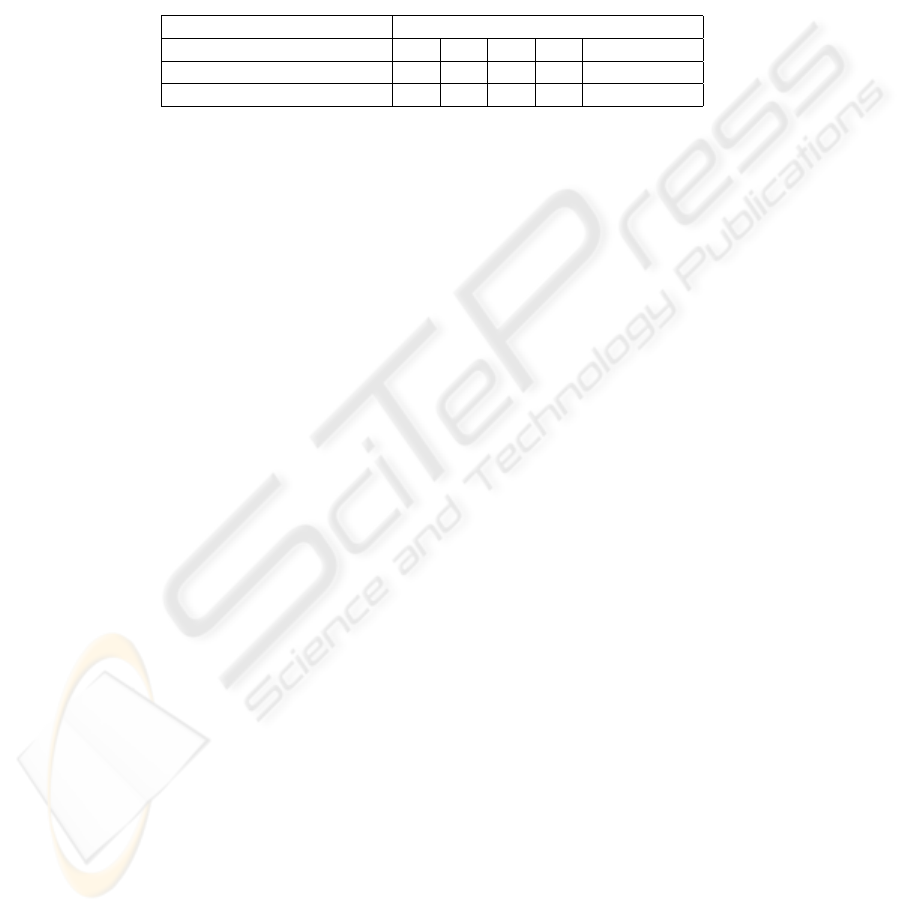
4 Results and Observations
A result of testing the network using five set of experiments are tabulated below. The
classification accuracy was 100% for all the experiments. The results are outlined in
table (3).
Table 3. Neural network testing results.
Action Performed Action identified for each sub experiments
Wrist flexion 100% 100% 100% 100% 100%
Finger Flexion 100% 100% 100% 100% 100%
Finger flexion and wrist flexion 100% 100% 100% 100% 100%
5 Discussions and Conclusion
A new approach that combines semi-blind ICA along with neural networks was used
to separate and identify hand gestures. The results demonstrate that the technique can
be effectively used to identify hand gestures based on surface EMG when the level of
activity is very small. The authors would like to mention that this is early stage of the
work, and work needs to be done to identify inter-day variations. It is also important to
test the technique for different actions, and for a large group of people. Further, there is
need to automate the semi-blind operation.
References
1. Schlenzig, J., Hunter, E., Jain, R. : Vision based hand gesture interpretation using recursive
estimation, Vol. 2. Twenty-Eighth Asilomar Conference on Signals, Systems and Computers,
(1994) 1267 - 1271
2. Rehg, J. M., Kanade, T. : Vision-based hand tracking for human-computer interaction, IEEE
Workshop on Motion of Non-Rigid and Articulated Objects, (1994) 16 - 22
3. Pavlovic, V. I., Sharma, R., Huang, T. S. : Visual interpretation of hand gestures for human-
computer interaction, Vol. 19. IEEE Transactions on Pattern Analysis and Machine Intelli-
gence, (1997) 677 - 695
4. Cheron, G., Draye, J., Bourgeios, M., Libert, G. : A Dynamic Neural Network Identification
of Electromyography and Arm Trajectory Relationship During Complex Movements, Vol.
43. IEEE Trans. Biomed. Eng, (1996) 552 - 558
5. Koike, Y., Kawato, M. : Human Interface Using Surface Electromyography Signals, Vol. 79.
Electronics and Communications, Japan, (1996) 15 - 22
6. Djuwari, D., Kumar, D. K., Polus, B., Raghupathy, S. : Multi-step independent component
analysis for removing cardiac artefacts from back semg signals, 8th Australian and New
Zealand Intelligent Information Systems Conference, Australia, (2003)
7. Greco, A., Costantino, D., Morabito, F. C., Versaci, M. A. : A Morlet wavelet classification
technique for ICA filtered SEMG experimental data, Vol. 1. Neural Networks Proceedings
of the International Joint Conference, (2003) 66 - 71
66