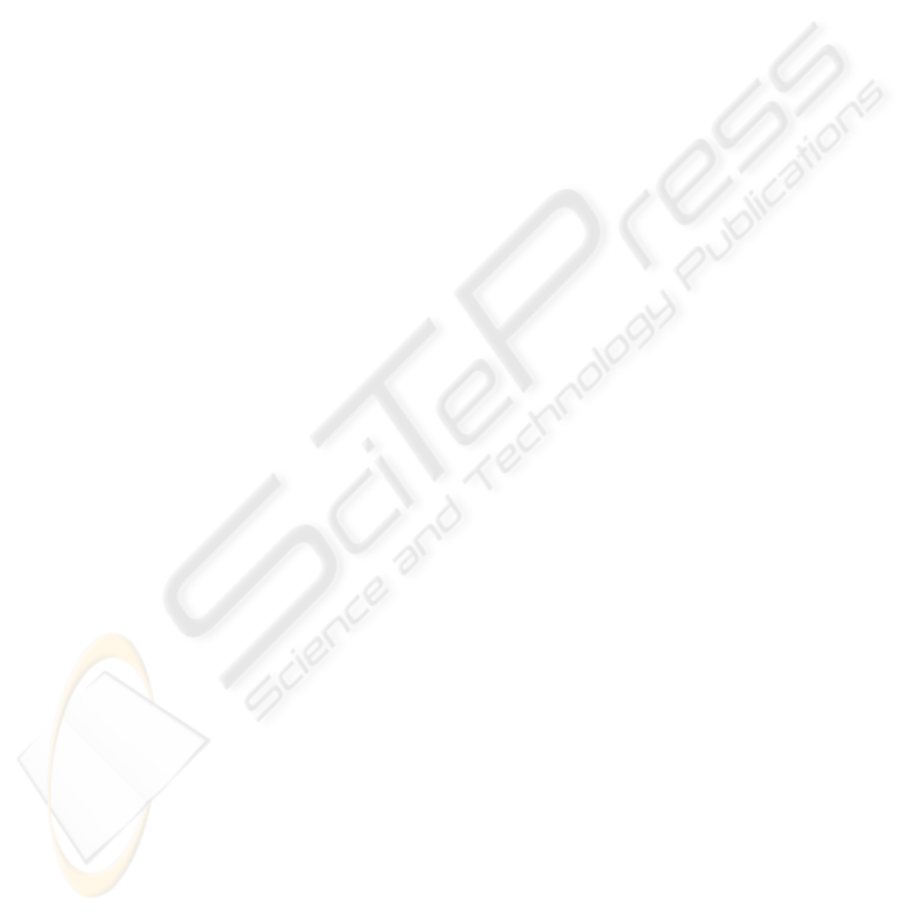
problem is cast in a hypothesis testing framework, linked to information theory. The
resulting classifier is based on the evaluation of the mutual information between the
audio signal and the mouths’ video features with respect to a threshold, issued from
the Neyman-Pearson lemma. A confidence level can then be assigned to the classifier
outputs. This approach results in the definition of an evaluation framework. The latter is
not used to determine the performance of the classifier itself, but considers rather rating
the whole classification process efficiency.
In particular, it is used to check whether a feature extraction step performed prior to
the classification can increase the accuracy of the detection process. Optimized audio
features obtained through an information theoretic feature extraction framework fed in
the classifier, in turn with non-optimized audio features. Analysis tools derived from hy-
pothesis testing, such as ROC graphs, establish eventually the performance gain offered
by introducing the feature extraction step in the process.
As far as the classifier itself is concerned, more intensive tests should be performed
in order to draw robust conclusions. However, preliminary remarks tend to indicate that
a hypothesis-based model can be used with advantage for multimodal speaker detection.
It would also be interesting to consider in future works the cases of simultaneous
silent or speaking states (cases 3 and 4 defined in sec. 3).
References
1. Hershey, J., Movellan, J.: Audio-vision: Using audio-visual synchrony to locate sounds. In:
Proc. of NIPS. Volume 12., Denver, CO, USA (1999) 813–819
2. Nock, H.J., Iyengar, G., Neti, C.: Speaker localisation using audio-visual synchrony: An em-
pirical study. In: Proceedings of the International Conference on Image and Video Retrivial
(CIVR), Urbana, IL, USA (2003) 488–499
3. Butz, T., Thiran, J.P.: From error probability to information theoretic (multi-modal) signal
processing. Signal Processing 85 (2005) 875–902
4. Fisher III, J.W., Darrell, T.: Speaker association with signal-level audiovisual fusion. IEEE
Transactions on Multimedia 6 (2004) 406–413
5. Besson, P., Popovici, V., Vesin, J.M., Kunt, M.: Extractionof audio features specific to speech
using information theory and differential evolution. EPFL-ITS Technical Report 2005-018,
EPFL, Lausanne, Switzerland (2005)
6. Ihler, A.T., Fisher III, J.W., Willsky, A.S.: Nonparametric hypothesis tests for statistical
dependency. IEEE Transactions on Signal Processing 52 (2004) 2234–2249
7. Moon, T.k., Stirling, W.C.: Mathematical Methods and Algorithms for Signal Processing.
Prentice hall (2000)
8. Meynet, J., Popovici, V., Thiran, J.P.: Face detection with mixtures of boosted discriminant
features. Technical Report 2005-35, EPFL, 1015 Ecublens (2005)
9. Fawcett, T.: Roc graphs: Notes and practical considerations for researchers. Technical Report
HPL-2003-4, HP Laboratories (2003)
10. Patterson, E., Gurbuz, S., Tufekci, Z., , Gowdy, J.: Cuave: a new audio-visual database for
multimodal human-computer interface research. In: Proceedings of International Conference
on Acoustics, Speech, and Signal Processing (ICASSP ). Volume 2., Orlando, IEEE (2002)
2017–2020
11. Besson, P., Monaci, G., Vandergheynst, P., Kunt, M.: Experimental evaluation framework for
speaker detection on the cuave database. Technical Report TR-ITS-2006.003, EPFL, 1015
Ecublens (2006)
115