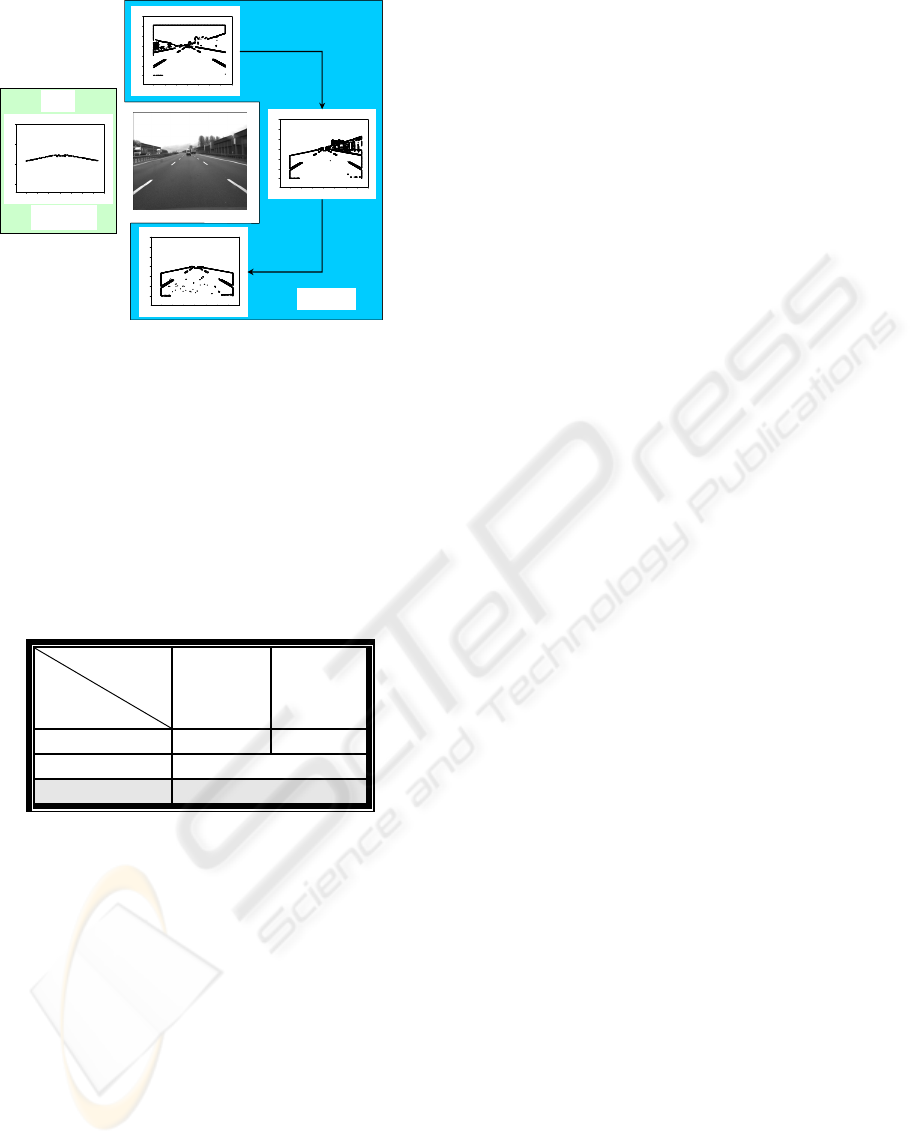
Image Width
0 100 200 300 400 500 600 700
Image Height
0
100
200
300
400
500
600
Image Width
0 100 200 300 400 500 600 700
Image Height
0
100
200
300
400
500
600
Image Width
0 100 200 300 400 500 600 700
Image Height
0
100
200
300
400
500
600
Image Width
0 100 200 300 400 500 600 700
Image Height
0
200
400
600
Auto Adapted to
Environment
Level Set
LDP
①
②
③
Image Width
0 100 200 300 400 500 600 700
Image Height
0
100
200
300
400
500
600
Image Width
0 100 200 300 400 500 600 700
Image Height
0
100
200
300
400
500
600
Image Width
0 100 200 300 400 500 600 700
Image Height
0
100
200
300
400
500
600
Image Width
0 100 200 300 400 500 600 700
Image Height
0
200
400
600
Auto Adapted to
Environment
Level Set
LDP
①
②
③
Figure 3: Comparison results of the segmentation between
Level Set and LDP.
(2), and 0.3 (3). However, the proposed LDP-
based segmentation automatically determines
classification classifier according to the feature
vector of the images at each image frame, and it
gives a key rules to keep same segmentation result in
the variant environment. In order to achieve
quantitative results, 1000 sequence images are
tested. The extension error rates are presented in
Table 2.
Table 2: The comparison of the over/under extension
ratios.
Coef. Of
Kernel
Method
0.7 0.1
Level Set ≅ 69.4 % ≅ 70.6 %
Automatic selection
LDP
8.6 %
In summary of Experiments, the proposed LDP-
based classification is a more powerful method for
the road following application in the classification
cost, the classification ability, and the feature vector
space points of view.
5 CONCLUSION
We proposed the real-time classification method
based on the robust LDP-density discriminator, i.e.,
LDP prior, for the road following application of the
Unmanned Ground Vehicle (UGV). We solved the
pixel classes merging and only road class selection
problem that appeared on the road region when the
number of classes increased, and reduced the
classification cost. In addition we improved the
classification ability by using the probability feature
vector space, i.e., LDP’s feature vector space, from
Gray intensity feature vector space.
REFERENCES
C. Thorpe, T. Kanade, and S.A. Shafer, 1987. Vision and
Navigation for the Carnegie-Mellon Navlab.
Proc.
Image Understand Workshop.
D.A. Pomerleau, 1994. Reliability Estimation for Neural
Network Based Autonomous Driving. Robotics and
Autonomous Systems
.
B. Southall, C. J. Taylor, 2001. Stochastic road shape
estimation. Proceedings of the Eighth International
Conference On Computer Vision (ICCV-01)
.
T.R. Reed, H. Wechsler, 1990. Segmentation of textured
images and Gestalt organization using spatial/spatial-
frequency representations.
IEEE Trans. Pattern
analysis and Machine Intelligent
.
C. Nikias, 1991. High Order Spectral Analysis. Advances
in Spectrum Analysis and Array Processing.
S.Haykin, Ed., Prentice Hall, Englewood Cliffs, NJ
O. Rioul and M. Vetterli, 1991. Wavelet and signal
processing.
IEEE SP mag.
G. Strang, 1989. Wavelet and dilation equation: a brief
introduction. SIAMRev.
L. Wiskott, J.-M. Fellous, N. Kruger, and C. von der
Malsburg, 1997. Face recognition by elastic graph
matching.
IEEE Trans. Pattern Analysis and Machine
Intelligence
.
R.O. Duda, P.E. Hart, D.G. Stork, 2001. : Pattern
classification.
S. Krishnamachari, and R. Chellappa, 1997.
Multiresolution Gauss-Markov Random Field Models
for Texture Segmentation.
IEEE Trans. Image
Processing
.
J. Theiler and G. Gisler, 1997. A contiguity-enhanced K-
Means clustering algorithm for unsupervised
multispectral image segmentation.
Processing SPIE.
P. Jeong, S. Nedevschi, 2003. Intelligent Road Detection
Based on Local Averaging Classifier in Real-Time
Environments.
12
th
IEEE International Conference
on Image Analysis and Processing
.
P. Jeong, S. Nedevschi, 2003. Unsupervised Muliti-
classification for Lane detection using the
combination of Color-Texture and Gray-Texture.
CCCT 2003.
N.K. Paragios, 2000. Geodesic Active Contours and Level
Set methods: Contribution and Applications in
Artificial Vision.
dissertation of doctoral.
VISAPP 2006 - IMAGE ANALYSIS
450