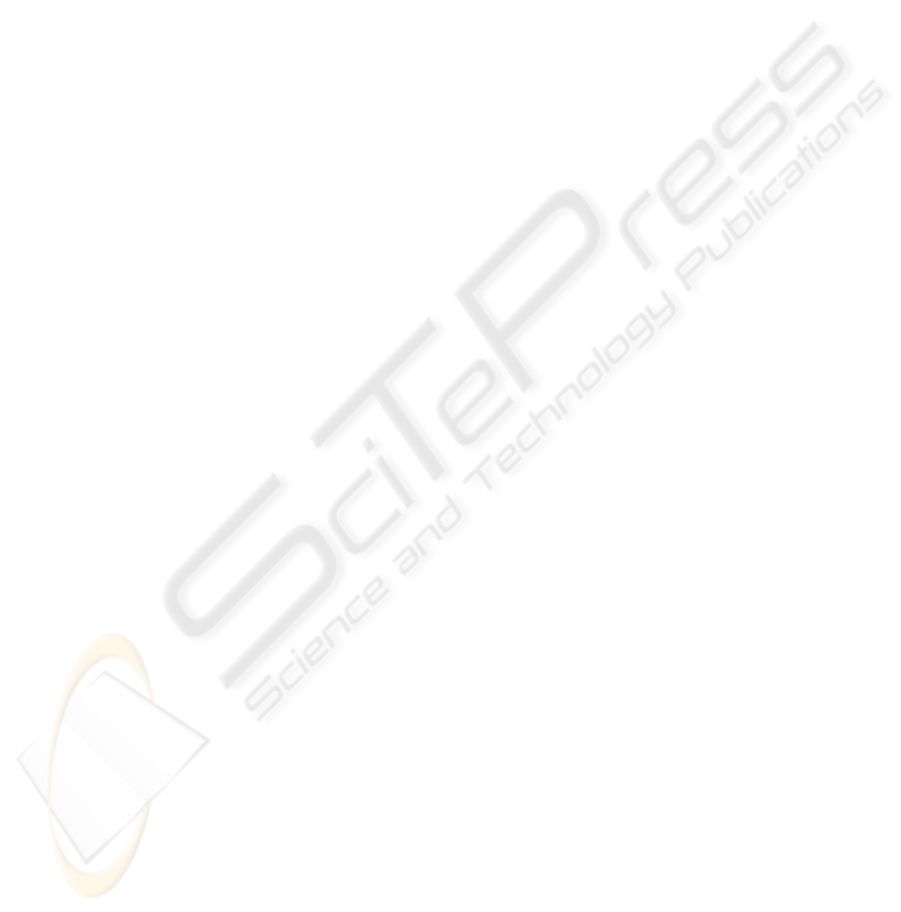
handled and then refined through interest association
analysis in order to remove false or redundant
interests. To each identified interest topic, users who
have this topic are clustered together, so a series of
multi-interest communities are obtained. Because
members of a community may have interest in the
topics of other communities, the formed
communities are also connected with each other,
resulting in a kind of community network indicating
interest associations of groups of users. Experiments
on the collected EachMovie data showed that the
formed multi-interest communities were more
cohesive and condensed when users were clustered
based on their refined interest topics.
Intra-community and inter-community
recommendations can be made based on formed
multi-interest communities. The former recommends
to a user popular resources deemed by other
community members within a community, whereas
the latter suggests resources of other categories but
most interesting to the other members of a
community. Consequently a user will receive
information within and beyond his identified
interests. From his responses to the
recommendations, e.g., accept or reject some of
them, the user’s real interests will be further
identified and even new interests of the user may be
discovered.
The recommendation approach presented in this
paper is more suitable to users that welcome all
information recommendations. Some users in reality
may only wish to receive carefully selected
information, which means their recommendation
requirements
1)(Re
uq
. How to tailor
recommendations according a user’s requirements
and preferences will be studied as our future work.
In addition, a user’s interest to a certain topic or
resource will be divided into positive and negative,
instead of all positive interests as shown in this
paper. Because the users of the EachMovie data are
anonymous, it is difficult to judge whether they
would like the groups allocated to them and the
recommendations suggested by our approach. The
proposed multi-interest communities and
community-based recommendation will be further
tested on real users so that the users’ feedback will
be used to examine and improve the approach
presented in this paper.
ACKNOWLEDGEMENTS
The author is grateful to the support from BT Long
Term Research Venturing, colleagues in Nanjing
University of China and the National Natural
Science Foundation of China Grant No.60402027.
RERERENCES
Cassiopeia http://www.cassiopeia.com/
EachMovie http://research.compaq.com/SRC/eachmovie/
e-groups http://www.egroups.com/
Han, J. and Kamber, M., 2000. Data Mining: Concepts
and Techniques, Morgan Kaufmann Publishers.
Iamnitchi, A., Ripeanu, M. and Foster, I., 2004. Small-
World File-Sharing Communities, The 23
rd
Conference of the IEEE Communications Society.
Hong Kong.
Khambatti, M., Ryu, K.D. and Dasgupta, P., 2003,
Structuring Peer-to-Peer Networks using Interest-
Based Communities. 1st International Workshop on
Databases, Information Systems, and Peer-to-Peer
Computing. Berlin, Germany, pp. 48-63.
Lawrence, R.D., Almasi, G.S., Kotlyar, V., et. al. 2001.
Personalization of Supermarket Product
Recommendations. Data Mining and Knowledge
Discovery 5(1-2):11-32.
Nijholt, A., 2002. Computer-facilitated community
building for E-learning, Proc. IEEE Inter. Conf. on
Advanced Learning Technologies. Kazan, Russia,
pp.541-543.
Ogston, E., Overeinder, B., van Steen, M., and Brazier, B.,
2003. Group Formation Among Peer-to-Peer Agents:
Learning Group Characteristics. Inter. Workshop on
Agents and Peer-to-Peer Computing, pp. 59-70.
Seufert, S., Lechner, U. and Stanoevska, K., 2002. A
reference model for online learning communities.
Inter. J. on E-Learning, Jan-Mar, pp.43-55.
Steinbach, M., Karypis, G. and Kumar, V., 2000. A
comparison of document clustering techniques, KDD
Workshop on Text Mining.
Talavera, L. and Gaudioso, E., 2004, Mining student data
to characterize similar behaviour groups in
unstructured collaboration spaces. Workshop on
Artificial Intelligence in CSCL, 16
th
European
Conference on Artificial Intelligence. pp.17-23.
Vignette http://vignette.com/
Webfair http://www.webfair.com/
Wang, F., 2002. Self-organising communities formed by
middle agents. Proc. of the 1st Inter. Conference on
Autonomous Agents and Multi-Agent Systems,
Bologna, Italy, pp 1333-1339.
Yang, F., Shen, R. and Han, P., 2004. A novel self-
organizing e-learner community model with award
and exchange mechanisms, Journal of Zhejiang
University Science, 5(11): 1343-1351.
WEBIST 2007 - International Conference on Web Information Systems and Technologies
44