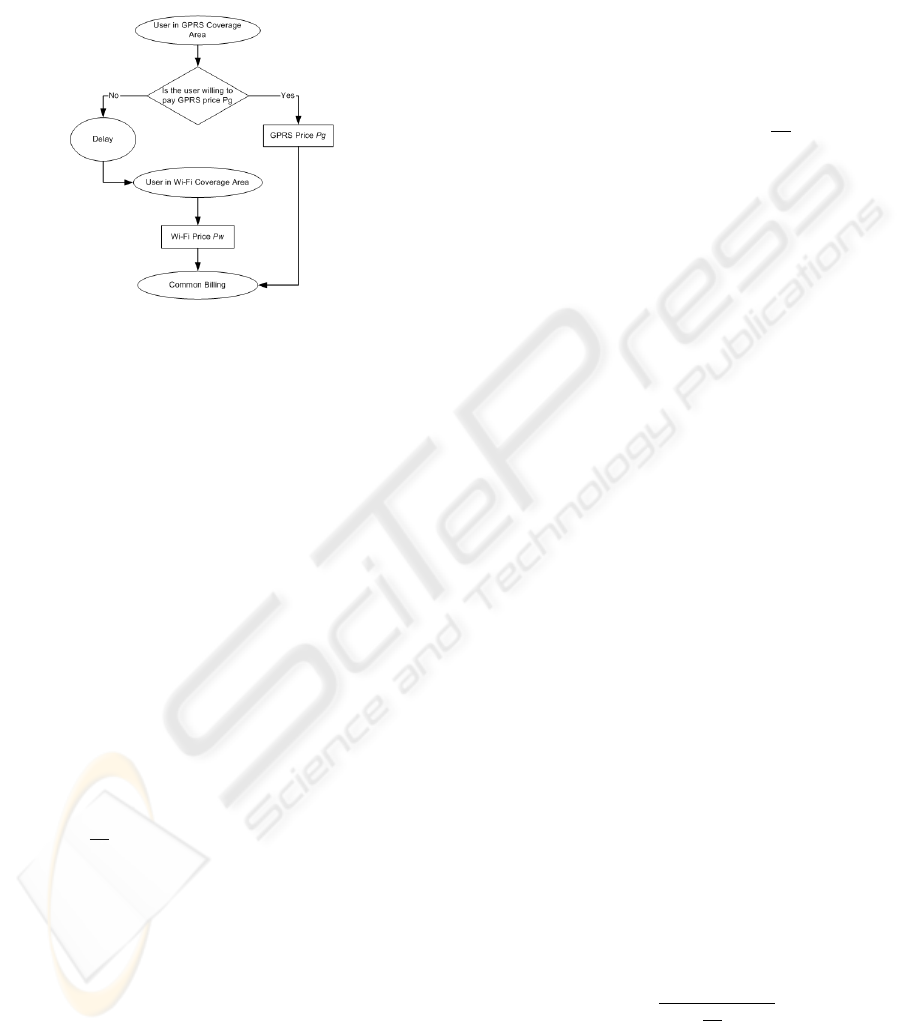
that fee is not significant enough to contribute to the
Wi-Fi charges when compared to charges from GPRS
services. Fig. 3 illustrates a blocking diagram for the
scenario we just described.
Figure 3: Pricing Block for GPRS networks with Wi-Fi in-
tegration.
As mentioned earlier, the cost of transmitting data
over a GPRS network is quite high when compared to
Wi-Fi network. This significant price difference be-
tween the two networks can influence the way users
use these networks. Some users may be willing to
search for a Wi-Fi network, if they need to perform
a large file transfer. Hence, price incentive can influ-
ence users to use either GPRS services with higher
price tag (more convenience since the network has
larger coverage) or Wi-Fi services with lower price.
We argue that the percentage of mobile users D who
accept to be charged by GPRS networks, depends
heavily on the price ratio between the two networks
(Hou, 2001). D can be mostly influenced by the de-
mand function which is a function that characterizes
the reaction of users to changes in price. In this paper
we use the demand function that appears in (Oldyzko,
2000) since it is used for different classes of users,
that fit our model. The demand function is as follows:
D = e
−(
p
g
p
w
−1)
2
, 0 ≤ D ≤ 1, p
h
≥ p
o
(1)
where p
g
is the GPRS charge and p
w
is the Wi-
Fi charge for each user (p
w
could be either operator
owned or third party owned hot spot charge). We are
interested in the case when price incentive can influ-
ence decision of users. Therefore, the session volume
charged by GPRS networks must be large enough to
allow p
g
to be greater than p
w
. As we know GPRS
pricing is usage-based charges, which do not depend
on holding time but the session volume, therefore, p
g
is a linear function of the number of megabytes trans-
mitted over the network. For Wi-fi charge, it is based
on flat pricing, which is basically a connection fee.
Therefore, p
g
and p
w
can be described as follows:
P
g
= r
g
· v
P
w
= r
w
(2)
where r
g
is the charging rate per megabyte for the
GPRS network, r
w
is the connection fee of the Wi-Fi
network, and v is the session volume of data that users
transmit over the GPRS network.
Lower curve in Fig. 4 illustrates the demand func-
tion in (1). The horizontal axis represents the price ra-
tio between GPRS and Wi-Fi charges (
p
g
p
w
− 1). This
function works quite well in our model because, first,
the demand function begins high for small price ratio
representing the situation when users have small vol-
ume of data to transmit. The price charged by GPRS
networks would not be much different from the Wi-
Fi charge. The users have little incentive to seek for
Wi-Fi hotspots resulting in the high user demand for
GPRS usage. Then, the demand decreases rapidly as
the curve gets into a mid-range and has very narrow
tail. This part of the curve represents the increase in
GPRS charges due to the increasing session volume
from users. There is enough incentive for some users
to start migrating to Wi-Fi resulting in the reduction of
GPRS usage. For example, when the price difference
at the horizontal axis equals to one, the GPRS charge
is double the Wi-Fi charge, resulting in the GPRS de-
mand dropping to 36.79 percents.
The revenue due to integration can be determined
by the weighted sum of the revenue created by the
GPRS networks and Wi-Fi networks based on their
corresponding demand. Therefore, from equation (2),
the average revenue of the integrated network can be
determined as follows:
R
int
= D · (r
g
· v) + (1 − D) · r
w
(3)
Regarding the revenue gained from GPRS network
without Wi-Fi integration, the GPRS users do not
have an alternative to migrate their traffic. Therefore,
the demand of users using non-integrated GPRS net-
works would be higher than equation (1). Since the
distribution of session volume in GPRS networks is
not available to us, we have to come up with a mean-
ingful demand function. This function needs to be
well above the demand function in equation (1), to
represent the higher demand of GPRS networks in the
absence of Wi-Fi. The demand function can be shown
as follows (Oldyzko, 2000):
D
w.o.int
=
1
1 + (
P
g
K
− 1)
4
(4)
where K is a constant. In our case, we set K equal
to p
w
for the purpose of fitting our demand curve ac-
cording to our assumptions. That, does not mean that
D
w.o.int
depends on the Wi-Fi charge. By compar-
ing the revenue generated in both cases, we can gain
A PRICING MODEL OF GPRS NETWORKS WITH WI-FI INTEGRATION FOR “HEAVY” DATA USERS
81