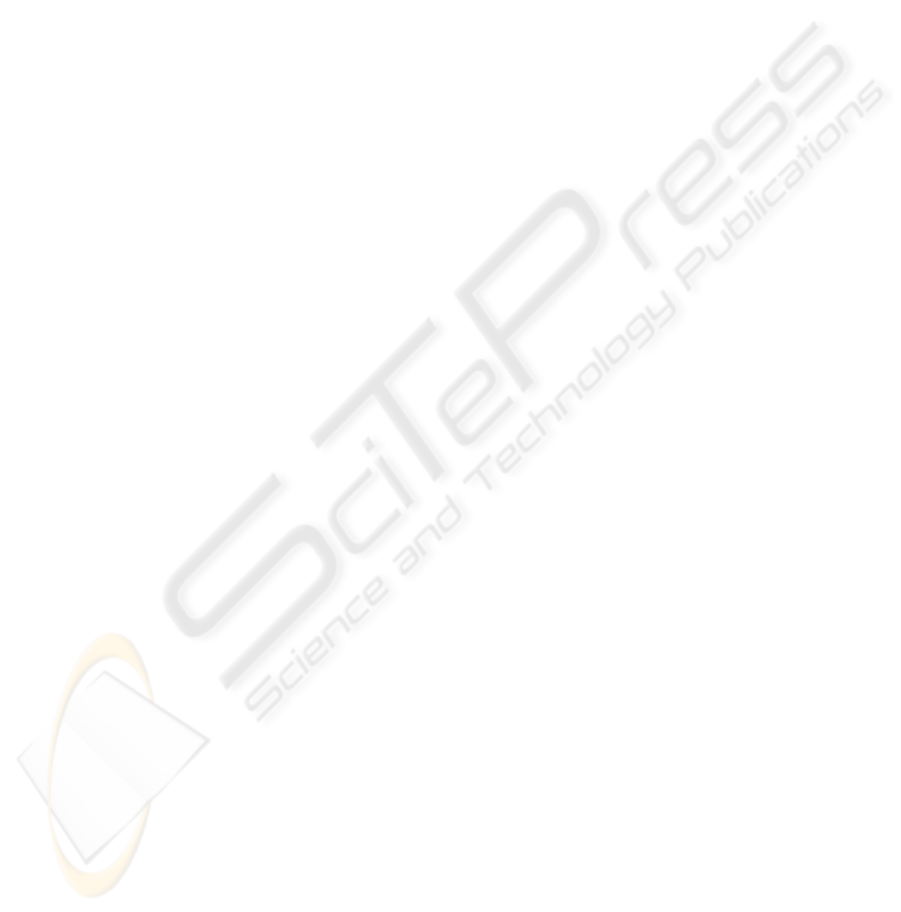
References
1. Bishop, C. M. (1995). Neural Networks for Pattern Recognition. Oxford University Press.
2. Castelli, V. and Cover, T. (1995). On the exponential value of labeled samples. Pattern
Recognition Letters, 16:105–111.
3. Cohn, D., Ghahramani, Z., and Jordan, M. (1996). Active learning with statistical models.
Journal of Artificial Inteligence Research, 4:129–145.
4. Constantinopoulos, C. and Likas, A. (2006). An incremental training method for the proba-
bilistic RBF network. IEEE Trans. Neural Networks, to appear.
5. Dempster, A., Laird, N., and Rubin, D. (1977). Maximum likelihood estimation from in-
complete data via the EM algorithm. Journal of the Royal Statistical Society, Series B,
39(1):1–38.
6. Freund, Y., Seung, H. S., Shamir, E., and Tishby, N. (1997). Selective sampling using the
query by comitee algorithm. Machine Learning, 28:133–168.
7. McCallum, A. K. and Nigam, K. (1998). Employing EM in pool-based active learning for
text classification. In Shavlik, J. W., editor, Proc. 15th International Conference on Machine
Learning. Morgan Kaufmann.
8. McLachlan, G. and Krishnan, T. (1997). The EM algorithm and extensions. John Wiley &
Sons.
9. McLachlan, G. and Peel, D. (2000). Finite Mixture Models. John Wiley & Sons.
10. Titsias, M. K. and Likas, A. (2000). A probabilistic RBF network for classification. In Proc.
International Joint Conference on Neural Networks 4, pages 238–243. IEEE.
11. Titsias, M. K. and Likas, A. (2001). Shared kernel models for class conditional density
estimation. IEEE Trans. Neural Networks, 12(5):987–997.
12. Titsias, M. K. and Likas, A. (2002). Mixture of experts classification using a hierarchical
mixture model. Neural Computation, 14(9):2221–2244.
13. Titsias, M. K. and Likas, A. (2003). Class conditional density estimation using mixtures with
constrained component sharing. IEEE Trans. Pattern Anal. and Machine Intell., 25(7):924–
928.
14. Zhu, X., Lafferty, J., and Ghahramani, Z. (2003). Combining active learning and semi-
supervised learning using Gaussian fields and harmonic functions. In Proc. 20th Interna-
tional Conference on Machine Learning.
12