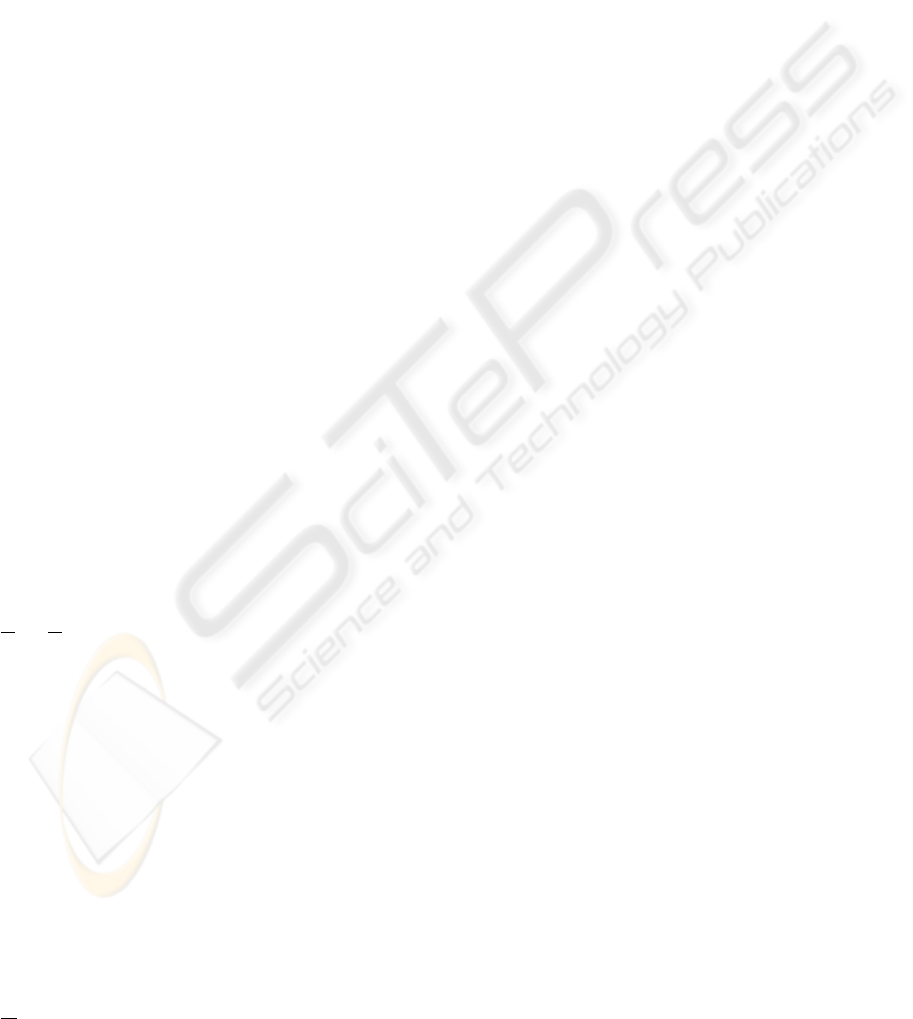
2.1 Construction of the Search Space
The search space S is a set of discrete search positions
with integer coordinates, as the SAD function defines
no finer resolution. A full search defines S as the set
containing every position within a rectangle with size
2sw +1(sw: search window size).
S = {(v
x
,v
y
)|−sw ≤ v
x
,v
y
≤ sw} (3)
Other algorithms like Three Step Search (Wang and
Mersereau, 1999) or 2D Logarithmic Search (Jain and
Jain, 1981) reduce the number of initial search posi-
tions to 9 resp. 5 and add more positions near the min-
imum position in several steps. As, due to their con-
struction, a best match is not guaranteed to be found,
only full search is used in the following.
Solving (1) is done by evaluating the SAD for
every position in S. The SAD calculation can be
aborted early, if the partial SAD already is above the
lowest SAD found so far.
Further speedup is possible by reducing the number
of search positions (early abort of search), the com-
plexity of SAD calculation (using estimates for the
SAD) and optimizing the visiting order of the search
positions. All of these possibilities are used in this
work and described in the following sections.
2.2 Multilevel Successive
Elimination Algorithm
The Multilevel Successive Elimination Algorithm
(MSEA) (Gao, 2003) speeds up SAD calculation.
It defines several lower bounds SAD
l
on the SAD
with decreasing quality and computational demands
to speed up SAD calculation.
Consider P
0
as the set of all pixel positions in
a block. P
l,i
is the level-l quad-tree partition i of
P
0
, it contains all pixels of sub-block i with size
B
2
l
×
B
2
l
. Vectors a and b contain all pixels of the
two blocks to be compared. The evaluation of a new
search position consists of subsequently computing
SAD
L
, SAD
L−1
... SAD
0
, and is aborted as soon
as SAD
l
>SAD
∗
, SAD
∗
being the lowest SAD
found so far.
SAD ≤ SAD
l
=
0≤j≤l
i∈P
l,j
a
i
−
i∈P
l,j
b
i
(4)
The sums over block partitions are precalculated
for every position in the reference image I
t+1
and
every level, taking additional memory of 2(L +1)
times the size of a single input image. Precalculation
time for B=16 and 2 levels equals that of 4.5 SAD
calculations per block. In this case, most positions
with sub-optimal SAD values can be eliminated with
1
16
of the operations a regular SAD would take.
2.3 Prediction and Search Position
Order
It can be seen in section 2.2 that MSEA performes
well if a good search position is known as early as
possible. This fact can be utilized as neighboring
blocks tend to have highly correlated motion vectors
(MVs). In our approach MVs of three adjacent blocks
(left, top and top-right, which have already been com-
puted), three temporally adjacent block MV (same
block, bottom-left and bottom-right in the previous
motion field) and the zero motion MV are evaluated
first. This choice of neighbors is taken from (Tourapis
et al., 2001) and extended by the two diagonal tempo-
ral candidates from (de Haan et al., 1993) to allow
better prediction of upward motion.
The MV having the lowest SAD defines the search
center. As this prediction most of the time leads to the
global minimum SAD being near the search center,
the remaining positions of S are visited in expanding
spiral order starting from the search center. The ef-
fective size of the search window will be larger than
that of a static search center, because the search win-
dow size now limits the maximum detectable accel-
eration instead of the maximum speed. The search is
aborted, if n rings have been completed without find-
ing a lower cost position. n can be adjusted to favor
speed or quality of the algorithm. In the following
experiments n =3is used.
2.4 Modification Towards True
Motion
Optical flow algorithms often incorporate a motion
field smoothness constraint so that local variations in-
crease cost (Brox et al., 2004). Equations determining
MVs usually include image intensities of just a few
pixels directly, which barely is a sound measure of im-
age part similarity, even in strongly textured regions.
Constraining adjacent MVs to be similar adds indi-
rect influence of more pixels, expanding the effective
image area used for similarity measurement, which
lowers noise sensitivity. As a second effect, uniform
image regions adopt MVs of surrounding textured re-
gions.
While block matching directly includes enough
pixels for textured regions, the SAD measure is not
meaningful in poorly textured regions, regions with
non-zero gradient in only one direction or when cal-
culated on periodic image content. In this cases, tak-
ing adjacent MVs into account may improve correct-
ness of determined motion vectors. In our work, we
combine the SAD with an additive term increasing
with MV distance from its surrounding MVs, leading
to a modified cost function (5).
VISAPP 2006 - MOTION, TRACKING AND STEREO VISION
360