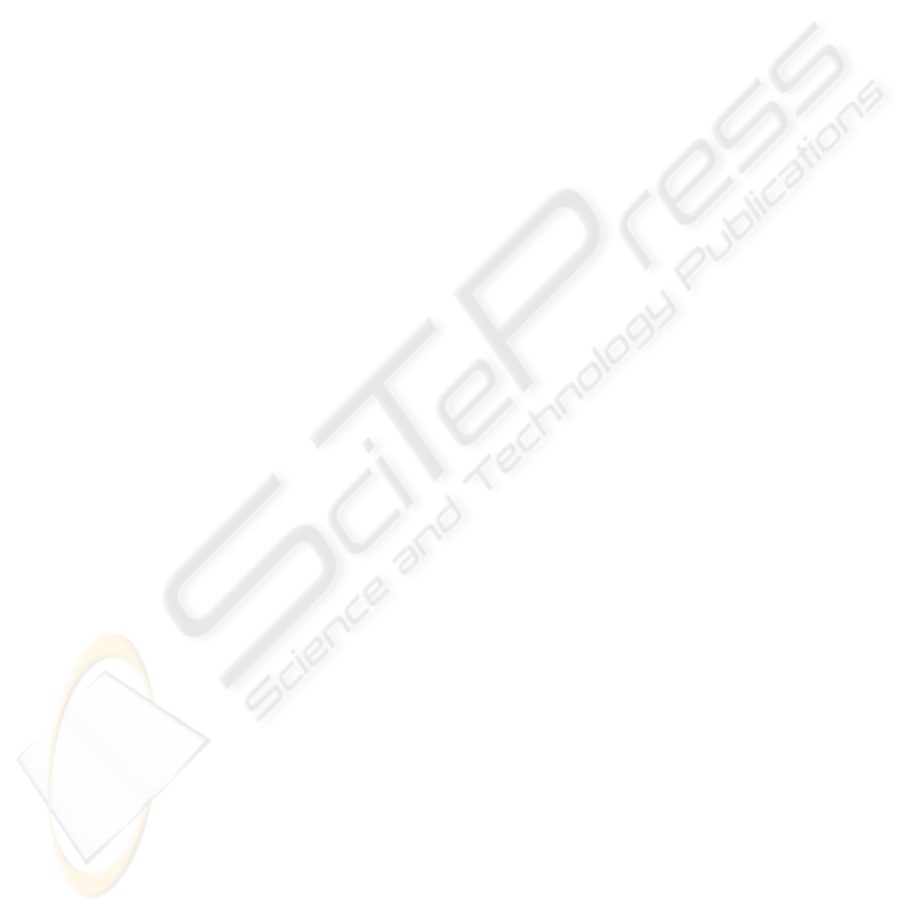
ally, progress in applying the constrained GPCA to
estimating more complicated multi-component struc-
tures will strongly depend on successful identification
of relevant constraints.
REFERENCES
(2003). Proc. IEEE Conf. Computer Vision and Pattern
Recognition.
Brooks, M. J., Chojnacki, W., Gawley, D., and van den Hen-
gel, A. (2001). What value covariance information in
estimating vision parameters? In Proc. Eighth Int.
Conf. Computer Vision, volume 1, pages 302–308.
Chojnacki, W., Brooks, M. J., van den Hengel, A., and
Gawley, D. (2000). On the fitting of surfaces to data
with covariances. IEEE Trans. Pattern Anal. Mach.
Intell., 22(11):1294–1303.
Chojnacki, W., Brooks, M. J., van den Hengel, A., and
Gawley, D. (2004a). From FNS and HEIV: A link be-
tween two vision parameter estimation methods. IEEE
Trans. Pattern Anal. Mach. Intell., 26(2):264–268.
Chojnacki, W., Brooks, M. J., van den Hengel, A., and
Gawley, D. (2004b). A new constrained parameter es-
timator for computer vision applications. Image and
Vision Computing, 22:85–91.
Chojnacki, W., Brooks, M. J., van den Hengel, A., and
Gawley, D. (2005). FNS, CFNS and HEIV: A
unifying approach. J. Math. Imaging and Vision,
23(2):175–183.
Duda, R. O. and Hart, P. E. (1972). Use of the Hough trans-
form to detect lines and curves in pictures. Commun.
ACM, 15:11–15.
Fitzgibbon, A., Pilu, M., and Fisher, R. B. (1999). Direct
least square fitting of ellipses. IEEE Trans. Pattern
Anal. Mach. Intell., 21(5):476–480.
Forsyth, D. A. and Ponce, J. (2003). Computer Vision: A
Modern Approach. Prentice Hall.
Hal
´
ı
ˇ
r, R. and Flusser, J. (1998). Numerically stable direct
least squares fitting of ellipses. In Proc. Sixth Int.
Conf. in Central Europe on Computer Graphics and
Visualization, pages 125–132.
Ho, J., Yang, M.-H., Lim, J., Lee, K.-C., and Kriegman,
D. J. (2003). Clustering appearances of objects un-
der varying illumination conditions. In (CVP, 2003),
pages 11–18.
Horn, R. and Johnson, C. (1985). Matrix Analysis. Cam-
bridge University Press, Cambridge.
Kanatani, K. (1996). Statistical Optimization for Geometric
Computation: Theory and Practice. Elsevier, Amster-
dam.
Leedan, Y. and Meer, P. (2000). Heteroscedastic regression
in computer vision: Problems with bilinear constraint.
Int. J. Computer Vision, 37(2):127–150.
Leonardis, A., Bischof, H., and Maver, J. (2002). Multiple
eigenspaces. Pattern Recognition, 35(11):2613–2627.
Lim, M. H. (1992). Conditions on decomposable symmetric
tensors as an algebraic variety. Linear and Multilinear
Algebra, 32:249–252.
Lou, X.-M., Hassebrook, L. G., Lhamon, M. E., and Li,
J. (1997). Numerically efficient angle, width, offset,
and discontinuity determination of straight lines by
the discrete Fourier-bilinear transformation algorithm.
IEEE Trans. Image Processing, 6(10):1464–1467.
Matei, B. and Meer, P. (2000). A general method for errors-
in-variables problems in computer vision. In Proc.
IEEE Conf. Computer Vision and Pattern Recognition,
volume 2, pages 18–25.
Nievergelt, Y. (2004). Fitting conics of specific types to
data. Linear Algebra and Appl., 378:1–30.
O’Leary, P. and Zsombor-Murray, P. (2004). Direct and spe-
cific least-square fitting of hyperbolæ and ellipses. J.
Electronic Imaging, 13(3):492–503.
Tipping, M. E. and Bishop, C. M. (1999). Mixtures of prob-
abilistic principal component analysers. Neural Com-
putation, 11(2):443–482.
Venkateswar, V. and Chellappa, R. (1992). Extraction of
straight lines in aerial images.
IEEE Trans. Pattern
Anal. Mach. Intell., 14(11):1111–1114.
Vidal, R. and Ma, Y. (2004). A unified algebraic approach
to 2-D and 3-D motion segmentation. In Proc. Eighth
European Conf. Computer Vision, volume 3021 of
Lecture Notes on Computer Vision, pages 1–15.
Vidal, R., Ma, Y., and Piazzi, J. (2004). A new GPCA al-
gorithm for clustering subspaces by fitting, differenti-
ating and dividing polynomials. In Proc. IEEE Conf.
Computer Vision and Pattern Recognition, volume 1,
pages 510–517.
Vidal, R., Ma, Y., and Sastry, S. (2003). Generalized prin-
cipal component analysis (GPCA). In (CVP, 2003),
pages 621–628.
Vidal, R., Ma, Y., and Sastry, S. (2005). Generalized princi-
pal component analysis (GPCA). IEEE Trans. Pattern
Anal. Mach. Intell., 27(12):1945–1959.
Vidal, R., Ma, Y., Soatto, S., and Sastry, S. (2002).
Segmentation of dynamical scenes from the multi-
body fundamental matrix. In ECCV Workshop
“Vision and Modelling of Dynamical Scenes”.
Available at http://www.robots.ox.ac.uk/
∼
awf/eccv02/vamods02-rvidal.pdf.
Vidal, R., Ma, Y., Soatto, S., and Sastry, S. (2006). Two-
view multibody structure from motion. Int. J. Com-
puter Vision. In press.
VISAPP 2006 - IMAGE ANALYSIS
212