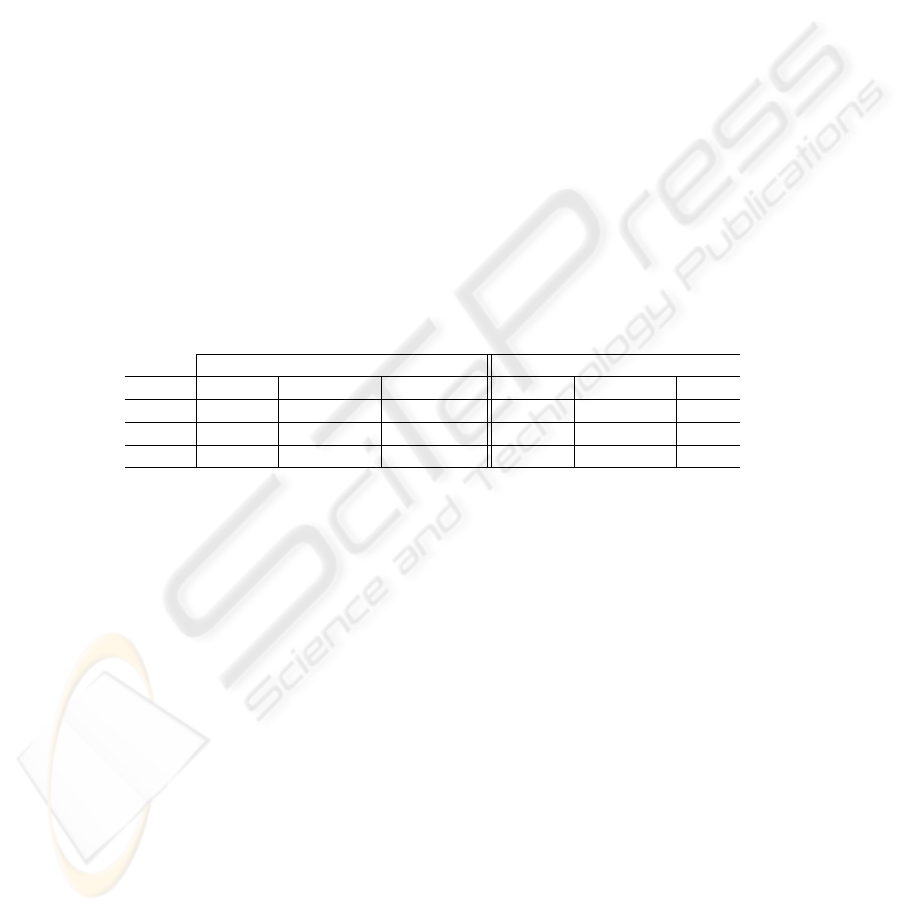
VT) is clearly a non-linear curve. In this case, 4 SVTs have been considered as VTs. The
dashed line represent the direction of maximum variance of the data set when only those
variables are taken into account. It would be easy to implement an algorithm that with
just 2 thresholds: check if the episode is in the upper right or bottom left areas of the
figure, and decide then that it is a SVT case, or, otherwise, decide a VT. For the linear
SVM drawn in Figure 2 b) a simple classification rule can be derived: if (f
6
> 200)
AND (f
10
> 195) decide VT (the values in the figure are normalized).
Table 2 shows the performance of the SVMs, depending on the number of vari-
ables. Classification with more than 4 variables performs worse in term of sensibility
and specificity. As mentioned before, specificity values include their bootstrap standard
error. Sensibility, on the contrary, has been forced to be 100%, at the expense of a lower
specificity; this has been done adjusting conveniently the bias parameter b of the SVM.
For comparison, in [1], a study with data from AICDs showed a 60% of specificty
in VT/SVT detection; the set of tachycardies in this case includes both 1:1 conduction
SVTs and normal SVTs. In [2], 160 SVTs episodes were used, only 16 of them showing
1:1 conduction. Although the algorithm used in [2] shows an 89% specificity, all 16 1:1
SVTs were misclassified. Consequently, the proposed methodology clearly overcomes
existing algorithms for AICDs.
Table 2. Results for both linear and non-linear SVMs, for the most informative features; we also
include the parameters of the best classifier
Non-linear Classifier Linear Classifier
#features Sensibility Specificity Params Sensibility Specificity Params
2 100 89.95 ± 3.90 C : 100, γ : 2 100 80.80 ± 6.97 C : 100
3 100 88.60 ± 5.71 C : 100, γ : 3 100 79.91 ± 6.73 C : 10
4 100 85.57 ± 4.88 C : 100, γ : 4 100 77.28 ± 8.17 C : 10
5 Conclusions and Further Work
In this paper we present a methodology for discriminate 1:1 ventricular tachycardias
from supraventricular ones, based on real signals from dual-chambers AICDs. This
pathology constitute a mayor issue due to existing algorithms do not perform with a
satisfactory level of specificity. Additionally, the occurrence frequency of this tach-
yarrhythmias is low, and the size of our data base is consequently small. The presented
methodology has obtained results that improve the specificity of existing AICDs algo-
rithms.
Our methodology could be used to explore other measurements on the HRV, just
creating new feature vectors without modifying the rest of the algorithms; new mea-
surements coul be based, for example, in mutual information or morphological criteria.
In order to implement SVM classifier into the AICD devices it would be necessary to
study and reduce its computational burden.
100