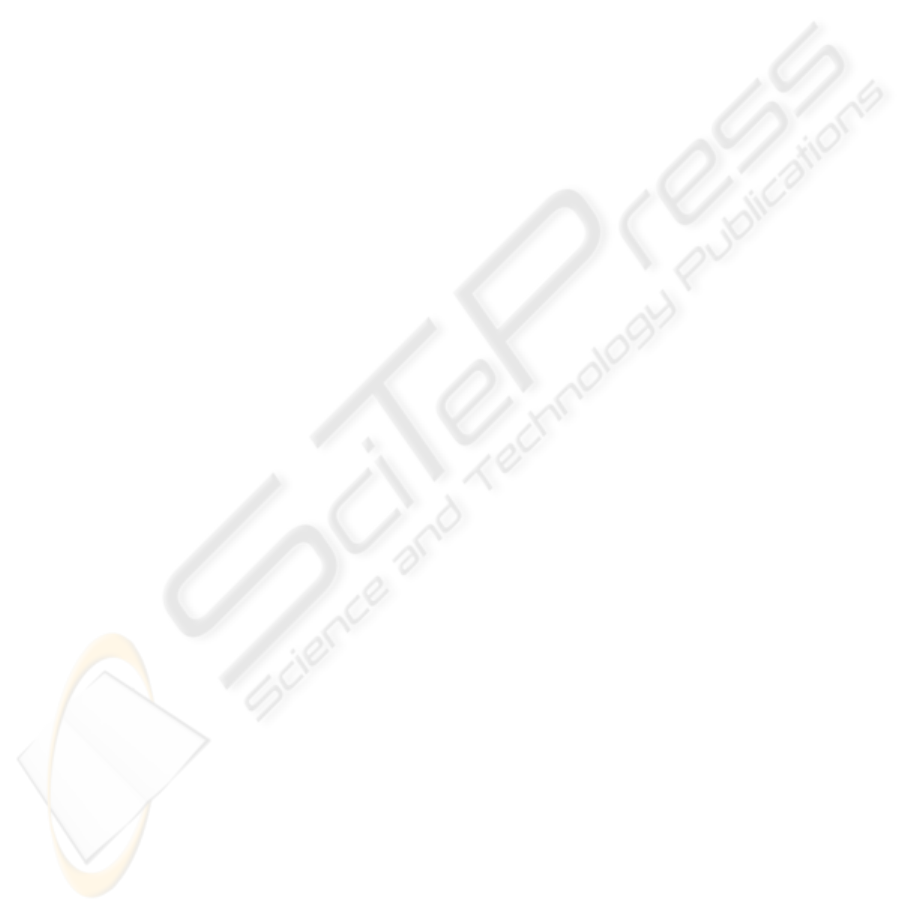
The same information can be obtained from the changes of the amide III band, and
from the intensity of the band on 901 cm
-1
.
5 Conclusion
When a skin sample is paraffinised, the direct analysis of recorded Raman spectra is
not possible because of the predominant intensity of paraffin spectrum over the other
compounds spectra. Thanks to the mutual independence of spectra of these species,
ICA is applicable and estimates physically meaningful sources.
A first conclusion is that paraffin spectrum can be decomposed in three
independent behavioural spectral bands. It means that the underlying tissue is more or
less reacting with some spectral bands of the paraffin spectrum. A second is that
melanin spectrum is not visible when paraffinised tissues are considered because of
the too energetic peaks of paraffin and CaF
2
. Third, more sources than suggested by
PCA must be employed in order to reveal the low energetic spectrum of keratin.
The last and important conclusion is that estimated keratin spectra of paraffinised
benign nevus and malignant melanoma contain a large amount of information. Little
spectral differences between these spectra lead to the identification of the kind of
analysed tissue. Molecular descriptors of the type of pathology have been found.
References
1. Gniadecka, M., Wulf, H., Mortensen, N., Nielsen, O. Christensen, D.: Diagnosis of basal
cell carcinoma by Raman spectra. Journal of Raman Spectroscopy, Vol. 28 (1997) 125–129
2. Sigurdsson, S., Philipsen, P., Hanson, L., Larsen, J., Gniadecka, M., Wulf, H.: Detection of
skin cancer by classification of Raman spectra. IEEE Transactions on Biomedical
Engineering, Vol. 51 (2004) 1784–1793
3. Tfayli, A., Piot, O., Durlach, A., Manfait, M.: Discriminating nevus and melanoma on
paraffin embedded skin biopsies using FTIR microspectroscopy. BBA General Subjects,
accepted manuscript
4. Mazet, V., Carteret, C., Brie, D., Idier, J., Humbert, B.: Background removal from spectra
by designing and minimising a non-quadratic cost function. Chemometrics and Intelligent
Laboratory Systems, Vol. 76 (2005) 121–133
5. Vrabie, V., Le Bihan, N., Mars, J.: 3D-SVD and partial ICA for 3D array sensors. In: 72
nd
International Conference of the Society of Exploration Geophysicists (SEG’2002). Salk
Lake City, USA.
6. Comon, P.: Independent component analysis: a new concept?. Signal Processing, Vol. 36
(1994) 287–314
7. Cardoso, J.-F., Souloumiac, A.: Blind beamforming for non-Gaussian signals. IEE
Proceedings F, Vol. 140 (1993) 362–370
8. De Lathauwer, L., De Moor, B., Vanderwalle, J.: Fetal electrocardiogram extraction by
blind source subspace separation. IEEE Transactions on Biomedical Engineering, Vol. 47
(2000) 567–572
26