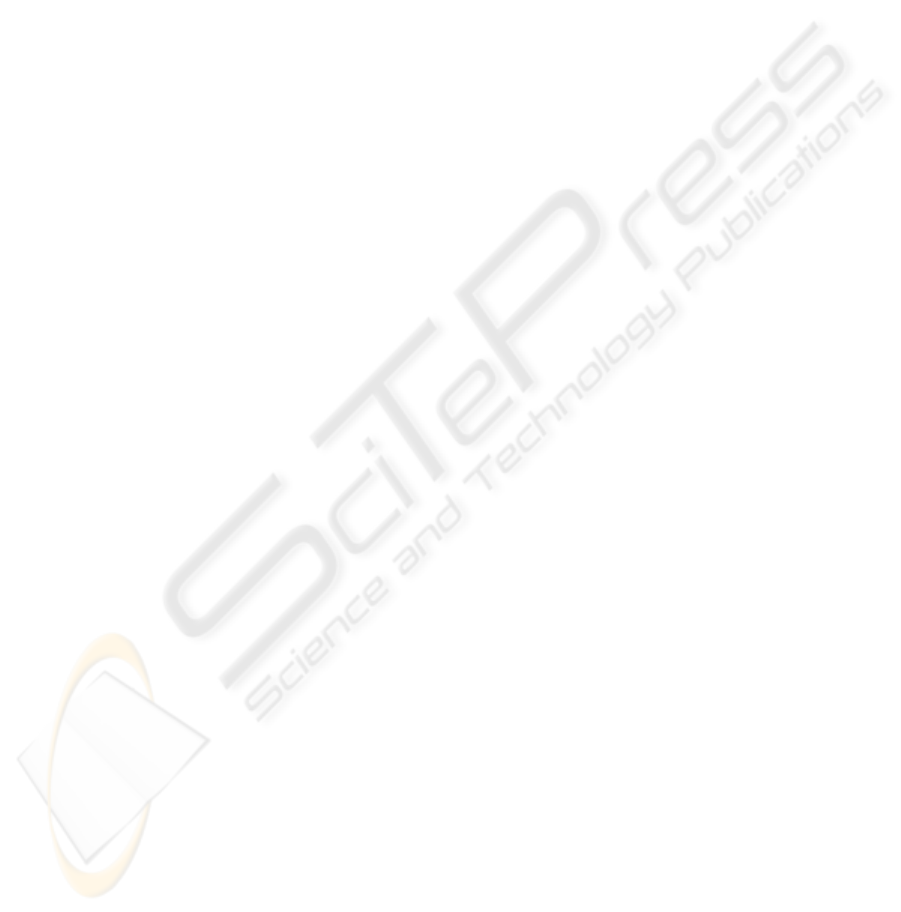
The main input to the proposed methodology is the
discrete choice data, which is collected from
interactive Stated Preference “games” that the e-
shopper agrees to participate. The data is then used
to calibrate a Logit model that will reveal the trade-
offs the e-shopper employed in his or her choice
decision-making process. Afterwards, these results
are employed to search for the available options and
calculate their values as the customer himself would.
Options with high utility value are then shown to the
e-shopper.
The benefits of using the methodology are
twofold. First, it has the potential to increase
customer satisfaction and therefore the likelihood of
sales and revisits. Second, the information on
customer’s choice decision-making process gives the
company insights on how to improve the business
(such as product design and sales). The
implementation of this methodology requires
investigation of the user’s decision-making process
for each product and the development of friendly
interfaces to reduce the time to collect stated
preference data online.
The major challenge of implementing S
POT is the
data gathering. The approach’s input is data from an
interactive SP game that demands customers’ time.
Customers have to be convinced that providing
answers to the interactive game will give them a
better service. A friendly interface can help
overcome this problem by reducing the cognitive
effort needed for the task.
Another alternative would be to insert an
additional reasoning step and try to match a current
e-shopper with a previously recorded decision-
making model. This match could be based on
similarity (i.e. using case-based reasoning) and
would reduce the number of required questions to
elicit the e-shopper’s preferences.
We should also consider that customers may not
be interested in wasting time to take part in a SP
game that evaluates low priced products or services.
This requires an analysis of the customer’s value of
time to discover the threshold from which they
would be willing to compare options further. As a
guideline, the company could employ this
methodology only to the most profitable or high
priced 20% products, which often represents
approximately 80% of the company’s profit.
REFERENCES
Ardissono, L. & Godoy, A. 2000. Tailoring the Interaction
with Users in Web Stores. User Modeling and User-
Adapted Interaction, 10: 251-303.
Ben-Akiva, M. & Lerman, R. 1985. Discrete Choice
Analysis. MIT Press. Cambridge, MA.
Burke, R. 2000. Knowledge-Based Recommender
Systems. In A. Kent (ed.), Encyclopedia of Library
and Information Systems, 69, 32. Marcel Dekker. New
York:
Burke, R. 2002. Hybrid Recommender Systems: Survey
and Experiments. User Modeling and User-Adapted
Interaction, 12(4): 331-370.
Cotter, P. & Smyth, B. 2000. PTV: Intelligent
Personalised TV Guides. Proceedings of Innovative
Applications of Artificial Intelligence, pp. 957-964.
AAAI Press/ The MIT Press.
De Carvalho, M. 1998. A Comparison of Neural Networks
and Econometric Discrete Choice Models in
Transport. Institute for Transport Studies, University
of Leeds, UK. (PhD-thesis)
Domingue, J., Martins, M., Tan, J., Stutt, A., Pertusson, H.
2002. Alice: Assiting Online Shoppers through
Ontologies and Novel Interface Metaphors. (In
Gómez-Pérez, A. & Benjamins, V. R. (eds.)
Knowledge Engineering and Knowledge Management.
Ontologies and the Semantic Web. LNCS, 2473.
Berlin: Springer. p. 335-351.)
Fowkes, T. & Shinghal, N. 2002. The Leeds Adaptive
Stated Preference Methodology. (In Danielis, R. (ed.)
Freight Transport Demand and Stated Preference
Experiments, F. Angeli, Milan.)
Gruber, T. R. 1993. A Translation Approach to Portable
Ontology Specifications. Knowledge Acquisition, 5(2):
199-220.
Kocur, G., Adler, T., Hyman, W. & Aunet, B. 1982. Guide
to Forecasting Travel Demand with Direct Utility
Assessment. Report No. UMTA-NH-11-0001-82,
Urban Mass Transportation Administration, US
Department of Transportation, Washington, DC.
Kozinets, R. V. 1999. E-Tribalized Marketing: the
Strategic Implications of Virtual Communities of
Consumption. European Management Journal, 17 (3):
252-264.
Luce, R. D. 1959. Individual Choice Behavior. New York.
John Wiley & Sons, Inc.
Louviere, J. J. 1988. Analyzing Decision Making: Metric
Conjoint Analysis. Newbury Park, CA: Sage
Publications, Inc.
McGinty, L. & Smyth, B. 2002. Comparison-Based
Recommendation. (In Craw, S. and Preece, A. (eds.)
Advanced in Case-Based Reasoning. LNAI, 2416.
Berlin, Springer. p. 575-589.)
Pearmain, D. & Kroes, E. 1990. Stated Preference
Techniques: a Guide to Practice. Steer Davies &
Gleave, UK.
WEBIST 2005 - WEB INTERFACES AND APPLICATIONS
262