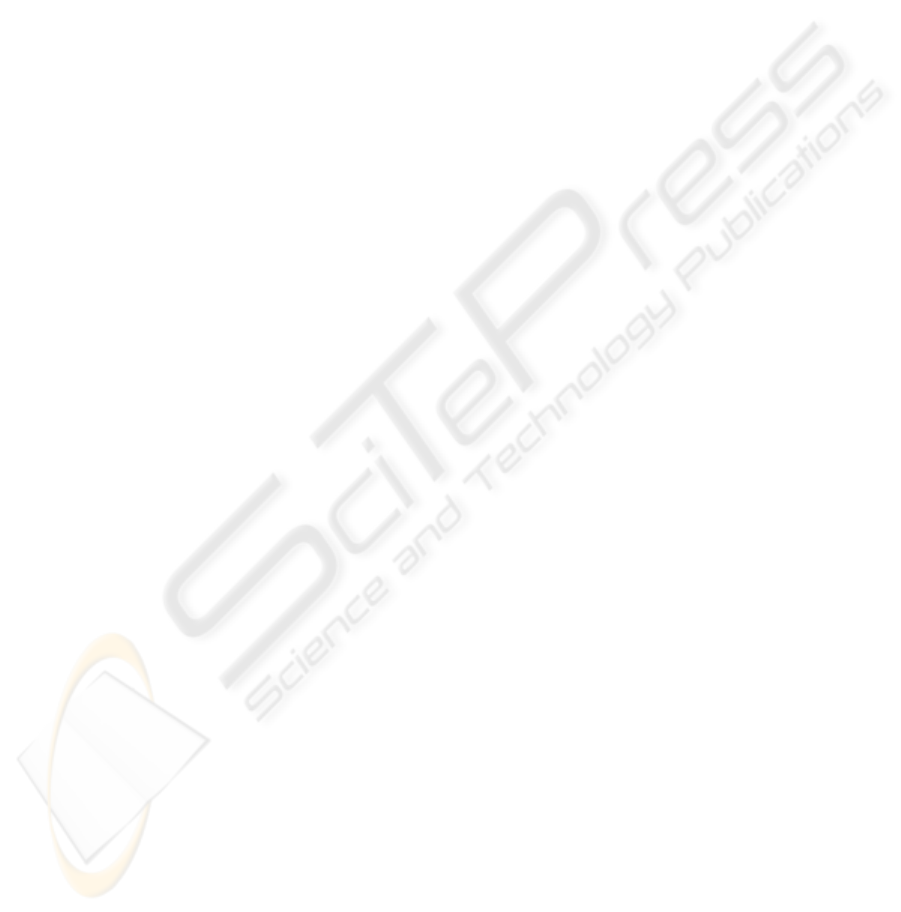
resulting in a higher resolution analysis to detect potential stimuli from exposure. It is
important to point out in such a case where the effects of an external stimulus is of
interest that it is not important to conduct analysis of the human EEG in its frequency
bands. This is due to the fact that the power in the EEG frequency bands is a
physiologically and mentally dependent parameter that presumably does not differ
during control and exposure conditions. Thus for linear analysis the EEG may be
statistically handled in a manner whereby it is fixed.
Overall, it is thought that the proposed spectral analysis of the EEG is a robust and
sensitive method for which to investigate the effects of radiofrequency exposures
from mobile phones on the human EEG. We look forward to incorporating and
further adapting this method into our current study that is underway to examine the
effects of GSM mobile phone exposures on multiple biosignal responses.
Acknowledgements
We would like to extend our thanks to Dr. Everarda Cunningham for her kind support
in reviewing this work.
References
1. Cook, C.M., Thomas, AW., Keenliside, L., Prato, F.S.: Resting EEG Effects During
Exposure to a Pulsed ELF Magnetic Field. Bioelectromagnetics (2005) 26(5):367-76
2. Eulitz, C., Ullsperger, P., Freude, G., Elbert, T.: Mobile phones modulate response patterns
of human brain wave activity. Neuroreport (1998) 9: 3229-3232
3. Huber, R., Graf, T., Cote, K., Wittmann, L., Gallmann, E., Mather, D., Schuderer, J.,
Kuster, N., Borbely, A., Achermann, P.: Exposure to pulsed high-frequency
electrogmagnetic field during waking affects human sleep EEG. Neuroreport (2000) 11:
1321-1325
4. Röschke, J., Mann, K.: No short-term effects of digital mobile radio telephone on the
awake human electroencephalogram. Bioelectromagnetics (1997) 18: 172-176
5. Hietanen, M., Kovala, T., Hämäläinen, A-M.: Human brain activity during exposure to
radiofrequency fieldsemitted by cellular phones. Scandinavian Journal of Work
Environment & Health (2000) 26: 87-92
6. Bachmann, M., Kalda, J., Lass, J., Tuulik, V., Säkki, M., Hinrikus, H.: Non-linear analysis
of the electroencephalogram for detecting effects of low-level electromagnetic fields.
Medical & Biological Engineering & Computing (2005) 43:142-149
7. Lipping, T., Olejarczyk, E., Parts, M.: Analysis of photo-stimulation and microwave
stimulation effects on EEG signal using Higuchi’s fractal dimension method. Proceedings
of SPIE (2004) 5505: 174-178
8. D’Costa, H., Trueman, G., Tang, L., Abdel-rahman, U., Abdel-rahman, W., Ong, K., Cosic,
I.: Human brain wave activity during exposure to radiofrequency field emissions from
mobile phones. Australasian Physical & Engineering Sciences in Medicine (2003) 26[4]:
162-167
9. Reiser, H.-P., Dimpfel, W. Schober, F.: The influence of electromagnetic fields on human
brain activity. European Journal of Medical Research (1995/96) 1: 27-32
10. Sirkin, R. Mark.: Statistics for the Social Sciences. 2nd Edn. Thousand Oaks, Calif.:Sage
Publications (1999)
11. Aston R, Principles of Biomedical Instrumentation and measurement, Meril (1990)
174