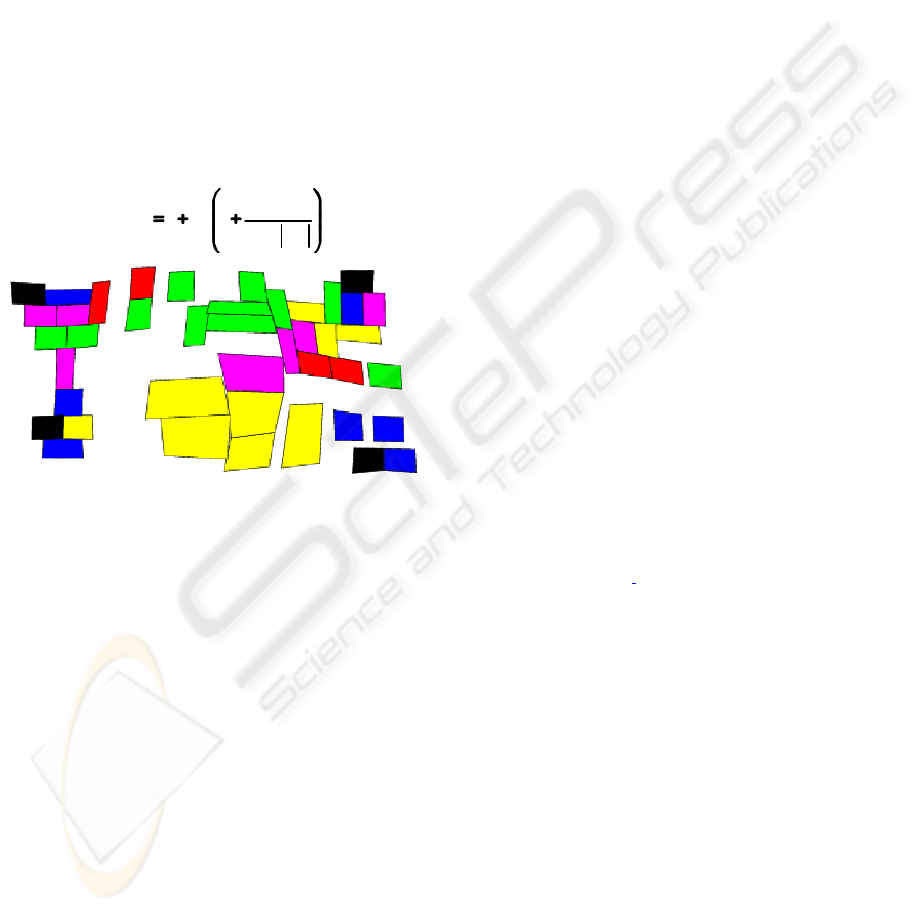
Newman (Gastner & Newman, 2004) who propose a
new method for constructing cartograms which is
simpler than many other methods, and therefore the
quickest to compute. They illustrated the method
with applications on the results of the 2000 U.S.
Presidential election, lung cancer cases in the State
of New York, and the geographical distribution of
stories appearing in the news. Gastner, Shalizi and
Newman (Gastner, Shalizi & Newman, 2004)
applied the same method to maps and cartograms of
the 2004 US presidential election results.
The last challenge was to find a representative
non negative distribution from internal indicators of
our agents. We called pseudoDensity the distribution
that we compute from the values of the
pseudoPosition. As this internal indicator could have
negative or positive values, we use the following
formulae to transform this indicator to a strictly
positive value:
PP
PP
LnitypseudoDens
max
11
Figure 11: Cartogram of Step 118 of the Game of Risk.
We use morphing between two successive
cartograms to alert the user that the current view will
be updated. Figure 11 shows the new shape of a
cartogram computed with pseudoDensity.
5 CONCLUSION
In this paper we describe a system designed to help
deciders interpret information of a current situation.
The system can represent information with its
dynamic evolution. The core of the system is made
of three MASs, and we have focused here on the
first layer, because it has to represent, and to store
information. The initial goal of the system was to
help deciders prevent crises by analysing the
information they have. We think that the main part
of the system is generic and can be re-used for
different applications. This is why we are testing our
system on various types of applications (prediction
crisis, game of Risk, E-learning, representation of
information). The heart and soul of the system is,
with an original representation of information and a
particular treatment of it, to be able to prevent or/and
predict (depending on the kind of application)
something will (or is) happen(ing). Representation
of information is done in the first layer we
described, by the factual agents which contain the
composite semantic features constituting the atomic
data elements of information. Some graphic tools we
use for helping the decider (but also debugging in
fact), are described in this paper. These tools help us
understand the parameters of the factual agents
which are the most accurate to characterise
information and what are the essential data to
transfer to the second layer of the global system.
We are currently working on some
complementary directions:
– developing new tools for a deeper analysis of the
MASs;
– generating a full set of scenarios for the game of
Risk. The game of Risk is an example we use to
adjust the generic aspects of the core. Other
applications will prove the genericity of the
architecture;
– connecting the representation MAS to the
characterisation MAS which is our immediate
objective.
REFERENCES
Andrieu D., 2005, L'intérêt de l'usage des cartogrammes :
l'exemple de la cartographie de l'élection présidentielle
française de 2002, M@ppemonde 77 (2005.1)
retrieved May 20 2005, from
http://mappemonde.mgm.fr/num5/articles/art05105.html
Barber K. S., Graser T., Col. Silva J. & Holt, J., 1999.
Reference Architecture Representation Environment
(RARE) Guiding Class Derivation and Managing
Tradeoffs during Object-Oriented Analysis. Technical
Report TR99-UT-LIPS-SEPA-03. University of Texas,
Laboratory for Intelligent Processes and Systems.
Bertin J.-C. & Gravé P., 2004. Didactic ergonomics and
Web-based materials design. CALICO 2004. Carnagie
Mellon University, USA.
Boukachour H., Coletta M., Galinho T., Person P., Serin
F. & Simon G., 2001. Preventive Vigil Multiagent
System. SCI2001. Orlando, USA.
Boukachour H., 2002. Système de veille préventive pour
la gestion de situations d’urgence : une modélisation
par organisations d’agents. Application aux risques
industriels. PhD Thesis, University of Le Havre,
France.
Boukachour H., Simon G., Coletta M., Galinho T., Person
P. & Serin F., 2002. Preventive Monitoring
Information System: a Model Using Agent
Organizations, SCI2002. Orlando, USA.
ICEIS 2006 - ARTIFICIAL INTELLIGENCE AND DECISION SUPPORT SYSTEMS
162