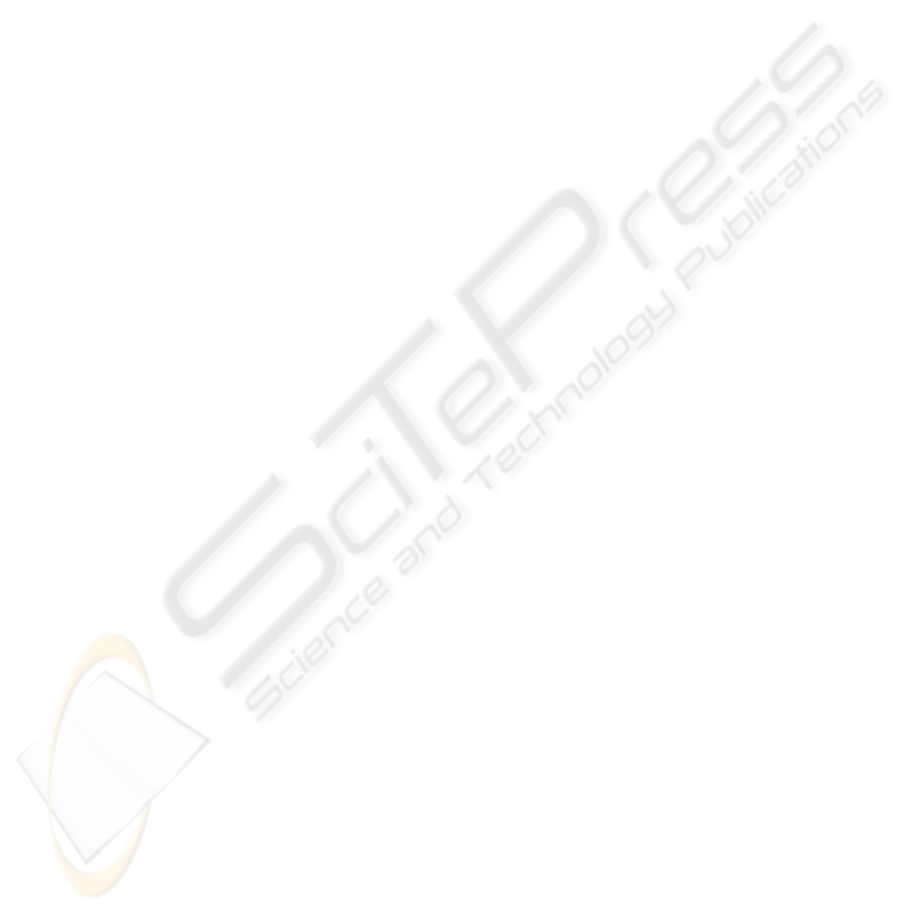
also found. As stated in [5], we also found that ICA2 gives best result when combined
with COS. We also agree with Navarrete et al. [15] that LDA+COS works better than
PCA. We agree with Moghaddam et al. [12] and with Yang et al. [8] who stated that
there is no significant difference between PCA and ICA. We also confirm the result
in [8] that there is no significant performance difference between ICA and preprocess-
ing whitening PCA step.
5 Conclusion
This paper presented an independent, comparative study of three most popular appear-
ance based face recognition projection methods (PCA, LDA and LDA) and their ac-
companied three distance metrics (City block, Euclidean and Cosine) in equal working
conditions. This experimental setup yielded 12 different algorithms to be compared.
From our independent comparative research we can derive that the L2 metric is the
most promising combination for all tasks. Although ICA1+L1 seems to be promising,
except for the illumination changes task where LDA+COS and ICA2+COS outperforms
PCA and ICA1. For all probe sets the COS seems to be the best choice of metric for
LDA and ICA2 and L1 for PCA and ICA1. LDA+COS combination turned out to be the
best choice for temporal changes task. In spite of the fact that L2 metric produced lower
results it is surprising that it was used so often in the past. We also tested only whitened
PCA preprocessing step of ICA method and it confirms that there is no performance
difference between ICA and preprocessing PCA.
References
1. Zhao, W., Chellappa, R., Phillips, P.J., Rosenfeld, A.: Face Recognition: A Literature Survey,
ACM Computing Surveys, (2003) 399–458
2. Solina, F.,Peer, P., Batagelj, B., Juvan, S., Kova
ˇ
c, J.: Color-Based Face Detection in the ”15
Seconds of Fame” Art Installation, International Conference on Computer Vision / Computer
Graphics Collaboration for Model-based Imaging, Rendering, image Analysis and Graphical
special Effects MIRAGE’03, (2003) 38–47
3. Turk, M., Pentland, A.: Eigenfaces for Recognition, Journal of Cognitive Neurosicence, 3(1),
1991, 71–86
4. Zhao, W., Chellappa, R., Krishnaswamy, A.: Discriminant Analysis of Principal Components
for Face Recognition, Proc. of the 3rd IEEE International Conference on Face and Gesture
Recognition, FG’98, (1998) 336
5. Bartlett, M.S., Movellan, J.R., Sejnowski, T.J.: Face Recognition by Independent Component
Analysis, IEEE Trans. on Neural Networks, 13(6), (2002) 1450–1464
6. Draper, B., Baek, K., Bartlett, M.S., Beveridge, J.R.: Recognizing Faces with PCA and ICA,
Computer Vision and Image Understanding (Spacial Issue on Face Recognition), 91(1-2),
(2003) 115–137
7. Hyv
¨
arinen, A., Oja, E.: Independent component analysis: algorithms and aplications. Neural
Networks. 13(4-5) (2000) 411–430
8. Yang, J., Zhang, D., Yang, J.Y.: Is ICA Significantly Better than PCA for Face Recognition?
ICCV. (2005) 198–203
79