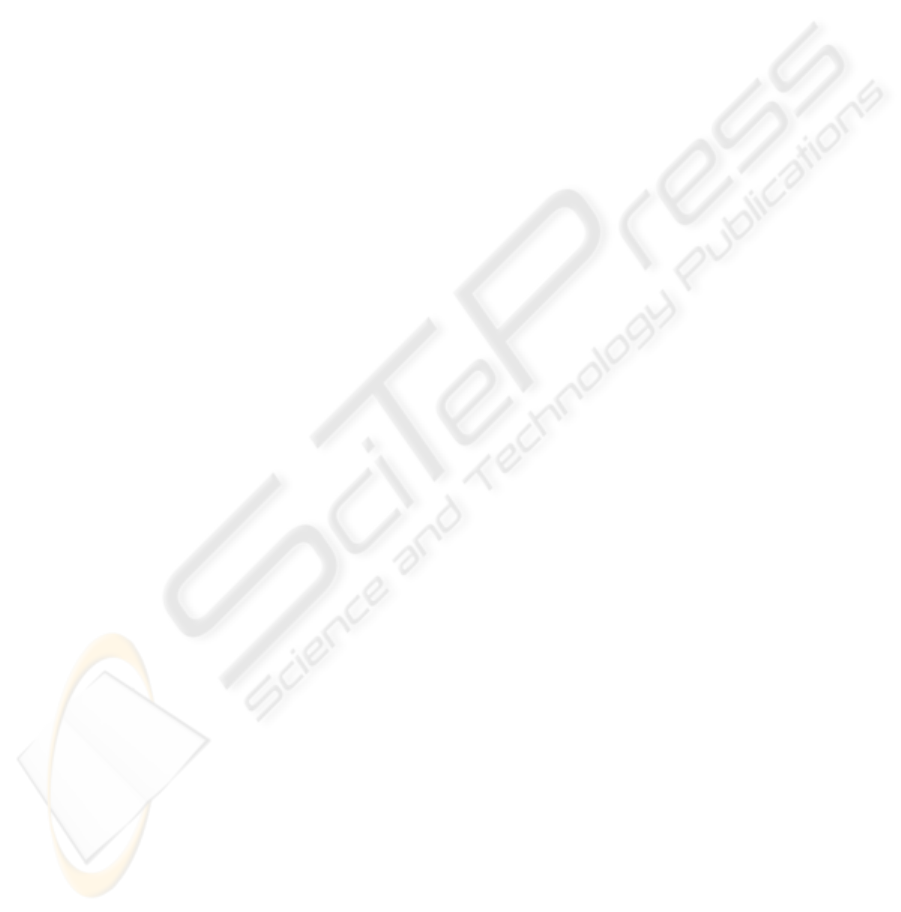
hierarchical models. Future research must attempt to
find means to estimate higher-order structures.
ACKNOWLEDGEMENTS
The work described in this paper was substantially
supported by a grant from the Research Grants
Council of the Hong Kong Special Administrative
Region, China [Project No. CityU 1361/04H].
REFERENCES
Bhattacherjee, A., 2001. An empirical analysis of the
antecedents of electronic commerce service
continuance, Decision Support Systems, 32, 201-214.
Bollen, K. A., 1989. Structural equations with latent
variables, Wiley.
Chin, W. W., 1998. Issues and opinion on structural
equation modeling, MIS Quarterly, 22, 7-16.
Chin, W. W. and Lee, M. K. O., 2000. In Twenty-First
International Conference on Information Systems
(Eds, Orlikowski, W. J., Ang, S., Weill, P., Krcmar, H.
and DeGross, J. I.) Brisbane, Australia, pp. 553-563.
DeLone, W. H. and McLean, E. R., 1992. Information
systems success: The quest for the dependent variable,
Information Systems Research, 3, 60-95.
DeLone, W. H. and McLean, E. R., 2003. The DeLone
and McLean model of information systems success: A
ten-year update, Journal of Management Information
Systems, 19, 9-30.
Doll, W. J. and Torkzadeh, G., 1988. The measurement of
end-user computing satisfaction, MIS Quarterly, 12,
259-274.
Doll, W. J., Xia, W. and Torkzadeh, G., 1994. A
confirmatory factor analysis of the end-user
computing satisfaction instrument, MIS Quarterly, 18,
453-461.
Fornell, C. and Larcker, D., 1987. Evaluating structural
equation models with unobservable variables and
measurement error, Journal of Marketing Research,
18, 39-50.
Gelderman, M., 1998. The relation between user
satisfaction, usage of information systems and
performance, Information and Management, 34, 11-
18.
Hair , J. F., Anderson, R. E., Tatham, R. L., 1992.
Multivariate Data Analysis: With Readings, 3
rd
ed.,
New York, MacMillan Publishing Co.
Hair, J. F., Anderson, R. E., Tatham, R. L. and Black, W.
C., 1998. Multivariate data analysis,5
th
Ed.,
Englewood Cliffs, NJ: Prentice-Hall.
Harlow, L. L. and Newcomb, M. D., 1990. Towards a
general hierarchical model of meaning and satisfaction
in life, Multivariate Behavioral Research, 25, 387-
405.
Isakowitz, T., Bieber, M. and Vitali, F., 1998. Web
Information Systems, Communications of the ACM,
41, 78-80.
Joreskog, K. G. and Sorbom, D., 1996. LISREL 8: User's
Reference Guide, Scientific Software International.
Kaschek, R., Schewe, K.-D., Wallace, C. and Matthews,
C., 2004. In Web Information Systems (Eds, Taniar, D.
and Rahayu, J. W.) IDEA Group Publishing, Hershey,
PA.
Marsh, H. W. and Hocevar, D., 1985. Application of
confirmatory factor analysis to the study of self-
concept: First- and higher order factor models and
their invariance across groups, Psychological Bulletin,
97, 562-582.
McHaney, R., Hightower, R. and Pearson, J., 2002. A
validation of the end-user computing satisfaction
instrument in Taiwan, Information and Management,
39, 503-511.
McKinney, V., Yoon, K. and Zahedi, F. M., 2002. The
measurement of web-customer satisfaction: An
expectation and disconfirmation approach,
Information Systems Research, 13, 296-315.
Rai, A., Lang, S. S. and Welker, R. B., 2002. Assessing
the validity of IS success models: An empirical test
and theoretical analysis, Information Systems
Research, 13, 50-69.
Rindskopf, D. and Rose, T., 1988. Some theory and
applications of confirmatory second-order factor
analysis, Multivariate Behavioral Research, 23, 51-67.
Zviran, M. and Erlich, Z., 2003. Measuring IS user
satisfaction: Review and Implications,
Communications of the Association for Information
Systems, 12, 81-103.
WEB-BASED INFORMATION SYSTEMS SATISFACTION - Theoretical Development and Testing of Competing
Models
53