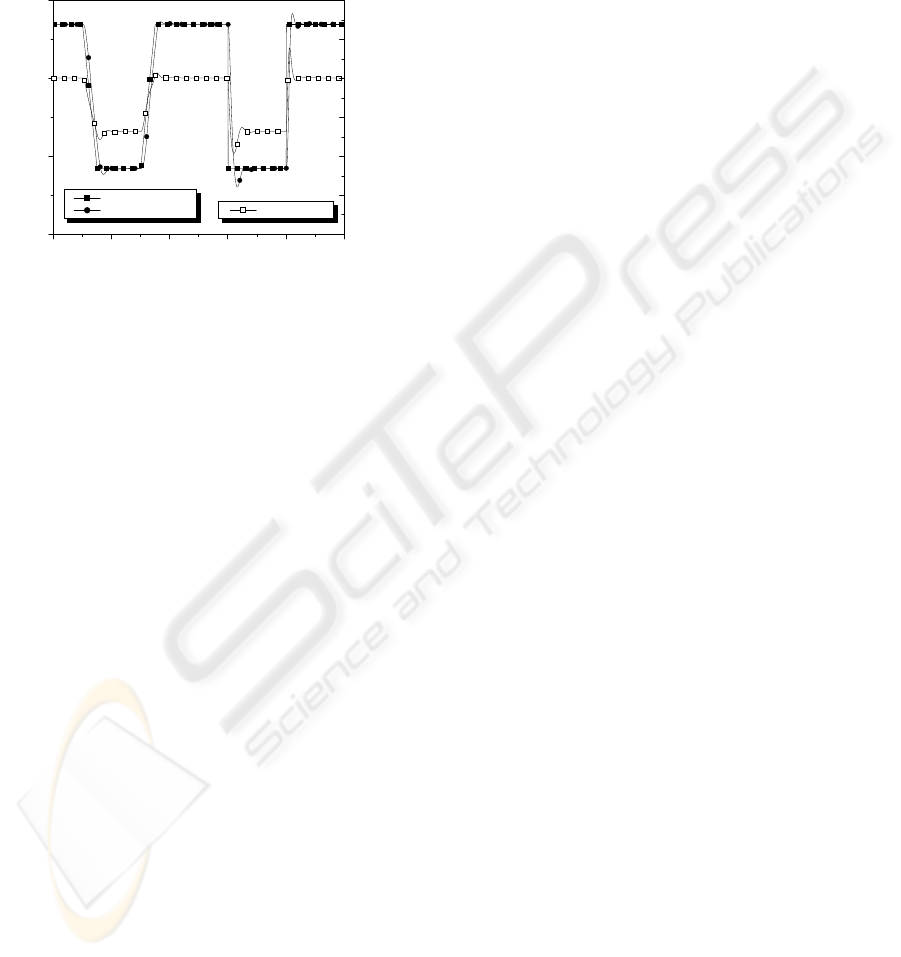
In addition, a conventional proportional-integral
(PI) controller was designed to compare the
performance of the power level response with the
proposed model predictive controller optimized by
the GA (refer to Fig. 4). The PI controller has a little
slower response and bigger overshoot and
undershoot than the proposed MPC.
0 200 400 600 800 1000
30
60
90
120
thermal reactor power (MW)
TE power (KW)
time(sec)
TE demand power
TE power
0.0
0.5
1.0
1.5
2.0
2.5
3.0
reactor power
Figure 4: Performance of a PI controller.
4 CONCLUSIONS
In this work, the model predictive controller
optimized by the GA and combined by SVMs was
developed to control the nuclear power in the SP-
100 space reactor system. The future TE power is
predicted by using the SVMs and the GA was used
to optimize the model predictive controller. It was
determined from many numerical simulation results
that the proposed controller was able to actuate the
control drum to regulate the control reactivity so that
the TE generator electric power followed the set
point changes according to load demands. Also, the
performance of the new proposed controller was
proved to be more efficient than that of the
conventional PI controller.
ACKNOWLEDGEMENTS
The research is supported in part by Korea MOST
(Ministry of Science and Technology) BAERI grant
and in part by a U.S. Department of Energy NEER
grant (DE-FG07-04ID14589) with the University of
Tennessee.
REFERENCES
Cho, N.Z., Grossman, L.M., 1983. Optimal Control for
Xenon Spatial Oscillations in Load Follow of a
Nuclear Reactor. Nucl. Sci. Eng., Vol. 83, pp. 136-
148.
Demuth, S.F., 2003. SP-100 Space Reactor Design.
Progress in Nuclear Energy, Vol. 42, No. 3, pp. 323-
359.
Garcia, C.E., Prett, D.M., Morari, M., 1989. Model
Predictive Control: Theory and Practice – A Survey.
Automatica, Vol. 25, No. 3, pp. 335-348.
Goldberg, D.E., 1989. Genetic Algorithms in Search,
Optimization, and Machine Learning, Addison
Wesley, Reading, Massachusetts.
Kwon, W.H., Pearson, A.E., 1977. A Modified Quadratic
Cost Problem and Feedback Stabilization of a Linear
System. IEEE Trans. Automatic Control, Vol.22, No.
5, pp. 838-842.
Na, M.G., Shin, S.H., Kim, W.C., 2003. A Model
Predictive Controller for Nuclear Reactor Power. J.
Korean Nucl. Soc., Vol. 35, No. 5, pp. 399-411.
Pai, P.-F., Hong, W.-C., 2005. Support Vector Machines
with Simulated Annealing Algorithms in Electricity
Load Forecasting. Energy Conversion and
Management, Vol. 46, pp. 2669-2688.
Sarimveis, H., Bafas, G., 2003. Fuzzy Model Predictive
Control of Non-linear Processes Using Genetic
Algorithms. Fuzzy Sets Systems, Vol. 139, pp. 59-80.
Shtessel, Y.B., 1998. Sliding Mode Control of the Space
Nuclear Reactor System. IEEE Trans. Aerospace and
Electronic Systems, Vol. 34, No. 2, pp. 579-589.
Vapnik, V., 1995. The Nature of Statistical Learning
Theory, Springer, New York.
Yan, W., Shao, H., Wang, X., 2004. Soft Sensing
Modeling Based on Support Vector Machine and
Bayesian Model Selection. Computers and Chemical
Engineering, Vol. 28, pp. 1489-1498.
A MODEL PREDICTIVE CONTROLLER BASED ON SUPPORT VECTOR REGRESSION AND GENETIC
OPTIMIZATION FOR AN SP-100 SPACE NUCLEAR REACTOR
141