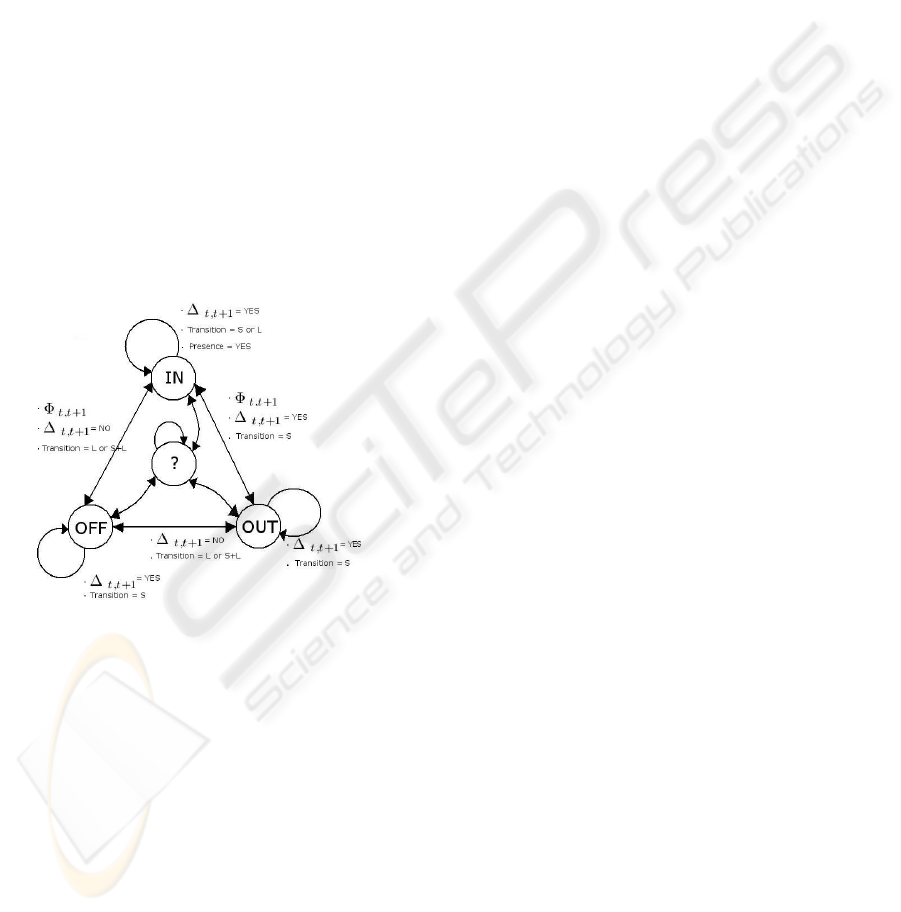
- Φ
t,t+1
: compared activity score between the two
characters having the strongest Lips Activity Rate and
living in the two adjoining segments t and t+1 (sec-
tion 3.2).
- ∆
t,t+1
∈ {yes, no} : stability or instability of
the acoustical environment between the two adjoining
segments t and t+1 (section 4.3).
- Transition ∈ {S,L,S+L} : audio and/or video
boundaries. S for Shot change detection, L for
Speaker change detection and S+L for both change
detection (figure 2 section 4.3).
We chose to create a 4 stated automaton : IN, OUT,
OFF, and a DOUBT state used both as an initial state
and as a temporary escape if the information extracted
from the sequence is not sufficient to classify the in-
tervenant (figure 3). We take in consideration that a
state remains stable on each analyzed segment, and
we define transition of this automaton like possibili-
ties to explore each time a decision has to be taken,
this is to say how the chosen descriptors were evolv-
ing.
Figure 3: Automaton.
As far as there is no corpus where the ground
truth take into account the IN/OUT/OFF classifica-
tion, we have developed our own evaluation con-
tentset of about 21 minutes. Here is a presentation
of results we obtained with our automaton :
- if we consider DOUBT as a correct classification,
we obtain an accuracy rate about 87.1%,
- if we consider DOUBT as a bad classification, we
obtain an accuracy rate about 55.8%,
- if we do not take the doubt into account, this is
to say if we only consider segments that are not clas-
sified as DOUBT cases, we obtain an accuracy rate
about 82.6%.
- the automaton enters into DOUBT state in 24.2%
of the cases,
6 CONCLUSION
We presented videos descriptors that allowed us to
compare visual speech activity between intervenants
from a segment to another, to determinate which char-
acter speaks inside a same segment, and finally to
avoid the Viola and Jones’s face detector deficiencies
if it is used into a face following way.
We also showed that MFCC variations considered
at the frontiers of the transitions between classes, rep-
resents a reliable descriptor to characterize change or
stability between two acoustical environments.
Finally, these information joined in an automaton
allowed us to create a reliable audiovisual descriptor
to get an original IN, OUT and OFF classification for
an intervenant.
REFERENCES
Furui, S. (1981). Cepstral analysis technique for automatic
speaker verification. In IEEE Trans. Acoust. Speech
Signal Process., volume 29, pages 254–272.
Jaffre, G. and Joly, P. (2004). Costume: A new feature
for automatic video content indexing. In RIAO 2004,
pages 314–325, Avignon, France.
Kijak, E. (2003). Structuration multimodale des videos de
sports par modeles stochastiques. PhD thesis, Univer-
site de Rennes 1.
Kraaij, W., Smeaton, A., Over, P., and Arlandis, J. (2004).
Trecvid 2004 - an introduction. In Proceedings of the
TRECVID 2004 Workshop, pages 1–13, Gaithersburg,
Maryland, USA.
Mokbel, C., Jouvet, D., and J., M. (1995). Blind equal-
ization using adaptitive filtering for improving speech
recognition over telephone. In European Conference
on Speech Communication and Technology, pages
817–820, Madrid, Spain.
Potamianos, G., Graf, H., and Cosatto, E. (1998). An image
transform approch for hmm based automatic lipread-
ing. In Proceedings of the Internationnal Confer-
ence on Image Processing, volume 3, pages 173–177,
Chicago.
Potamianos, G., Neti, C., Luettin, J., and Matthews, I.
(2004). Audio-visual automatic speech recognition:
An overview. In Bailly, G., Vatikiotis-Bateson, E., and
Perrier, P., editors, Issues in Visual and Audio-Visual
Speech Processing. MIT Press.
Tianhao, L., Q.-J. F. (2006). Analyze perceptual adaptation
to spectrally-shifted vowels with gmm technique. In
10th Annual Fred S. Grodins Graduate Research Sym-
posium, pages 120–121. USC School of Engineering.
SIGMAP 2006 - INTERNATIONAL CONFERENCE ON SIGNAL PROCESSING AND MULTIMEDIA
APPLICATIONS
188