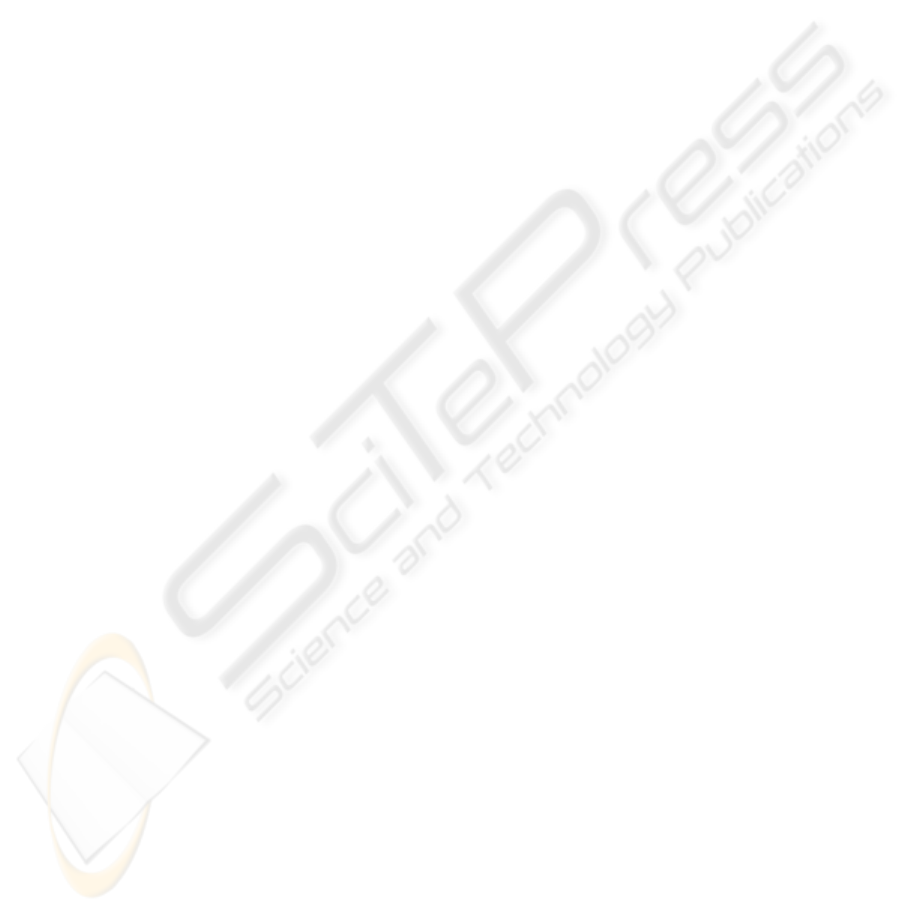
higher level of risk. On this basis, the best classifier
in Table5 is the Support Vector Machine (SVM)
trained using 50% split.
4 CONCLUSIONS AND FURTHER
WORKS
POSSUM and PPOSSUM are generic clinical tools
that allow a metric factor to be used in assessing the
severity of illness. The risk assessments are
compared to reported mortality across a group of
patients. The ratio between the predictions of
POSSUM, PPOSSUM and the observed mortality
shows the performances of the system. However,
each individual patient has an assessment of risk,
which is based on clinical judgement. The value of
the scoring system quantifies the risks of patient, and
these risks can be compared to the reported ones
(Jones & Cossart, 1999).
POSSUM and PPOSSUM seem to over predict
mortality for the data. These models are restricted to
predictions of mortality, morbidity and death rates.
For cardio vascular disease the combination of other
outcomes such as 30 day MR or stroke or dead may
give rise to more appropriate measures of risk.
By using a confusion matrix, the
misclassification of each model is evaluated. From
table 3 and table 4 it seems that using different
models of neural network produces smaller
misclassification errors than with POSSUM, and
PPOSSUM. More interestingly, the models using the
new outcome of risk (High, Medium, Low) had the
smallest percentage of misclassification compared to
the other risk predication models (i.e. mortality or
morbidity). The bias of misclassification for each
neural network models needs to be subjected to
further investigation. More over, a comparison of
supervised versus unsupervised classifiers may help
in determining more appropriate patient
classifications. These results can then be applied in
determining what of the original data should be used
to generate a better set of classifiers and indicators
of use in predicting cardio vascular risk.
The selection of input attributes for patient
classification is an issue for this and further work.
The set of attributes, and their value ranges, can be
made small enough they will reduce the
complication of developing classifiers for the
domain. The domain independent attribute and data
reduction techniques will be developed from the
theory of mutual information (Cover & Thomas,
1991). If the domain derived techniques are not to be
trusted or are to be independently validated, then
alternative means of clustering patients (according to
risk) are required. We will use unsupervised neural
techniques of various types to achieve this.
ACKNOWLEDGEMENTS
Thank you to the Clinical Biosciences Institute,
University of Hull and the Institute for Systems and
Technologies of Information, Control and
Communication for support funding.
REFERENCES
Copeland G P, Jones D, Walters M. (1991). POSSUM: a
scoring system for surgical audit. British Journal
Surgery, 78, 355-360.
Copeland G P. (2002). The POSSUM system of surgical
audit. Archives of Surgery, 137, 15-19.
Cover, T. M., Thomas, J. A. (1991). Elements of
Information Theory. New York, Wiley.
Dunham, M. H. (2002). Data Mining Introductory and
Advance Topics. Upper Saddle River, NJ, Prentice
Hall/Pearson Education.
Jones H.J.S, & Cossart de L., (1999) Risk scoring in
surgical patients. British Journal Surgery, 86, 149-
157.
Siganos, D. (1996). Neural Networks in Medicine.
Retrieved January 9, 2006 from:
http://www.doc.ic.ac.uk/~nd/surprise_96/journal/vol2/
ds12/article2.html
Turton E. P., Scott D. J., Delbridge M., Snowden S., &
Kester R. C. (2000). Ruptured abdominal aortic
aneurysm: a novel method of outcome prediction
using neural network technology. European Journal of
Vascular and Endovascular Surgery 19(2), 184-9.
WEKA software (University of Waikato, New Zealand,
version 3.4.5). (n.d.). Retrieved January 9, 2006 from
http://www.cs.waikato.ac.nz/~ml/weka/index.html
Witten, I.H., & Eibe F. (1999). Data Mining: Practical
Machine Learning Tools and Techniques with Java
Implementations. Morgan Kaufmann.
Yii M. K., and Ng K. J., (2002), Risk-adjusted surgical
audit with the POSSUM scoring system in a
developing country, British Journal of Surgery,
89:110-113.
ICEIS 2006 - ARTIFICIAL INTELLIGENCE AND DECISION SUPPORT SYSTEMS
234