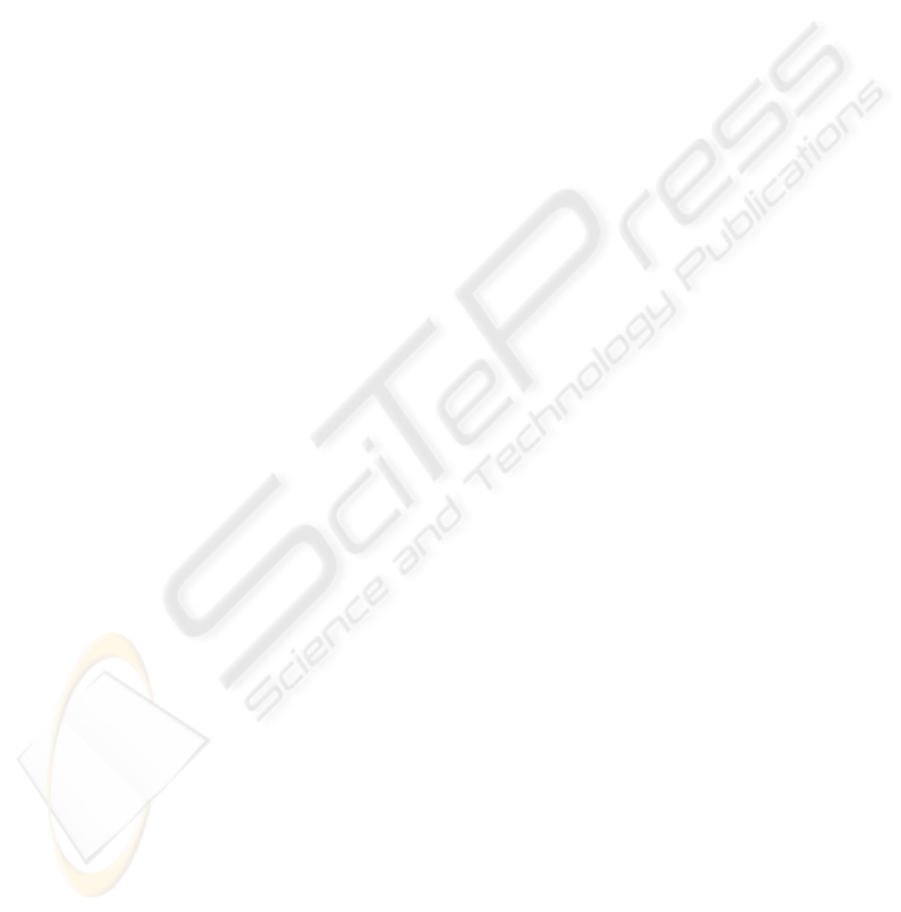
be paid for the great reduction of a response time,
which has been reported in the previous sub-section.
This much shorted duration of filtering can be also
the only possible explanation that the received feed-
back value has been reduced for only 3%, which is
two times less that is the reduction of a precision.
6 CONCLUSION
The goal of this paper was to provide solutions to
the challenges in filtering community cooperation,
being unavoidable in nowadays rich information
society. This was achieved through methods being
able both to determine how promising is each avail-
able community for a particular request and to com-
pose the final recommendation set by choosing the
best from the found results.
Even though the first solutions for describing in-
formation being stored at each community are given,
a future work will be focused on the usage of shared
ontologies for taking care about very diverse seman-
tics that the same word has in different communities.
The price, being paid in a user feedback, precision
and recall domains, will be tried to be reduced also
through the application of specialised strategies for
keeping updated the content descriptions of distrib-
uted, heterogeneous and dynamic filtering communi-
ties. As soon as it becomes unfeasible that each and
every community has descriptions of all others, the
Time-To-Live parameter will be assigned to every
filtering job, which will enable their further propaga-
tion, being the basic idea behind all P2P data sharing
systems. Even though given results are just the ini-
tial step towards intelligent cooperation in a multi
agent framework, authors’ hope is that the deployed
cooperation lays the foundation for the provision of
sophisticated filtering services.
REFERENCES
Albayrak, S., Milosevic, D., 2004a. Generic Intelligent
Personal Information Agent. IPSI-2004S. Serbia.
Albayrak, S., Milosevic, D., 2004b. Self Improving Coor-
dination in Multi Agent Filtering Framework. IEEE,
WIC and ACM Conference on IAT and WI. China.
Albayrak, S., Milosevic D., 2004c. Situation-aware Coor-
dination in Multi Agent Filtering Framework. The
19th Symposium on Computer and Information Sci-
ences (ISCIS 04). Antalya. Turkey. pp 480-493.
Balabanovic, M., Shoham Y., 1997. Fab: Content-Based
Collaborative Recommendation. Communication of
the ACM. 40(3). pp. 66-72.
Blake, C., Pratt W., 2001. Better Rules, Fewer Features: A
Semantic Approach to Selecting Features from Text.
Proceedings of the IEEE International Conference on
Data Mining. San Jose. California. pp. 59-66.
Boley, D., 1998. Principal Direction Divisive Partitioning.
Data Mining and Knowledge Discovery. 2(4).
Brin, S., Page L., 1998. The anatomy of a large-scale hy-
per textual (Web) search engine. 7
th
WWW Confer-
ence on Computer Networks. 30(1-7). pp. 107-117.
Delgado, J., 2000. Agent based information filtering and
recommender systems. Ph.D. Thesis, Nagoya Institute.
Faloutsos, C., Oard, D., 1995. A Survey of Information
Retrieval and Filtering Methods. Technical Report
CS-TR-3514. University of Maryland.
Fricke, S., Bsufka, K., Keiser, J., Schmidt, T., Sesseler, R.,
Albayrak, S., 2001. Agent-based Telematic Services
and Telecom Applications. Comm. of ACM. pp. 43-48.
Frigui, H., Nasraoui, O., 2004. Simultaneous clustering
and dynamic keyword weighting for text documents.
Survey of Text Mining Clustering, Classification, and
Retrieval. Springer-Verlag. pp. 45-72.
Han, J., Kamber, M., 2001. Data Mining: Concepts and
Techniques. Morgan Kaufmann Publishers.
Kohonen, T., Kaski, S., Lagus, K., Salojärvi, J., Honkela,
J., Paatero, V., Saarela, A., 2000. Self organization of
massive document collection. IEEE Transactions on
Neural Networks. 11(3). pp. 574-585.
Michalewicz, Z., Fogel, D., 2000. How to Solve It: Mod-
ern Heuristics, Springer-Verlag New York.
Mohammadian, M., Jentzsch, R., 2004. Computational
Intelligence Techniques Driven Intelligent Agents for
Web Data Mining and Information Retrieval. Intelli-
gent Agents for Data Mining and Information Re-
trieval. Idea Group Publishing. pp. 15-29.
Oard, D., Marchionini, G., 1996. A Conceptual Frame-
work for Text Filtering. MD 20742. Maryland.
Saurabh, S., Eui-Hong, H., Vipin, K., 2001. Web Search
Engine Technologies: A Survey. Minnesota. USA.
Sesseler, R., Albayrak, S., 2002. JIAC IV – An Open,
Scalable Agent Architecture for Telecommunications
Applications, 1
st
International NAISO Congress on
Autonomous Intelligent Systems, Geelong. Australia.
Sheth, B. D,, 1994. A Learning Approach to Personalized
Information Filtering. M.S. Thesis. MIT- EECS. USA.
Tauritz, D., 2002. Adaptive Information Filtering: con-
cepts and algorithms. Ph.D. Thesis. Leiden University.
Yang, H., Zhang, M., 2004. Potential Cases, Database
Types, and Selection Methodologies for Searching
Distributed Text Databases. Intelligent Agents for
Data Mining and Information Retrieval. IG. pp. 1-14.
Zamir, O., Etzioni, O., Madani, O., Karp, R., 1997. Fast
and Intuitive Clustering of Web Documents. Knowl-
edge Discovery and Data Mining (KDD 1997). pp.
287-290.
DISTRIBUTED COMMUNITY COOPERATION IN MULTI AGENT FILTERING FRAMEWORK
407