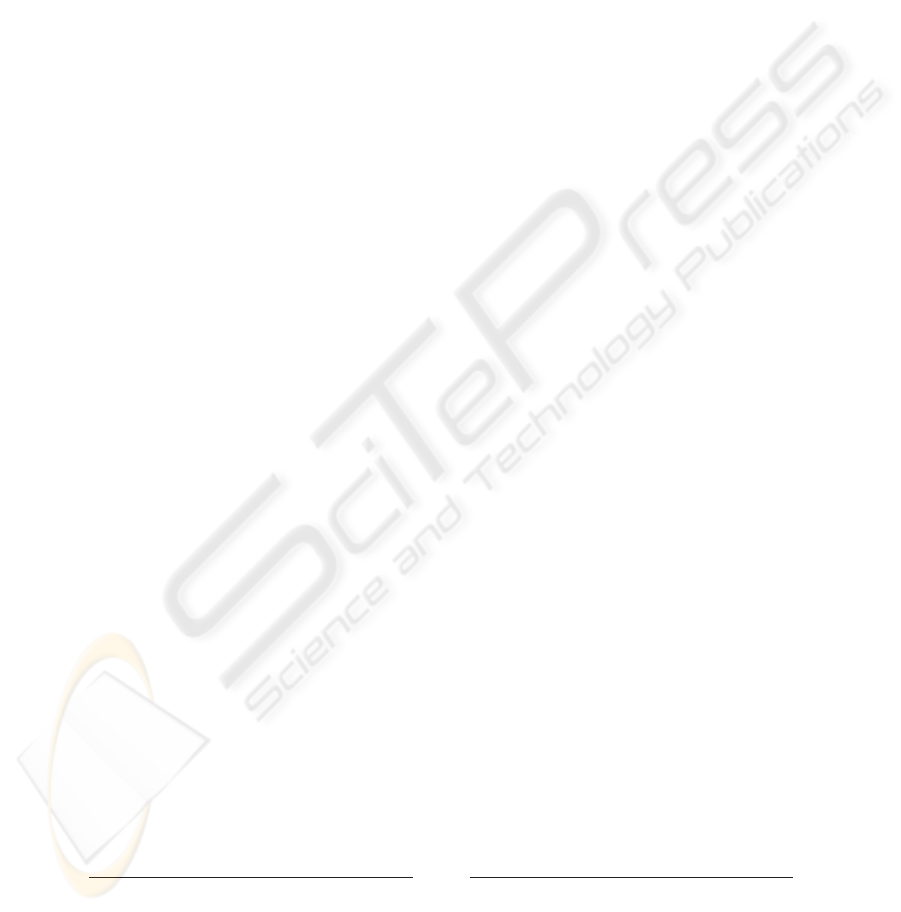
is also commonly found the lowest classification test error rate, as it occurs in our case.
As the number of components keeps increasing, the well-known overtraining effect
appears, the log-likelihood in test falls and the accuracy degrades. For this reason we
decided to limit the number of mixture components to 100, since additional trials with
an increasing number of mixture components confirmed this performance degradation.
Figure 2 shows competing curves for test error-rate as a function of the number of
mixture components for the English-based, bilingual bag-of-words-based, global and
local classifiers; there are two plots, one for Traveller and the other for BAF. Error bars
representing 95% confidence intervals are plotted for the English-based classifiers in
both plots, and the global classifier in BAF.
From the results for Traveller in Figure 2, we can see that there is no significant
statistical difference in terms of error rate between the best monolingual classifier and
the bilingual classifiers. The reason behind these similar results can be better explained
in the light of the statistics of the Traveller dataset shown in Table 1. The simplicity of
the Traveller dataset, characterised by its small vocabulary size and its large number of
running words, allows for a reliable estimation of model parameters in both languages.
This is reflected in the high accuracy (∼ 95%) of the monolingual classifiers and the
little contribution of a second language to boost the performance of bilingual classifiers.
Nevertheless, bilingual classifiers seem to achieve systematically better results.
In contrast to the results obtained for Traveller, the results for BAF in Figure 2 in-
dicate that bilingual classifiers perform significantly better than monolingual models.
More precisely, if we compare the curves for the English-based classifier and the global
classifier, we can observe that there is no overlapping between their error-rate confi-
dence intervals. Clearly, the complexity and data scarcity problem of the BAF corpus
lead to poorly estimated models, favouring bilingual classifiers that take advantage of
both languages. However, the different bilingual classifiers have similar performance.
Additional experiments using smooth n-gram language models were performed
with the well-known and publicly available SRILM toolkit [17]. A Witten-Bell [18]
smoothed n-gram language model was trained for each supervised class separately and
for both languages independently. These class-dependent language models were used to
define monolingual and bilingual Naive Bayes classifiers. Results are given in Table 2.
From the results in Table 2, we can see that 1-gram language models are similar
to our 1-component mixture models. In fact, both models are equivalent except for the
parameter smoothing. The results obtained with n-gram classifiers with n > 1 are much
better that the results for n = 1 and slightly better than the best results obtained with
general I-component multinomial mixtures. More precisely, the best results achieved
with n-grams are 1.1% in Traveller and 2.6% in BAF, while the best results obtained
with multinomial mixtures are 1.4% in Traveller and 2.9% in BAF.
Table 2. Test-set error rates for monolingual and bilingual naive classifiers based on smooth
n-gram language models in Traveller and BAF.
Traveller 1-gram 2-gram 3-gram
English classifier 4.1 1.9 1.3
Spanish classifier 2.8 1.2 1.2
Bilingual classifier 3.3 1.2 1.1
BAF 1-gram 2-gram 3-gram
English classifier 5.3 3.5 3.6
French classifier 6.7 4.4 4.4
Bilingual classifier 4.1 2.8 2.6
100