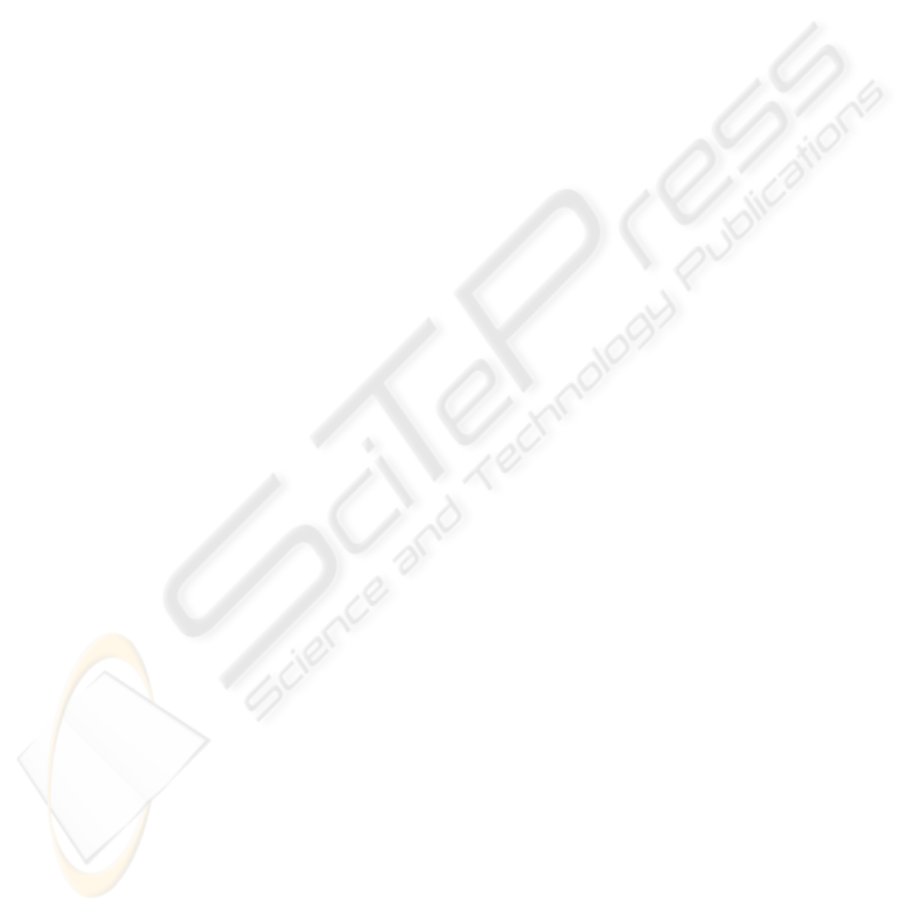
from the production chief because it had a ‘black
head’. The work presented, carried out in laboratory
conditions, with this classification reliability has to
be repeated with a bigger dataset and repeated in the
working conditions in the fish processing plant. The
work shows a feasibility of sorting one type of fish
into different grading classes based on the standards
specified by the fish processing industry. There are
several problems on which one must focus attention
when doing image acquisition of salmon and
labelling them into grading classes:
1. The illumination/backlighting system has
to be carefully set in order to provide easy
thresholding and segmentation of fish
images.
2. Labelling of salmon, for the training
phase, into grading classes has to be
carried out by experts; otherwise one
might end up with fish having, for
instance, a wrong class label without
satisfying any of the parameters defining
that class.
4 CONCLUSION
A computer vision system and algorithm for sorting
Atlantic salmon into two grading classes is
described. This classification algorithm works with
an estimated sorting reliability of 87%. An improved
version of this system can potentially be used to
substitute manual inspectors in the fish processing
line. Further work is required in acquiring a bigger
dataset and expert help on the correct, unmistakable
labelling of grading classes, before building a
prototype.
ACKNOWLEDGEMENTS
The project was funded by the Research Council of
Norway (NFR project No. 145634/140 –‘Efficient
and economic sustainable fish processing industry’).
REFERENCES
Arnarson, H., Bengoetxea, K. and Pau, L.F. 1988. Vision
applications in the fishing and fish product industries.
International Journal of Pattern Recognition and
Artificial Intelligence 2, 657-671.
Brosnan, T., Sun, W. D., 2002. "Inspection and grading of
agricultural and food products by computer vision
systems--a review." Computers and Electronics in
Agriculture 36(2-3), 193-213.
Gunasekaran, S., 2001. In: Nondestructive Food
Evaluation Techniques to Analyze Properties and
Quality.Marcel Dekker, New York.
Gunnlaugsson, G.A. 1997. Vision technology: intelligent
fish processing systems. In: Seafood from producer to
consumer, integrated approach to quality (Eds. J.B.
Luten, T. Børresen and J. Oehlenschläger), Elsevier
Science B.V., 351-359.
HVDUO-5M, HanVision CO, Korea, Retrieved from
http://www.alt-vision.com/documentation/8208-0004-11-
00%20HVDUO-5M%20PD.pdf
Jia, P., Evans, M.D. and Ghate, S.R. 1996. Catfish feature
identification via computer vision. Transactions of the
ASAE 39,
1223-1931.
Theodoridis, S., Koutroumbas, K., 2003. Pattern
Recognition,Academic Press, San Diego, 2
nd
edition..
Pau, L. F., Olafsson, R., 1991. Fish Quality Control by
Computer Vision. New York, Marcel Dekker, 23-38
.
Strachan, N.J.C. and Murray, C.K. 1991. Image analysis in
the fish and food industries. In: Fish quality control by
computer vision (Eds. L.F. Pau and R. Olafsson), M.
Dekker, New York, 209-223.
Strachan, N.J.C. 1993. Recognition of fish species by
colour and shape. Image Vision and Computing 11, 2-
10.
Wagner, H., Schmidt, U. and Rudek, J.H. 1987. Zur
Artenunterscheidung von Seefischen.
Lebensmittelindustrie 34, 20-23.
Walkott, A. P., 1996. Object recognition using colour,
shape and affine invariant ratios. In BMVC96,
Edinburgh, Scotland, 273-282.
Zion, B. Shklyar, A. and Karplus, I. 1999 Sorting fish by
computer vision. Computers and Electronics in
Agriculture 23, 175-187.
VISAPP 2006 - IMAGE ANALYSIS
270