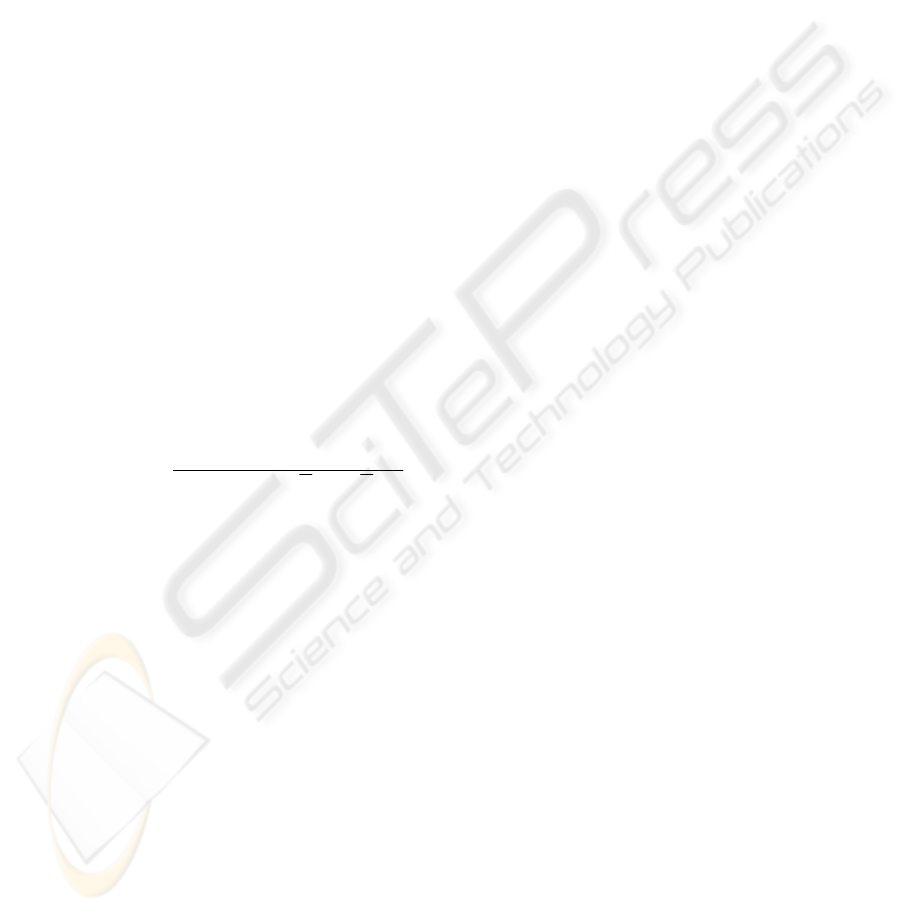
A conceptual model of the context of each
information source builds a basis for integration on
the semantic level. In this process, we take the
information about the context of the source
providing a new context description for that entity
within the new information source. Here, we focus
on context transformation by classification [8].
5.2 Mapping between Ontologies
In this system, we apply machine learning
techniques to semi-automatically create semantic
mappings. Since taxonomies are central components
of ontologies, we focus on finding correspondences
among the taxonomies of two given ontologies: for
each concept node in one taxonomy, find the most
similar concept node in the other taxonomy. The
first issue we address is the meaning of similarity
between two concepts. In our approach similarity
measure is based on the joint probability
distribution.
To match concepts between two taxonomies, we
need a measure of similarity. First, we would like
the similarity measures to be well-defined. Second,
we want the similarity measures to correspond to our
intuitive notions of similarity.
Many practical similarity measures can be
defined based on the joint distribution of the
concepts involved. A possible definition for the
exact similarity measure is
),(),(),(
)(/)(
),(
BAPBAPBAP
BAPBAP
BAsimJaccard
++
=−
UI
T
his similarity measure is known as the Jaccard
coefficient. It takes the lowest value 0 when A and B
are disjoint, and the highest value 1 when A and B
are the same concept [
Doan, 2002].
6 CONCLUSIONS
In this paper, we dealt with the problem of
information integration from different sources.
Integrating information from web sources starts by
extracting the data from the Web pages exported by
the data sources. So, we have proposed a
framework for extracting reliable data and to convert
standard form.
By using shared ontology, we also address
terminological semantic heterogeneity in semantic
integration. With the proliferation of data sharing
applications that involve multiple ontologies, the
development of automated techniques for ontology
matching will be crucial to their success.
REFERENCES
Bayrak C., Kolukısaoğlu H., 2003. Data Extraction from
Repositories on the Web: A Semi-automatic
Approach, Computer Science Department, University
of Arkansas at Little Rock, Little Rock, AR, U.S.A. ,
SEPTEMBER, Vol. 7, No. 4, pp. 13-23.
Cui Z., Jones D., Brien P. O, 2001. Issues in Ontology-
based Information Integration, Intelligent Business
Systems Research Group Intelligent Systems Lab.
Doan A., Madhavan J., Domingos P., Halevy A., 2002.
Learning to Map between Ontologies on the Semantic
Web. In Proceedings of the World-Wide Web
Conference (WWW-2002), pages 662-673, ACM
Press.
Embley D. W., Campbell D. M., Smith R. D., and Liddle
S. W., 1998. Ontology-based extraction and
structuring of information from data-rich unstructured
documents. In International Conference on
Information and Knowledge Management (CIKM).
Gruber T.R., 2003. A Translation Approach to Portable
Ontology Specification, Knowledge Acquisition, 199–
220.
Hendler J., Lee T.B., Miller E., 2002. Integrating
Application on the Semantic Web, Journal of the
Institute of Electrical Engineers of Japan, Vol. 122
(10).
Maedche A., 2002. Tying Up Information Integration and
Web Site Management by Ontologies, IEEE Data
Engineering Bulletin.
Stuckenschmidt H., 2002.
Information Sharing on the
Semantic Web, AI Department, Vrije University,
Amsterdam, De Boelelaan 1081a, 1081HV
Amsterdam, The Netherlands.
Soderland S., 1998. Learning information extraction rules
for semi-structured and free text,
www.cs.washington.edu/homes/soderlan/ WHISK.
Staab S., Maedche A., 2001. Comparing Ontologies
Similarity Measures and a Comparison Study, Internal
Report No. 408.
Staab S., 2002. The Semantic Web-New Ways to Present
and Integrate Information, Institute of Applied
Informatics and Formal Description Methods (AIFB),
University of Darlsruhe.
Wache H., Vögele T., Visser U., Stuckenschmidt H.,
Schuster G., Neumann H., Hübner S., 2001.
Ontology-Based Integration of Information: A Survey
of Existing Approaches.
ICEIS 2005 - DATABASES AND INFORMATION SYSTEMS INTEGRATION
460