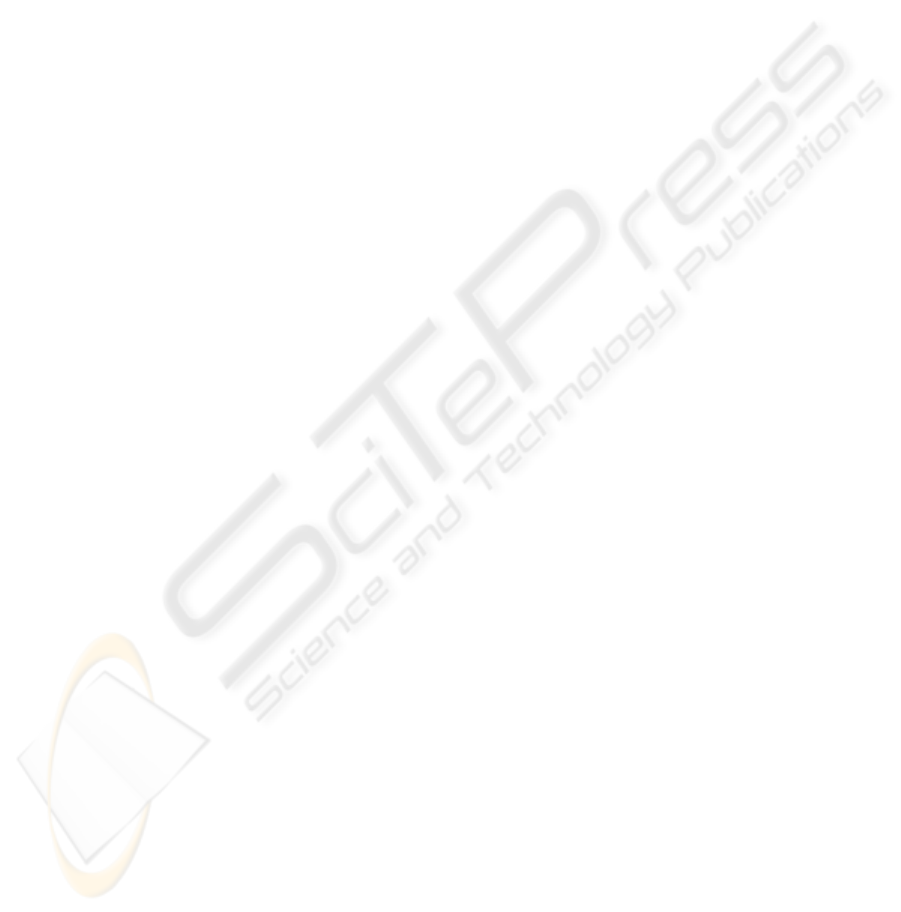
survey how consumers make trade-offs between
attributes collects.
Methods that use POS data, however, fail to
collect information on the preferences of the
shoppers who do not make purchases, while
methods that use elaborate questionnaires are
expensive and time-consuming even if questionnaire
survey can be conducted by online environment such
as Internet (Miller and Dickson, 2001). When we are
dealing with short-life-cycle products like PCs, we
need an economical and fast means of tracking shifts
in consumer needs in response to new product
launches, price changes, and competitors’ moves.
The widespread use of Internet has allowed
companies to offer product information and prices in
real time, and take purchase orders through online
shopping systems (Kalakota and Whinston, 1997).
Ono and Matsuo (2000) focused on the browsing
data of consumers who did not make purchases. The
proposed method used data on consumer browsing
history involving available product configurations in
an online shopping environment. The information
could easily and inexpensively be collected by the
seller, reflecting individual consumer preference as
well as her/his chosen set.
The proposed method consisted of three steps:
(1) collecting data on individual consumers’
browsing histories for quotations and purchase
requests, (2) converting requests for quotations and
purchase order data into ordinal preference data, and
(3) estimating consumer preference on product
attributes by applying a multiattribute utility
function (Green and Krieger, 1993).
The proposed method assumed that that a
product configuration quoted later would be
preferred to those quoted earlier. It also assumed that
how many times a product configuration was quoted
would not affect estimates of product preference as
long as it was quoted at least once. However, some
consumers might prefer a configuration that was
quoted earlier or one that was selected more
frequently. In this paper, we evaluate the validity of
several conceivable hypotheses regarding the
relationships between product preferences and the
sequences and frequency of quoted product
configurations. We are not concerned with the wider
issue affecting preferences such as choice of store,
quality of on-line store and prior experience
(Marsden et al., 1999).
The rest of this paper is organized as follows.
Section 2 describes the procedure for estimating
product preference. Section 3 describes proposed
methods of converting requests for quotation data
into ordinal preference data. Section 4 reports our
experimental evaluation of the proposed methods
through experiments. Section 5 concludes the paper.
2 ESTIMATING PRODUCT
PREFERENCE
2.1 Definition of Product Preference
The product attributes of the PC we consider in this
paper are its total price and the performance levels
of its components such as the CPU, random access
memory and hard disk drive. Product attributes have
various levels. The random access memory level, for
example, is measured by its storage capacity (e.g.,
256 and 512 MB).
When consumers purchase a product, they decide
whether some attributes are more important than
others, and what levels for all attributes are required
or preferred. A particular configuration that a
consumer purchases is regarded as the configuration
that has the largest total preference calculated from
the preference for each product attribute. The
product preference of each consumer can be
expressed as an additive model of the multiattribute
utility function (Green and Krieger, 1993):
)1( )(),,,(
1
21
∑
=
=
K
k
kkK
xuxxxU L
where x
k
is the level of attribute k, U(x
1
, x
2
,…,x
k
) is
the product preference for attribute levels equal to x
1,
x
2, …,
and x
k
, and u
k
(x
k
) is the preference for attribute
k at level x
k
.
As preference differs from one consumer to
another, the attribute preferences are estimated for
each consumer. When the preferences of all
attributes are estimated, we can estimate the
preference for a product that is represented by the
combination of attributes.
2.2 Procedure for Estimating Product
Preference
Ono and Matsuo’s method proposed (2000) consists
of three steps. This subsection describes each step in
detail.
(1) Collecting requests for quotations and
purchase order data
The consumer’s series of requests for quotations
and orders are collected using an online shopping
system. Figure 1 shows a web screen for such a
system. The consumer’s operational procedure using
online shopping system is as follows.
EVALUATION OF METHODS FOR CONVERTING REQUEST FOR QUOTATION DATA INTO ORDINAL
PREFERENCE DATA: Estimating Product Preference in Online Shopping System
25