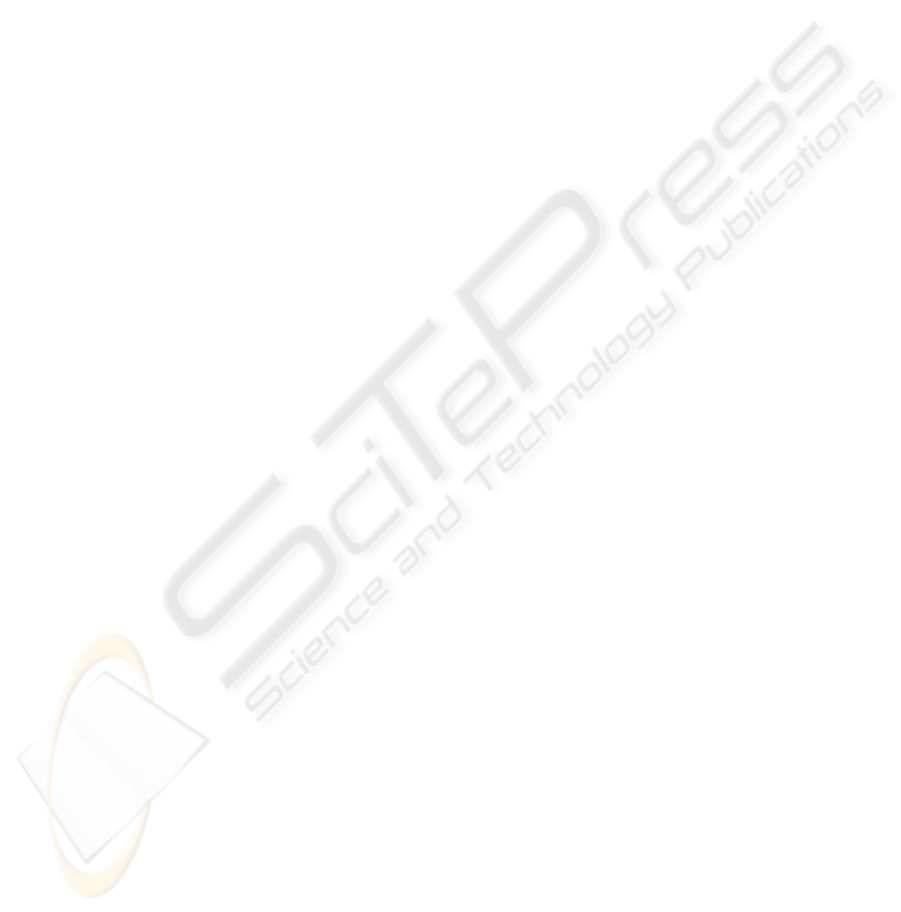
5 Conclusions
The accuracy of automated classification is currently insufficient for a fully
automated system with no input from the tutor as to which announcements are sent to
students. This is not surprising given the short length of most announcements. In the
experiments showing how performance improved with increasing amounts of training
data the performance appears to still be steadily improving, although the accuracy for
the single data point for 80 announcements does confuse this issue. Also, it is not
unusual for automated systems to perform worse than humans and yet prove to be of
use. This can be due to cost implications, or if there is a problem getting tutors to
manually label announcements. Language translation systems are an example of a
technology where although human translators produce results of much higher quality,
they still have many uses. Either as a “first pass” later improved by human translators,
or in situations where a human translator would be too expensive and slow, such as
when browsing foreign language documents on the internet.
However, it is important to note that the human classification was also quite low.
Since the two authors who created the training data had taught together on a number
of modules over some years, higher accuracy near to 100% might have been expected.
The fact that only 80% accuracy was achieved suggests that the content of the
announcements is insufficient for very high accuracies, no matter how much
intelligence and background knowledge is bought to the task.
It was noted that many of the most indicative words had meaning in the context of the
module, rather then being generally applicable across many modules. This is a serious
problem, as if the system needs to be trained on a module by module basis, even the
80 announcements used here then many announcements will be required before the
system starts working. This is a strong indication that background-knowledge free
text classification will not be applicable in this domain.
Hence both human classification, applying full human intelligence and background
knowledge, and a good machine classification technique indicate that announcement
classification is unlikely to be useful. Our conclusions are that there does not appear
to be enough information in announcements themselves to classify correctly, and
larger amounts of context knowledge will be necessary.
Despite these negative results, several enhancements to the classifier are planned,
most of which are fed by parallel research into author attribution. In particular,
information fusion [4] approaches have shown promise in improving the confidence
we can have in automated authorship attributions, if not the total accuracy. Like much
technology, we would expect steady improvements in the performance of the
automated system, while human requirements and performance are likely to remain
static. We are encouraged by the comments of Christensen et al (2001) who argue that
technology advances faster than user requirements. However, our results from human
classification do call into question whether any amount of technology will really be
able to solve this problem.
Whether sending a SMS text that should not be sent, or failing to send an SMS text
that should not be sent is a greater error is a question for management, not technology.
The ability to tweak the classifier to achieve different balances between false accept
and false reject means that different management policies can be implemented in the
system. This is also important given that tutors may (or may not) choose to override
123