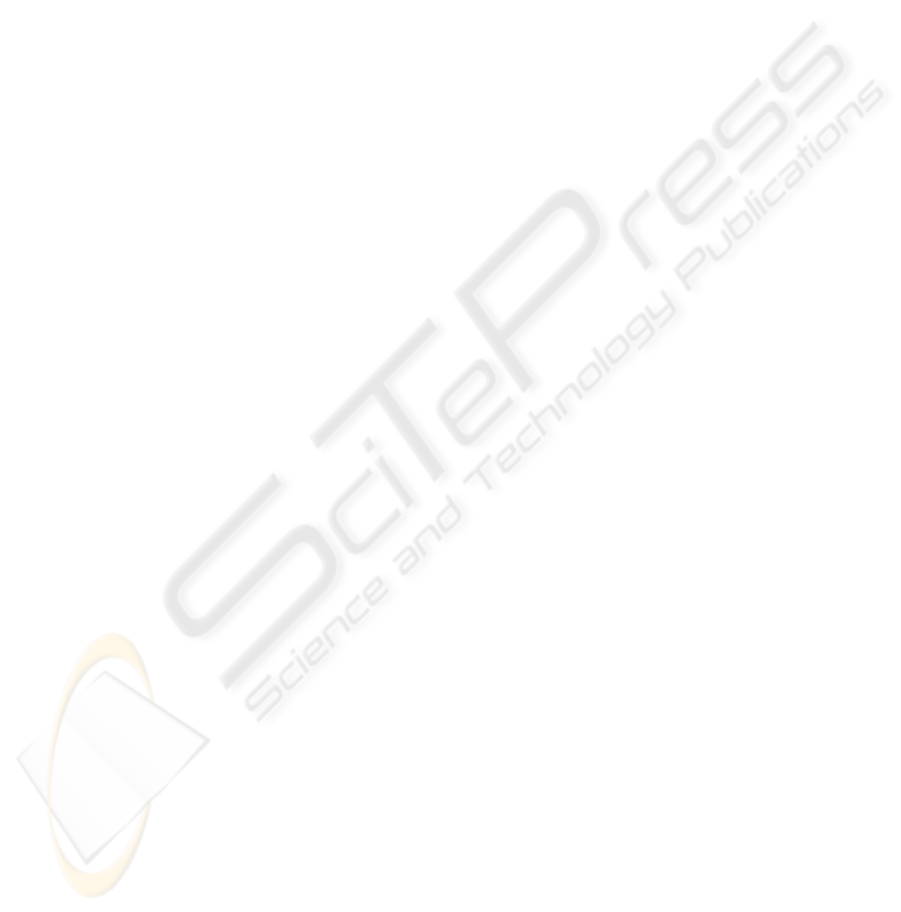
5 Conclusion
We proposed a noticeable performances improvement of a neural classifier based an
RBF network. The new classifier is very general and simple. It generates automati-
cally a powerful RBF network without any introduction of parameters fixed a priori.
The number of hidden neurons is very optimized what will allow its use for the very
large databases. Indeed, the new classifier obtains excellent recognition results for a
variety of different databases and particularly the buried tag recognition. On this
application we can also note a reduction in the error rate (relatively weak) but espe-
cially a very clear reduction in the number of hidden neurons (division by 2). This
allows a notable saving of the training times necessary to the development of the
system.
References
1. D.S. Broomhead and D. Lowe, Multivariable functional interpolation and adaptive net-
works, Complex Systems, Vol.2, 1988, pp. 321-355.
2. J. Moody and C.J. Darken, Fast Learning in Networks of Locally-Tuned Processing Units.
Neural Computation, Vol.1, 1988, pp. 281-294.
3. F. Girosi and T. Poggio, Networks and The Best Approximation Property, Technical Re-
port C.B.I.P. No. 45, Artificial Intelligence Laboratory, Massachusetts Institute of Tech-
nology, 1989.
4. Park J. and Sandberg I.W., Universal Approximation Using Radial-Basis-Function Net-
works, Neural Computation, Vol.3, 1991, pp. 246-257.
5. M. Bianchini, P. frasconi and M. Gori, Learning without Local Minima in Radial Basis
Function Networks, IEEE Transactions on Neural Networks, Vol.6:3, 1995, pp. 749-756.
6. B. Fritzke, Supervised Learning with Growing Cell Structures, In Advances in Neural
Processing Systems 6, J.C. Cowan, Tesauro G. and Alspector J. (eds.), Morgan Kaufmann,
San Mateo, CA., 1994.
7. B. Fritzke, Transforming Hard Problems into Linearly Separable one with Incremental
Radial Basis Function Networks, In M.J. Vand Der Heyden, J. Mrsic-Flögel and K. Weigel
(eds.), HELNET International Workshop on Neural Networks, Proceedings Volume I/II
1994/1995, VU University Press, 1996
8. C.G. Looney, Pattern Recognition Using Neural Network - Theory and Algorithms for
Engineers and Scientits, Oxford University Press, Oxford - New York, 1997.
9. F. Belloir, A. Fache and A. Billat, A General Approach to Construct RBF Net-Based Clas-
sifier, Proc. of the European Symposium on Artificial Neural Networks (ESANN’99),
April 21-23, Bruges Belgium, 1999, pp. 399-404.
10. C. Aviles-Cruz, A. Guerin-Dugué, J.L. Voz and D. Van Cappel, Deliverable R3-B1-P Task
B1: Databases, Technical Report ELENA ESPRIT Basic Research Project Number 6891,
June 1995.
11. F. Blayo, Y. Cheneval, A. Guerin-Dugué, R. Chentouf, C.Aviles-Cruz, J. Madrenas,
M. Moreno and J.L. Voz, Deliverable R3-B4-P Task B4: Benchmarks, Technical Report
ELENA ESPRIT Basic Research Project Number 6891, June 1995.
12. Merz, C.J. and Murphy, P.M. (1996). UCI Repository of machine learning databases.
[http://www.ics.uci.edu/~mlearn/MLRepository.html]. Irvine, CA: University of Califor-
31