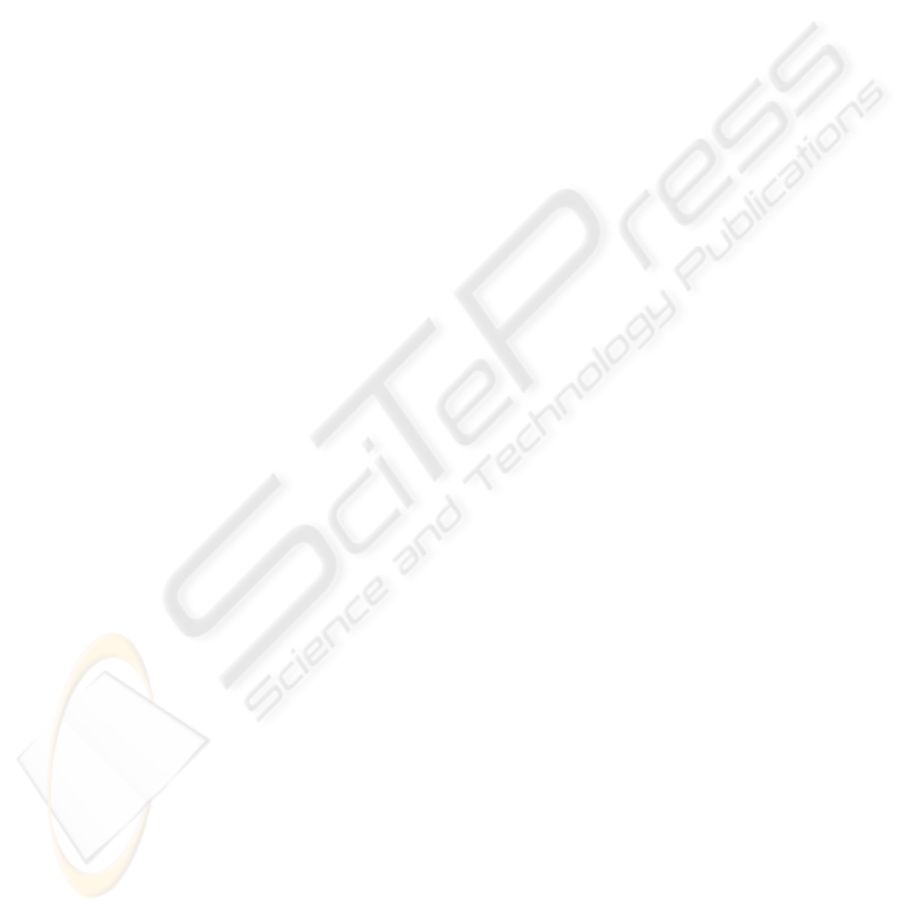
REFERENCES
Agrawal, R., Imielinski, T., and Swami, A. N. (1993). Min-
ing association rules between sets of items in large
databases. In Buneman, P. and Jajodia, S., editors,
Proceedings of the 1993 ACM SIGMOD International
Conference on Management of Data, pages 207–216,
Washington, D.C.
Agrawal, R., Mannila, H., Srikant, R., Toivonen, H., and
Verkamo, A. I. (1996). Fast discovery of association
rules. In Advances in knowledge discovery and data
mining, pages 307–328. American Association for Ar-
tificial Intelligence.
Bayardo, R. J. and Agrawal, R. (1999). Mining the most
interesting rules. In Proc. of KDD’99, pages 145–154,
San Diego, USA.
Ben-Yahia, S. and Mephu-Nguifo, E. (2004). Emulating
a cooperative behavior in a generic association rule
visualization tool. In Proc. of ICTAI’04, Boca Raton,
Florida, USA.
Blanchard, J., Guillet, F., and Briand, H. (2003). Ex-
ploratory visualization for association rule rummag-
ing. In Proceedings of the 4th International Workshop
on Multimedia Data Mining MDM/KDD’03, Wash-
ington.
Fayyad, U., Piatetsky-Shapiro, G., and Smyth, P. (1996).
From data mining to knowledge discovery: An
overview. In Advances in Knowlegde Discovery and
Data Mining, pages 1-34.
Freitas, A. A. (1999). On rule interestingness measures.
Knowledge-Based Systems journal, 12(5-6):309–315.
Han, J. and Fu, Y. (1995). Discovery of multiple-level
association rules from large databases. In Proc. of
VLDB’95, pages 420–431, Z
¨
urich, Switzerland.
Hilderman, R. J. and Hamilton, H. J. (2001). Knowledge
discovery and measures of interest. Kluwer Acae-
demic Publishers.
Hipp, J., Myka, A., Wirth, R., and G
¨
untzer, U. (1998). A
new algorithm for faster mining of generalized asso-
ciation rules. In Proc. of PKDD ’98, pages 74–82,
Nantes, France.
Klemettinen, M., Mannila, H., Ronkainen, P., Toivonen, H.,
and Verkamo, A. I. (1994). Finding interesting rules
from large sets of discovered association rules. In
Proc. of CIKM’94, pages 401–407. ACM Press.
Klemettinen, M., Mannila, H., and Toivonen, H. (1996).
Interactive exploration of discovered knowledge : A
methodology for interaction and usability studies.
Technical report, University of Helsinki.
Kuntz, P., Guillet, F., Lehn, R., and Briand, H. (2000). A
user-driven process for mining association rules. In
Proc. of PKDD’00, pages 160–168, Lyon, France.
Liu, B., Hsu, W., and Chen, S. (1997). Using general im-
pressions to analyze discovered classification rules. In
Proc. of KDD’97, pages 31–36. AAAI Press.
Liu, B., Hsu, W., Chen, S., and Ma, Y. (2000). Analyz-
ing the subjective interestingness of association rules.
IEEE Intelligent systems, 15(5):47–55.
Liu, B., Hsu, W., Mun, L.-F., and Lee, H.-Y. (1996). Find-
ing interesting patterns using user expectations. vol-
ume 11, pages 817–832.
Liu, B., Hsu, W., Wang, K., and Chen, S. (1999). Visually
aided exploration of interesting association rules. In
Proc. of PAKDD’99, pages 380–389, Beijing, China.
Matheus, C. J., Piatetsky-Shapiro, G., and McNeill, D.
(1996). Selecting and reporting what is interesting :
the kefir application to heathcare data. In Advances in
Knowlegde Discovery and Data Mining, pages 495–
516.
Morimoto, Y., Fukuda, T., Matsuzawa, H., Tokuyama, T.,
and Yoda, K. (1998). Algorithms for mining associa-
tion rules for binary segmentations of huge categorical
databases. In Proc. of VLDB’98, pages 380–391, New
York City, New York, USA.
Ohsaki, M., Kitaguchi, S., Okamoto, K., Yokoi, H., and Ya-
maguchi, T. (2004). Evaluation of rule interestingness
measures with a clinical dataset on hepatitis. In Proc.
of PKDD’04, pages 362–373, Pisa.
Padmanabhan, B. and Tuzhilin, A. (1998). A belief-driven
method for discovering unexpected patterns. In Press,
A., editor, Proc. of KDD’98, pages 94–100, New
York, USA.
Padmanabhan, B. and Tuzhilin, A. (1999). Unexpectedness
as a measure of interestingness in knowledge discov-
ery. Decision support system, 27.
Piatetsky-Shapiro, G. and Matheus, C. J. (1994). The inter-
estingness of deviations. In Proc. of KDD’94, pages
25–36, Seattle, USA.
Roddick, J. F. and Rice, S. (2001). What’s interesting about
cricket? on thresholds and anticipation in discovrerd
rules. SIKKDD Explorations, 3(1):1–5.
Shekar, B. and Natarajan, R. (2002). A fuzzy-graph-based
approach to the determination of interestingness of as-
sociation rules. In Proc. of PAKM’02, pages 377–388.
Silberschatz, A. and Tuzhilin, A. (1995). On subjective
measures of interestingness in knowledge discovery.
In Proc. of KDDM’95, pages 275–281, Menlo Park,
Californie, USA.
Silberschatz, A. and Tuzhilin, A. (1996). User-assisted
knowledge discovery : how much should the user be
involved. In Proc. of DMKD’96, Montreal, Canada.
Srikant, R. and Agrawal, R. (1997). Mining generalized as-
sociation rules. Future Generation Computer Systems,
13(2–3):161–180.
Srikant, R., Vu, Q., and Agrawal, R. (1997). Mining associ-
ation rules with item constraints. In Proc. of KDD’97,
pages 67–73.
Tan, P.-N., Kumar, V., and Srivastava, J. (2002). Selecting
the right interestingness measure for association pat-
terns. In Proc. of KDD’02, pages 32–41. ACM Press.
Tseng, S. (2001). Mining association rules with interesting-
ness constraints in large databases. In International
Journal of Fuzzy Systems, 3(2).
INTERACTIVE DATAMINING PROCESS BASED ON HUMAN-CENTERED SYSTEM FOR BANKING
MARKETING APPLICATIONS
109