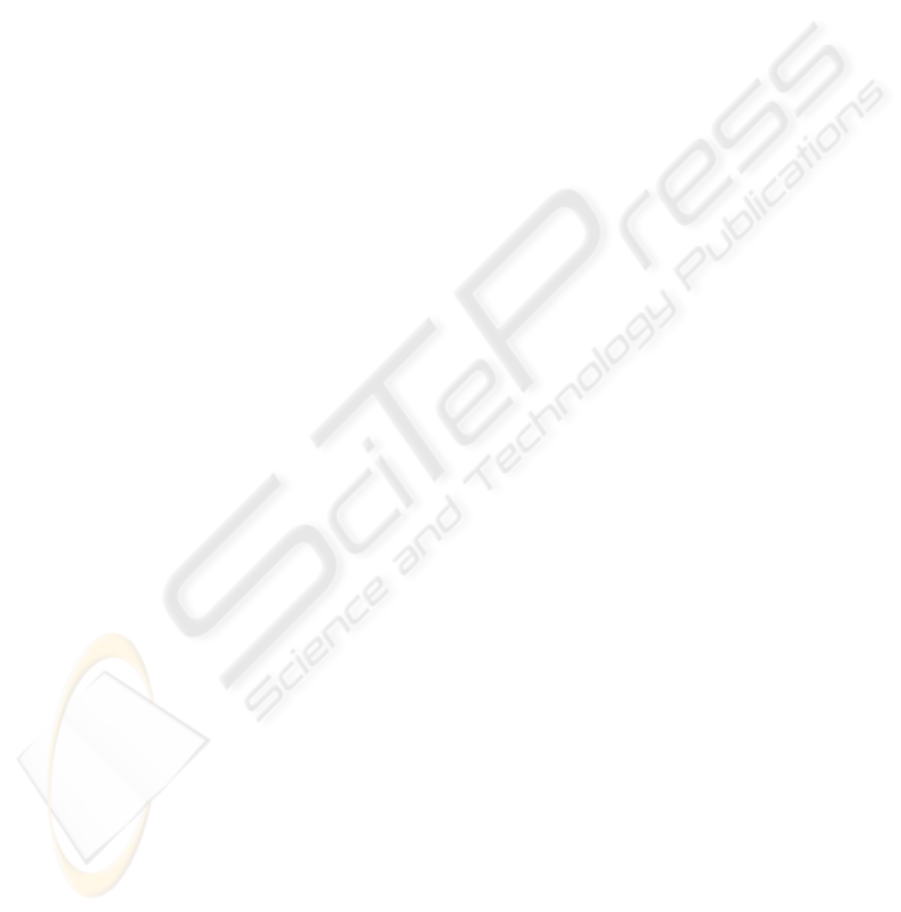
where
)()()( ttt
εε
XXX −=
)0()0(
ε
XX −=
for almost all initial difference vectors ε = X(0) – X
ε
(0). We estimated λ
1
using the
algorithm of Sano and Sawada [3]. λ
1
describes the divergence and instability of the
orbits in the phase space.
The largest Lyapunov exponents, λ
1
, were calculated for a basic window of 8,000
points (40 sec). The 180 sec (36,000 points) were covered by sequentially sliding,
200 points (1 sec) at a time and λ
1
was determined for each window.
4 Activities of daily living (ADL) and dementia level data of the
elderly subjects
Data recorded by the persons caring for the subjects in the care home was used as the
ADL data. The dementia data used were those recorded by healthcare professionals.
For the ADL, each activity of daily living, such as walking, eating, toileting, bathing,
dressing, and grooming was assigned to one of three care dependence categories: a)
can do independently although it takes a long time; b) needs some assistance; and c)
totally dependent on assistance. Each subject’s communication skill was also as-
signed to one of the three levels: a) can communicate normally; b) can communicate
to some extent; and c) can hardly communicate.
Data on dementia obtained from the healthcare professionals were categorized into
the five grades of dementia: 0: none, 1: mild, 2: moderate, 3: severe, and 4: very se-
vere.
5 Pulse wave parameters and analysis of ADL indices and severity
of dementia
We examined whether the values of the largest Lyapunov exponent (group means and
standard deviations) had any significant correlation with each of the factors—sex,
age, body temperature, systolic blood pressure, diastolic blood pressure, pulse rate,
walking, eating, toileting, bathing, dressing, grooming, communication skill, and
severity of dementia—in order to examine the relationship between the ADL indices
and dementia with the pulse wave data.
We found that only communication skill and the severity of dementia were signifi-
cantly correlated with the mean and standard deviation of the Lyapunov exponent.
Figs. 1 and 2 show the relationship of these factors with mean and standard deviation
of the Lyapunov exponent, respectively.
The Lyapunov exponent is a measure of the divergence of the attractor trajectory. It
became clear from the above results that communication skill and dementia were
related to the deviation of the Lyapunov exponent and that elderly persons who could
194