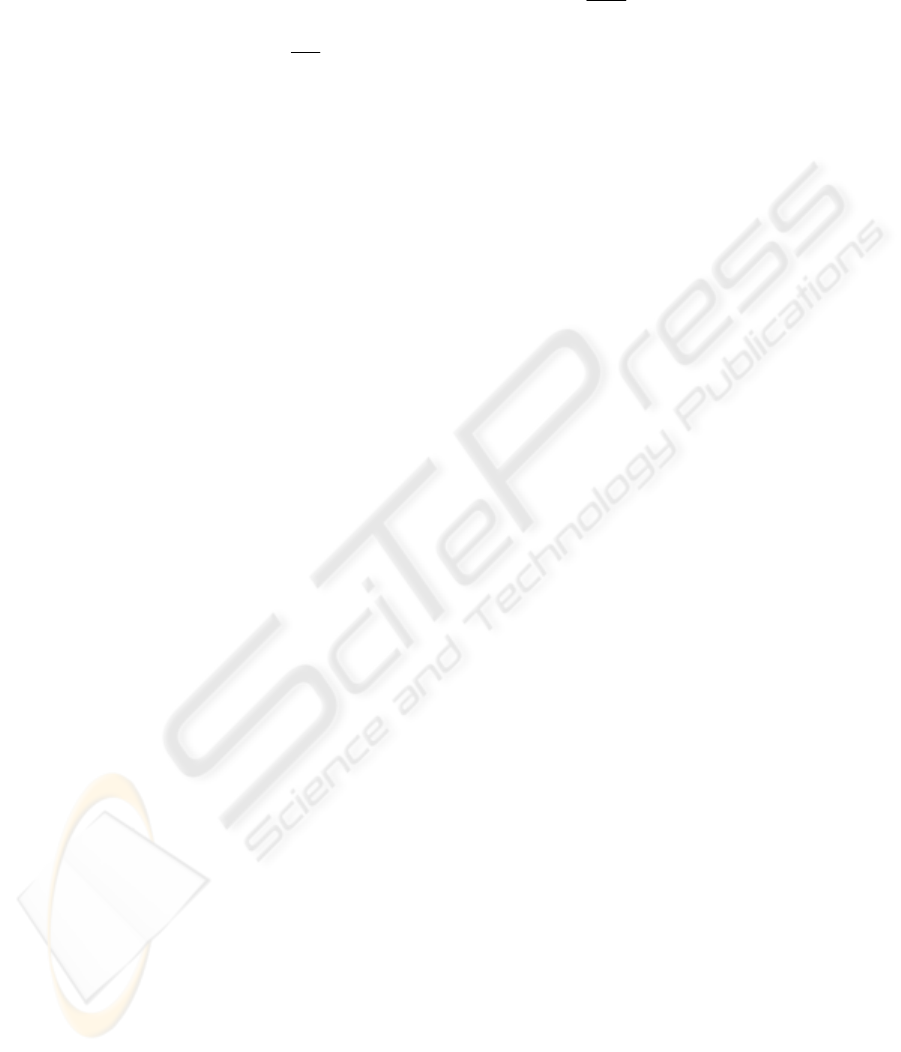
the Fisher test at different values of the confidence levels α%. When this level
increases, the number of significant factors and interactions increased, and thus the
number of inputs of the ANN increases. Average learning
MSE which express the
average error between the training data and the corresponding predicted values and
the generalization error
Eg
which express the deviation from the testing data have
shown the existence of a critical set of inputs offering the highest prediction capability
of the developed ANNs. By using this approach, substantial improvements of the
prediction capability of the ANNs could be realized comparatively with the prediction
ability of the quadratic models developed using the DoE. On the other hand the
developed ANNs have shown better capability comparatively with the commonly
used structures, which use the DoE factors as inputs.
It was also remarked that the developed ANNs present higher sensibility to the input
variations than the DOE as they can distinguish between particular phenomena
occurring at low and high work speeds. At last, problems related to the minimal
negative predicted value, calculated by using models established with DOE method
could be solved as ANNs respects the output sign.
References
1. H.K. Tönshoff, J. Peters, I. Inasaki, T. Paul, Modelling and Simulation of Grinding Process,
Annals of the CIRP vol. 41, 2, (1992) 677-688.
2. Y. S. Lio, J. T. Huang, H.C. Su, A study on the machining-parameters optimization of wire
electrical discharge machining, J. Mater. Proc. Technol. 71 (1997) 487-493.
3. M. Thomas, Y. Beauchamp, Youssef, A. Youssef, An experimental design for surface
roughness and built-up edge formation in lathe dry turning, Int. J. of Quality Science, Vol.
2 (3), (1997) 167-180.
4. Y. A. Youssef, Comparison of a full factorial experiment to fractional and Taguchi design
in a lathe dry turning operation, Computers in ind. Engng., Vol. 27 (1-4), (1994) 59-62.
5. J. L. Lin, K.S. Wang, B.H. Yan, Y. S. Tarng, Optimization of the electrical discharge
machining process based on the Taguchi method with fuzzy logics, J. Mater. Proc.
Technol., 102 (2000) 48-55.
6. Y. Ali, L.C. Zhang, Estimation of Residual Stress Induced by Grinding Using a Fuzzy
Logic Approach, J. Mater. Proc. Technol. 63 (1997) 875-880.
7. Y.-H. Tsai, J. C. Chen, S.-J. Lou, An in-process surface recognition system based on neural
networks in end milling cutting operations, Int. J. Mach. Tools Manufact., Vol. 39, (1999)
583-605.
8. R. Azouzi, M. Guillot, On-line prediction of surface finish and dimensional deviation in
turning using neural network based sensor fusion, Int. J. Mach. Tools Manufact., Vol. 37
(9), (1997) 1201-1217.
9. T. Warren, L.J. Chen, A neural network approach for grinding process: Modeling and
optimization, Int. J. Mach. Tools Manufact., Vol. 34 (7), (1994) 919-937.
10. N. Zouaghi, Y. Ichida, N. Ben Fredj, N. Kimura, Grinding mode identification of silicon
carbide by using neural network, in: Proceeding of the third international conference on
progress of cutting and grinding Vol. III (JSPE, Osaka, 1996) 342-347.
11. K. M. Tsai, P. J. Wang, Predictions on surface finish in electrical discharge machining
based upon neural network models, Int. J. Mach. Tools Manufact., Vol. 41, (2001) 1385-
1403.
130