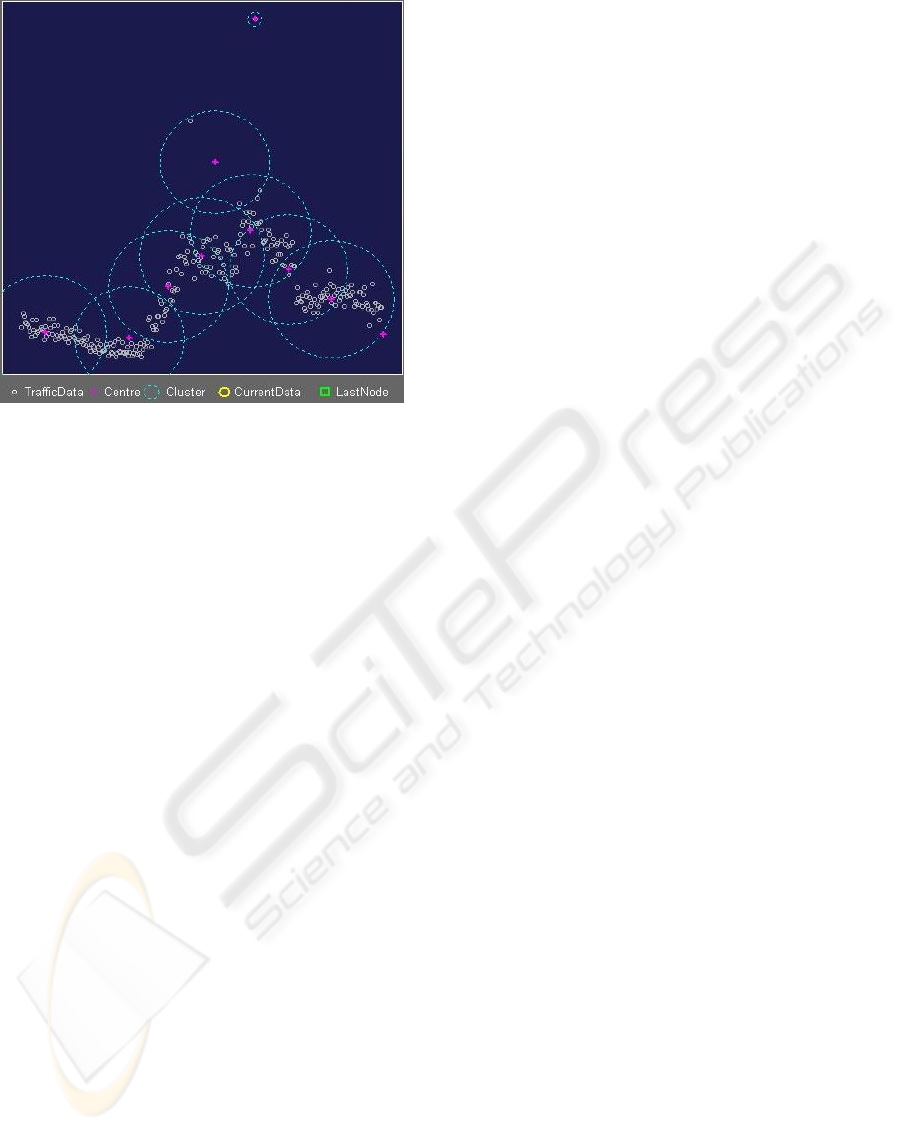
Figure 7: Clusters Produced For Thursday, 03 February
In this testing phase, the system detects some
device failure (especially our switch) happened in
some network segment. A simple DoS attack that we
simulate was also successfully detected by the
engine. As a whole, the system performance was
satisfactory.
6 CONCLUSION
This work is an important step to build a complete
evolvable network monitoring system. By
understanding the network traffic characteristic,
plenty can do to keep on eye on the network, these
includes detecting more anomalous activity such as
current worms, DDoS attack, and other advanced
network intrusion.
Working in offline mode, the integrity of the
data in the capturing process can be argued. While
capturing the data for duration of (let say) two
months, it cannot completely represent the normal
traffic happened in the network. A case might
happened for some heavy traffic captured in those
two months period was because the corporate where
the network resides was in an intensive work load to
launch a product. This thing will affect the integrity
of the resulted profile and might lead to be more
burdens for network administrator to recapture the
traffic, reevaluate the traffic, and finally re-profile
the traffic.
Our system still needs improvements in many
ways for future works. Currently we are improving
the structure of the connectionist model by
proposing new methods. By having these methods
which specifically designed for network traffic data,
the results can be more accurate and the system can
grow from scratch. We also try to consider
implementing a signature based intrusion detection
engine to improve the detection engine’s
performance. Lastly, our future work will also to add
in an intelligent module to automate an action as
responses for such an alarmed event to prevent
network down, which in turn will reduce the
dependency of the network from network
administrator’s presence when an event which
requires an immediate attention or response
happened at late night.
REFERENCES
Pasha, M. F. and Budiarto, R., 2004. Developing Online
Adaptive Engine for Profiling Network Traffic using
Evolving Connectionist Systems. In NCEI’04,
Conference on Neuro-Computing and Evolving
Intelligence 2004. Auckland, New Zealand.
Kasabov, N., 2003. Evolving Connectionist System:
Methods and Applications in Bioinformatics, Brain
Study and Intelligent Machines, Springer-Verlag.
London, 1
st
edition.
Pasha, M. F., Budiarto, R., Sumari, P., and Osman, A.,
2004. Data Mining and Rule Generation in Network
Traffic using Fuzzy Clustering Techniques. In
M2USIC’04, MMU International Symposium on
Information and Communications Technologies.
Putrajaya, Malaysia.
Lampinen, T., Koivisto, H., and Honkanen, T., 2002.
Profiling Network Application with Fuzzy C-Means
Clustering and Self Organizing Map. In First
International Conference on Fuzzy System and
Knowledge Discovery: Computational Intelligence for
the E-Age. Singapore.
Song, Q. and Kasabov, N., 2001. ECM, A Novel On-line,
Evolving Clustering Method and its Applications. In
ANNES’01, Fifth Biannual Conference on Artificial
Neural Networks and Expert Systems.
Marchette, D., 1999. A Statistical Method for Profiling
Network Traffic. In Workshop on Intrusion Detection
and Network Monitoring. USA.
Paxson, V., 1998. Bro: A System for Detecting Network
Intruders in Real-Time. In 7
th
USENIX Security
Symposium. USA.
Kasabov, N. and Song, Q., 2002. DENFIS: Dynamic
Evolving Neuro-Fuzzy Inference System and its
Application for time-series prediction. In IEEE Trans.
Fuzzy System, 10(2), 144-154.
Purvis, D., Kasabov, N., Benwell, G., Zhou, Q., and
Zhang, F., 1999. Neuro-Fuzzy methods for
environmental modelling. In System Research and
Information Systems, 8(4), 221-239.
ICETE 2005 - SECURITY AND RELIABILITY IN INFORMATION SYSTEMS AND NETWORKS
208