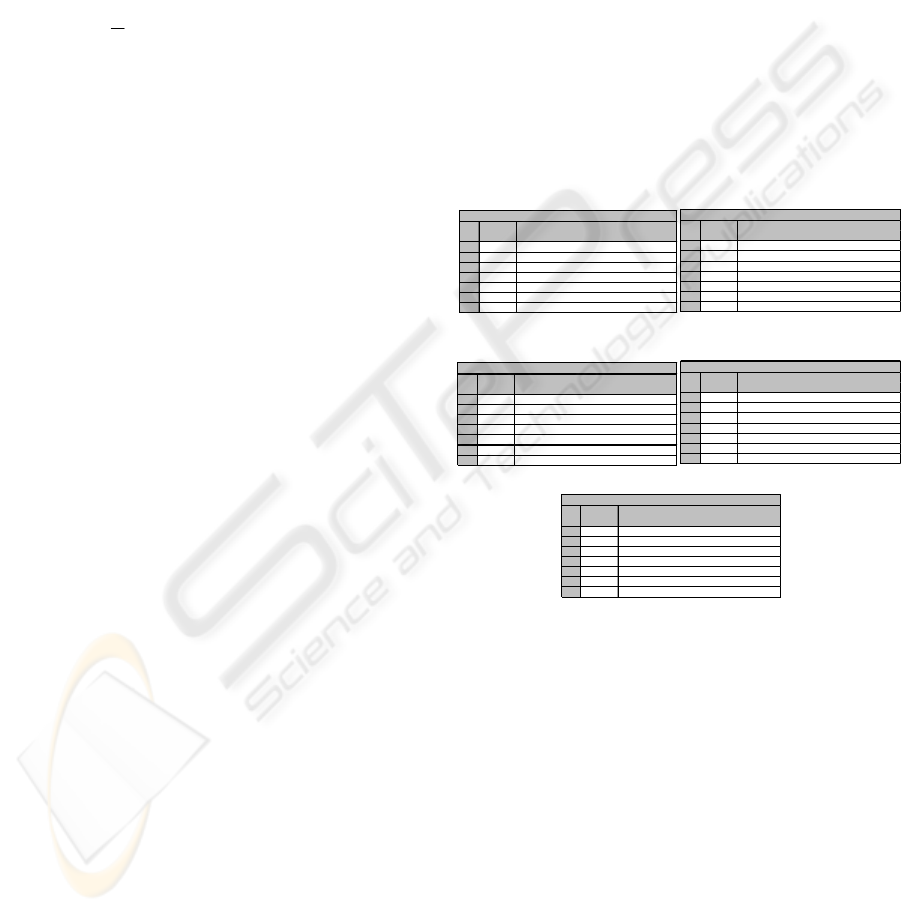
language by using a probability model M. We need
the cross-entropy because, the exact probability
distribution of a language is never certain. Hence,
H(L) is just a theoretical value. The cross-entropy of
a language L calculated using a model M is
formulated in Eq. 1. The entropy and cross-entropy
are both measured in bits per character – bpc
.
H
In Eq. 1, the entropy of a language L, i.e. H(L) is
calculated as follows (Eq. 2)
:
Entropy H in Eq. 1 is the average number of bits
per symbol needed to encode a message (Shannon,
1948)
.
Assuming that the probabilities summing to 1 are
independent and the k possible symbols have a
probability distribution P=p(x
1
), p(x
2
), …, p(x
k
), the
entropy can be calculated as below (Eq. 3):
For this study, we incorporated Corpora from five
European languages as English, French, Turkish,
German and Spanish. These languages differ from
each other to varying degrees. For example, while
English, French and Spanish are more alike, Turkish
is totally different from these languages and German
has some common linguistic characteristics with the
above mentioned group of three. The alphabet size
for these languages are 26 letters for English and
French, 29 letters for Turkish and 30 letters for both
German and Spanish.
All languages of concern in this study are
analytical, agglutinative and fusional (having
affixes). Analytical languages either does not
combine concepts into single words at all (like in
Chinese) or does so economically as is the case in
English and French. The sentence itself is of primary
concern in analytical languages, while the word is of
minor interest. Turkish is synthetic and a free
constituent order language, morphologically
extendible with the its rich set of derivational and
inflectional suffixes. In a synthetic language, the
concepts cluster more thickly, the words are more
richly chambered, but there is a tendency to keep the
range of concrete significance in the single word
down to a moderate compass (Sapir, 1921). German
has extensive use of inflectional endings and
compound words are quite common (German
Linguistic URL). Spanish has quite the same
linguistic characteristics as French, with higher
average word length.
Our Corpora consists of these five different
languages and each language has a group of seven
texts. The texts within each language have been
deliberately selected from different essay categories
to reflect the changes of the style into our language
discrimination implementation. These categories are
novel, technical document, poetry, manual, theatre
text, Holy book (Bible or Qoran) and a
dictionary/encyclopedia. We first based our Corpora
construction on text files from the standard English
Cor-pus Canterbury (Canterbury Corpus). Modeling
after the Canterbury Corpus, we then compiled
Corpora from the other four source languages as
French, Turkish, German and Spanish. The texts in
Turkish Corpus are from Celikel (Celikel, 2004),
and the rest of the texts in other languages are all
from Internet. The sizes and contents of each Corpus
are listed in Tables 1 through Table 5
:
∑
−= ),...,,(log),...,,(),(
2121 mMmM
xxxpxxxpML
1
∑
−
=
∞→
),...,,(log),...,,(
1
lim
)(
2121 mm
m
xxxpxxxp
m
LH
2
∑
=
−=
k
i
ii
xpxpPH
1
)(log)()(
3
Table 1: English Corpus Table 2: French Corpus.
Table 3: Turkish Corpus Table 4: German Corpus
Table 5: Spanish Corpus
3 RESULTS
To discriminate among languages, we applied the
PPM model on texts from each Corpus. During
implementation, we repeated the language
identification experiments on each text file. Within
each language set, we employed each of seven text
files as the training text to PPM to compress the
texts of the whole Corpora. Since there are five
different languages, it makes 7x5=35 runs for each
text; since there are seven texts within each language
set, it makes 7x5x7=245 runs for each language; and
since there are five different languages, it makes
245x5=1,225 runs in total.
In order to evaluate the performance of our
language discriminator, we used the accuracy rate
measure (Eq. 4). In this formula, the successes are
the cases when both the training text and the
EN G L I S H
Fi l e s i z e
(bytes)
E1
152,089 Novel: Lewis Carroll's "Alice in Wonderland"
E2
426,754 Technical document
E3
481,861 English poetry
E4
4,227 GNU manual
E5
125,179 Theatre text of the play "As You Like it"
E6
4,047,392 Bible in English
E7
2,473,401 World Fact Book of CIA
l e Expl an at io nFi
FRENC H
Fi l e si z e
(bytes)
F1
871,286 Novel: Jules Verne's "20000 Leagues under the Sea"
F2
66,049 Technical document
F3
185,205 French poetry
F4
32,428 GNU manual
F5
135,477 T heatre t ext of the play "T artuffe" by Molière
F6
4,669,107 Bible in French
F7
51,521 French dictionary of computers
Fi le Expl an ati on
TU R KI S H
Fi l e si z e
(bytes)
167,799 Novel: Ataturk's Discourse
9,664 Technical document
59,386 Turkish poetry
18,526 GNU manual
113,545 T heat re t ext of t he play "Galilei Galileo"
937,532 Quran in T urkish
765,624 Online Philosophy terms disctionary
ile Explanation
T1
F
T2
T3
T4
T5
T6
T7
GERMAN
Fi l e si z e
(bytes)
G1
716,800 Novel
G2
25,872 Technical document
G3
105,868 German poetry
G4
4,622 GNU manual
G5
100,712 T heatre t ext of the play "Faust"
G6
4,359,878 Bible in German
G7
157,289 Online dictionary of medical terms
Fi le Expl an ati on
SPANISH
Fi l e si z e
(bytes)
S1
63,170 Novel "Oración Cívica" by Gabino Barreda
S2
25,295 Technical document
S3
129,005 Spanish poetry
S4
5,865 GNU manual
S5
158,991 T heatre text of t he play "Las Mocedades Del Cid"
S6
4,126,848 Bible in Spanish
S7
216,267 Online ophtalmology dictionary
File Explanation
A CRYPTOGRAPHIC APPROACH TO LANGUAGE IDENTIFICATION: PPM
215