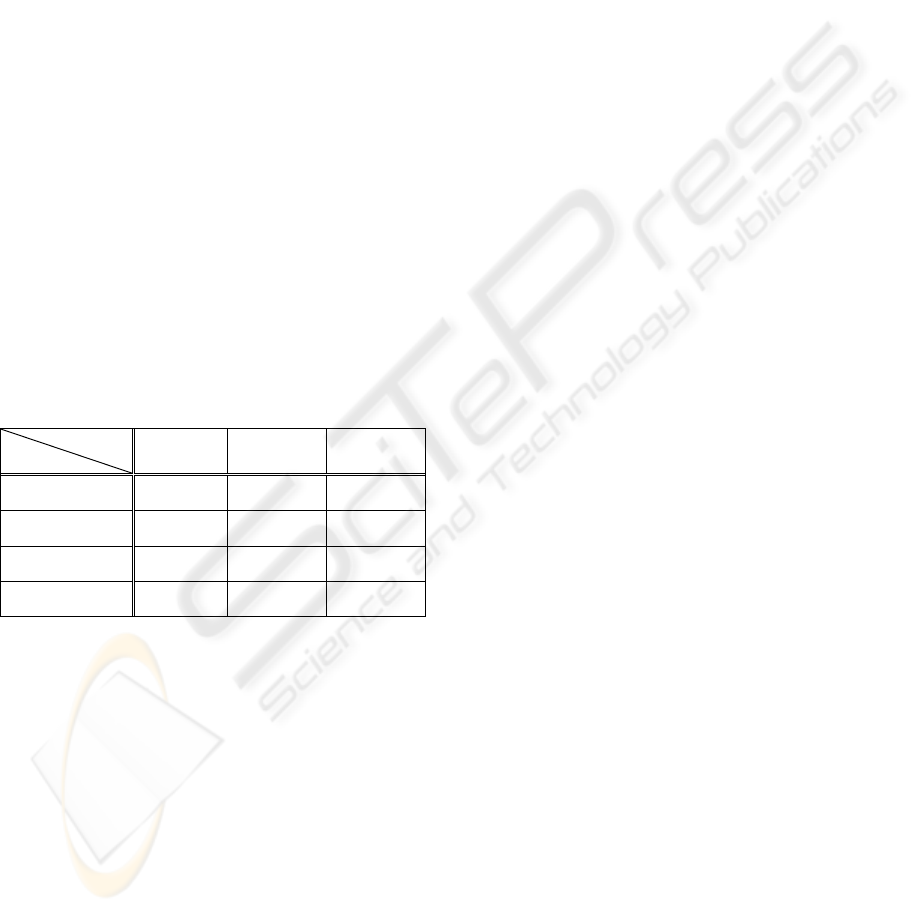
influenced by the register sequence, that indicated
that the recognition performance of the system was
not affected by increase of templates. While for
counterfeit rejection capability, there were two cases:
one case was that new register led to retraining on
the templates registered previously (Table 5 and
Table 6); the other case was that no retraining was
resulted by new register (Table 7 and Table 8).
According to Table 5 and Table 6, in both two
systems with different activation function, the
rejection capability of template A was enhanced by
new register of B and this improvement was
especially remarkable with employment of Gaussian
function. From Table 7 and Table 8, the rejection
performance of template B kept untouched because
no retraining was caused by recruitment of template
A. these experiment results showed that recruitment
of new templates was helpful to decreasing the
possibility of mis-recognition on counterfeit
signatures in one template.
The results in Table 3 and 4 involved the
influence among templates. Excluding the influence
among templates, the rejection performance of
templates with different activation function was
investigated and corresponding results were listed in
Table 9. Note that in each case, only one registrant
was involved in the system.
Table 9: Rejection performance of template with
different activation function (ideal value: 100%)
Function
Registrant
Sigmoid Gaussian Difference
A only 57.78% 68.89% +11.11%
B only 85.56% 90.0% +4.44%
C only 73.77% 87.04 % +13.27%
Average 72.37% 81.97% +9.60%
It can be seen that even without the help of
favourable influence of templates, Gaussian function
was still effective on improving the rejection
capability of template.
During experiments, the width parameter σ was
found to have great influence on both recognition
capability and counterfeit rejection capability of the
system with Gaussian function. We are engaging in
developing automatic optimisation method of σ for
each registrant’s neuro-template.
6 CONCLUSION
In this paper, both of the construction and algorithm
of the individual recognition system with writing
pressure were firstly described. Then, Gaussian
function, which is one of RBF, was proposed as
activation function of neuro-template to improve the
rejection capability of the system for counterfeit
signatures. Furthermore the influence among neuro-
templates was investigated in this paper. The
experiments results suggested that the influence
among templates was favourable for rejection
capability of the system, and more importantly the
experiment results shown that Gaussian function
combined with neuro-template was seemed to be
very effective in improving rejection performance of
the system for counterfeit signatures on premise of
ensuring the recognition performance satisfied.
REFERENCES
Takeda, F. and Omatu, S., 1995. High speed paper
currency recognition by neural networks. IEEE
Transaction on Neural Networks, Vol.6, No.1,pp.73-77.
Takeda, F. and Omatu, S., 1996. A neuro-system
technology for bank note recognition, Proceedings of
the Japan/USA Symposium on Flexible Automation,
Boston,USA, Vol.2, pp.1511-1516.
Takeda, F. and Omatu S., 1998, Neural network systems
Technique and applications in paper currency
recognition neural networks systems, Technique and
Applications, Vo1.5, Chapter 4, pp.133-160,
ACADEMIC Press.
Takeda, F. Omatu, S., and Nishikege, 1996, A neuro-
recognition technology for paper currency using
optimized masks by GA and its hardware, Proceedings
of the International Conference on Information System
Analysis and Synthesis, Orlando, USA, pp147-152.
Takeda, F., Nishikage, T. and Matsumoto, 1998, Y.,
Characteristic extraction of paper currency using
symmetrical masks optimized by GA and neuro-
recognition of multi-national paper currency,
Proceedings of IEEE World Congress on
Computational Intelligence, Alaska, USA, Vol.1,
pp.634-639.
Fnakubo, M., 1991, Pattern Recognition, Japan: Kyouritsu
Press,pp15-25.
Abhijit S. Pandya, and Robert B. Macy, 1996, Pattern
Recognition with Neural Networks in C++, Chapter 5,
pp147-151, IEEE Press.
Kikuti, T., 1989, Introduction to neuro-computer, pp.35-
67, Japan: Omu Press.
Nagao, M., 1993, Pattern Recognition, Japan: CORONA
publishing CO. LTD., pp.92-161.
ICEIS 2005 - ARTIFICIAL INTELLIGENCE AND DECISION SUPPORT SYSTEMS
162