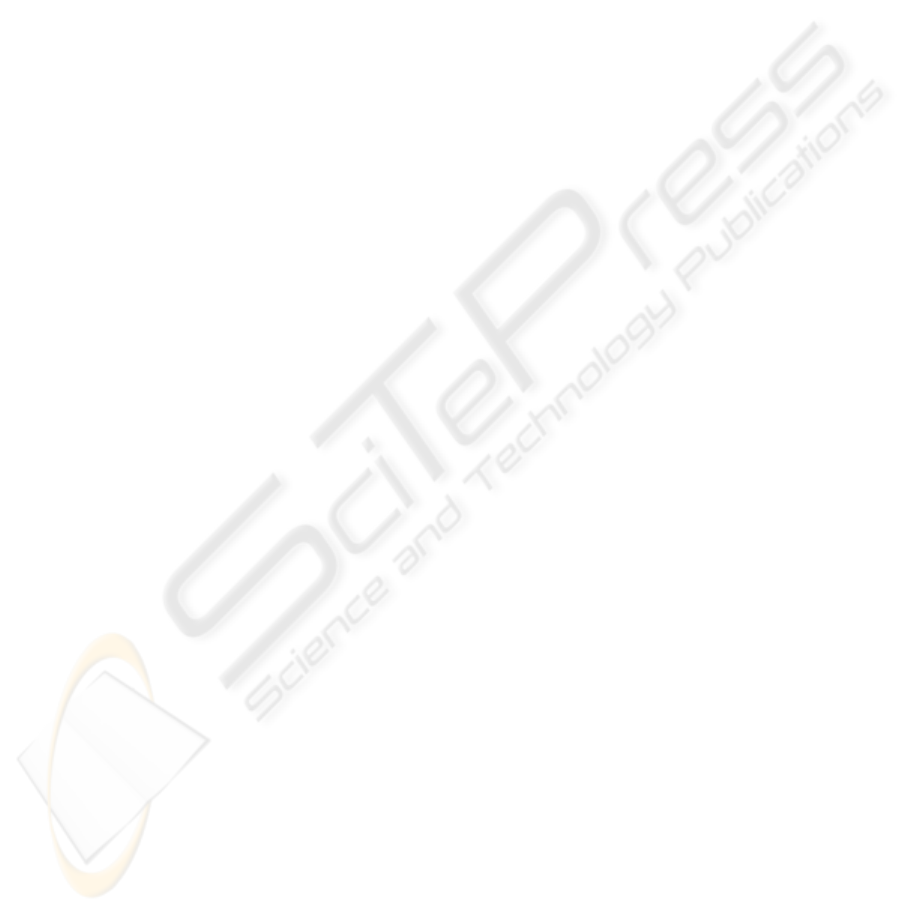
As for each member of the family, the phonetic
content of the voiceprint is different, the identifica-
tion process is performed on both the voice and the
phonetic context, although there is no explicit name
recognition process. Thus it can be expected that the
identification error rate will be negligeable. On the
other hand, as the authentication is performed on a
short utterance (e.g. 4 syllables), the authentication
performances are expected to be rather poor.
2.2 Common sentence-based
scenario
In this scenario, the user pronounces a sentence,
which is common to all the members of the family.
The main characteristics of such an application, com-
pared to the name-based scenario are:
• It is less easy to recall: the sentence should be
prompted to the user, if he does not remember.
• The length of the sentence may be longer than a
simple name.
• It prevents deliberate impostor attempts: e.g. a
mother can not claim to be her daughter.
As for each member of the family the phonetic con-
tent of the voiceprint is the same, the identification
process is performed only on the voice. Thus it can
be expected that the identification error rate will be
higher than in the context of name-based recognition.
On the other hand, as the authentication is performed
on a longer sentence (10 syllables), the authentication
performances are expected to be better than those of
the name-based scenario.
3 DATABASE DESIGN
3.1 Family profile
The families were required to be composed of 2 par-
ents and 2 children. The children are older than 10.
They all live in the area of Lannion, where this work
was conducted. 33 families were recruited: 19 with
one son and one daughter, 10 with 2 sons and 4 with 2
daughters. They were asked to perform 10 calls from
home, with their landline phone, during a period of
one month. Hence, factors such as voice evolution
over time or sensitivity to call conditions are not stud-
ied in this work.
3.2 Name-based scenario
In this scenario, the key point is the fact that there
might be deliberate impostor attempts on a target
speaker, as the user claims an identity to be recog-
nised.
3.2.1 Training
For each member of a family, training is performed
with 3 repetitions of his/her complete name (first +
last name). This number of repetitions represents a
good trade-off between performances and tediousness
of the task.
3.2.2 Within family attempts
Each member of a family is asked to perform attempts
on his own name, and also attempts on the name of
each of the other members of his family.
3.2.3 External impostor attempts
A set of impostor attempts from people who do not
belong to the family is collected. The external im-
postors are composed of members of other families
who pronounced the name of the member of the tar-
get family.
3.2.4 Collected Database
As we focus on speaker recognition, we only retained
the utterances where the complete name was correctly
pronounced.
• 16 families completed their training phase.
• 13 families completed the testing phase also.
• 672 true speaker attempts collected for the 13 fam-
ilies, that makes an average of 52 true speaker
attempts per family, thus an average of 13 true
speaker attempts per user.
• 582 within family impostor attempts collected for
the 13 families, that makes an average of 45 within
family impostor attempts per family, thus an aver-
age of 11 within family impostor attempts per user.
• 2173 external impostor attempts (impostor at-
tempts are performed on all the families who have
completed the training phase)
3.3 Common sentence-based
scenario
In this scenario, the key point is the fact that there
can not be deliberate impostor attempts on a target
speaker, as the user does not claim identity to be
recognised.
3.3.1 Training
For each member of a family, training is performed
with 3 repetitions of the common sentence.
VOICE BIOMETRICS WITHIN THE FAMILY: TRUST, PRIVACY AND PERSONALISATION
331