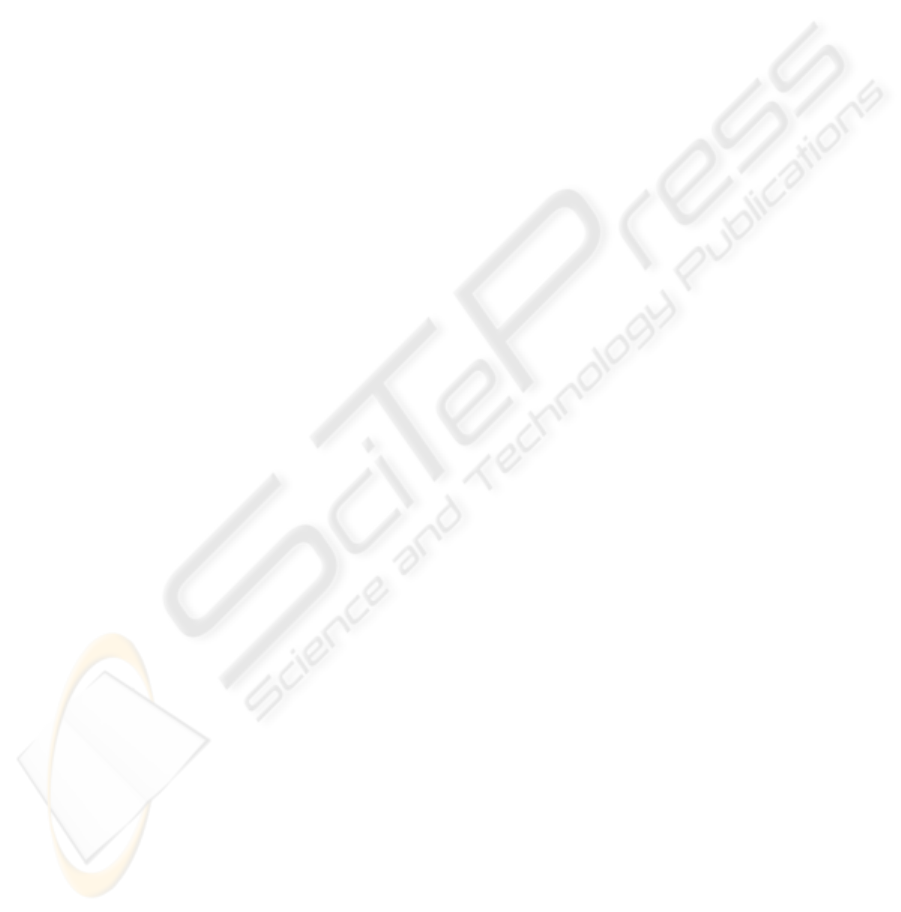
domain are considered in a separate phase (the phase of ranking). Such decomposition
favors the reuse of our inference approach in other personalization applications.
For example, our proposal could be applied in recommender systems for the Se-
mantic Web, where few proposals have been defined up to now. So, a possible example
for motivating the usefulness of our approach in the Semantic Web, could be a system
which suggests to a user travel destinations, by considering relations between coun-
tries according to their customs, culture, etc. For that purpose, it is only necessary to
conceptualize this new domain by an OWL ontology, and to know the user preferences.
At this moment, we have a first prototype of AVATAR by which appealing recom-
mendations have been obtained. Our goal is to extend these encouraging results to a real
scenario with a greater number of users. For that reason, we are working on statistical
studies by considering different population groups with diversified characteristics, such
as genre, age, cultural level, etc. This way, we evaluate the usefulness of the semantic
inference as novel recommendation strategy both in the TV domain and out of it.
References
1. Ardissono L., Kobsa A. and Maybury M. In: Personalized Digital TV: Targeting Programs
to Individual Viewers. Kluwer Academic Publishers (2004)
2. Davies J., Fensel D. and van Harmelen F. In: Towards the Semantic Web: Ontology-driven
Knowledge Management. John Wiley & Sons (2003)
3. Burke R.: Hybrid Recommender Systems: Survey and Experiments. User Modeling and
User-Adapted Interaction 12 (2002) 331–370
4. Lang K.: Newsweeder: Learning to filter netnews. In: Proc. of 12th International Conference
on Machine Learning. (1995)
5. Armstrong R., Freitag D., Joachims T. and Mitchell T.: WebWatcher: A Learning Apprentice
for the World Wide Web. In: AAAI Spring Symposium on Information Gathering. (1995)
6–12
6. Terveen L., Hill W., Amento B., McDonald D. and Creter J.: PHOAKS: A system for sharing
recommendations. Communications of the ACM 40 (1997) 59–62
7. Konstan J., Miller B., Maltz D., Herlocker J., Gordon L. and Riedl J.: GroupLens: Applying
collaborative filtering to Usenet news. Communications of the ACM 40 (1997) 77–87
8. Balabanovic M. and Shoham Y.: FAB: Content-Based Collaborative Recommender. Com-
munications of the ACM 40 (1997) 66–72
9. Smyth B. and Cotter P.: A personalized television listings service. Communications of the
ACM 43 (2000) 107–111
10. Difino A., Negro B. and Chiarotto A.: A multi-agent system for a personalized electronic
program guide. In: Proc. of the Workshop on Personalization in Future TV (TV-02). (2002)
11. Kurapati K., Gutta S., Schaffer D., Martino J. and Zimmerman J.: A multi-agent tv recom-
mender. In: Proc. of the Workshop on Personalization in Future TV (TV-01). (2001)
12. Anyanwu K. and Sheth A.: ρ-Queries: Enabling Querying for Semantic Associations on the
Semantic Web. In: Proc. of the 12th International World Wide Web Conference (WWW-03),
ACM Press, 1-58113-680-3/03/0005 (2003) 690–699
13. Blanco Y., Pazos J., Gil A., Ramos M., López M. and Barragáns B.: AVATAR: Modeling
Users by Dynamic Ontologies in a TV Recommender System based on Semantic Reasoning.
In: Proc. of the 3rd European Conference on Interactive Television (EuroITV-05). (2005)
14. Blanco Y., Pazos J., Gil A., Ramos M., García J., Díaz R., Fernández A., Barragáns B. and
López M.: AVATAR: Advanced Telematic Search of Audiovisual Contents by Semantic
Reasoning. In: Proc. of the 4th Workshop on Personalization in Future TV (TV-04). (2004)
78