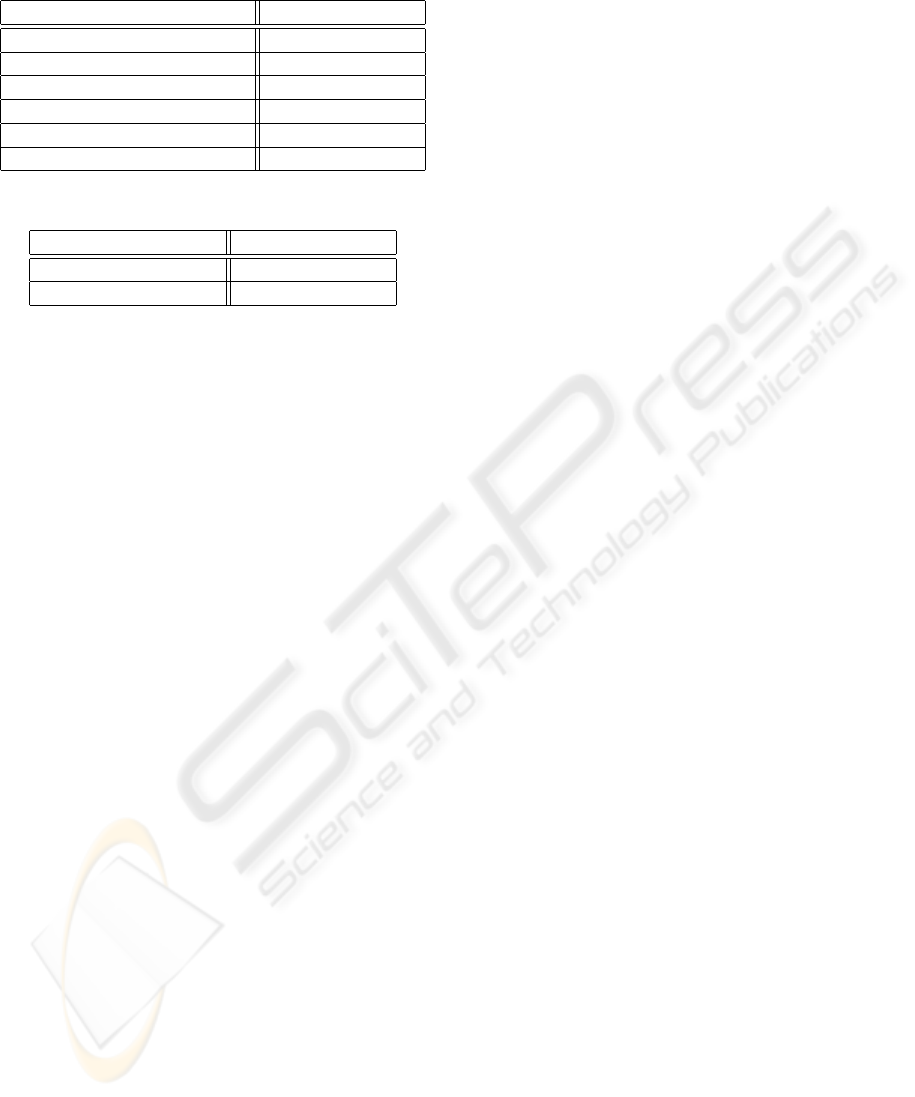
Table 1: Detection Results (Scenario 1).
Method Detection rate(%)
Proposed Method 60.8%(104/171)
(M.Mahoney, 2003) 71.4%(132/185)
(Tyson et al., 2000) 55.6%(15/27)
(Neumann and Porras, 1999) 50.3%(85 / 169)
(Vigna et al., 2000) 46.8%(81 / 173)
(Barbara et al., 2001) 40.2%(41 / 102)
Table 2: Detection Results (Scenario 2).
Method Detection rate(%)
The proposed System 58.5%(100/171)
NETAD 37.8%(70/185)
the attacks are not used for learning. In both scenar-
ios, we normalize all elements of feature sets to zero
mean and unit variance.
The parameters setup of the experiment are fol-
lows: # of permissible false alarms is 10/day(R. and
et al, 2000), time-slot interval is 60 seconds, time out
(T
u
) of UDP flow is 600 seconds and validity time
(T
f
) is 600 seconds.
The total number of false alarms permitted in two
weeks(10 days) is 100 (10 per day). The threshold of
projection distance for each day for each module is
determined by preliminary experiment.
4.2 Detection Performance
The detection results of the proposed system and con-
ventional systems are shown in Table 1. The pro-
posed system has better results for both the number
of attacks detected and the detection rate compared
to other methods, except for NETAD (M.Mahoney,
2003). The total number of each method is different
because each method observes different objects.
The detection results of the proposed system and
NETAD for scenario 2 are shown in Table 2. Table
2 shows that the detection number and the detection
rate of NETAD, which shows best performance when
attack-free data is used for learning, have greatly de-
creased. On the other hand, the detection result of
the proposed system hardly deteriorates and the detec-
tion number and detection rate are higher than those
of NETAD. Most of the anomaly-based IDS require
attack-free data for learning. But in practice, such
attack-free data are very hard to get and it is thought
that the learning data that such IDSs learn have at least
a few attacks. The results of scenario 2 are close to
those of a real network. Therefore, the detection abil-
ity of the proposed system does not deteriorate much
even under an environment close to that of a real net-
work, scenario 2. This proves that our proposed sys-
tem has high accuracy and robustness.
5 CONCLUSION
In this paper, we have proposed a anomaly detec-
tion system using three different feature sets which
are extracted based on the reclassification of attacks.
Our proposed method effectively detects wide range
of attacks by independently treating the feature sets,
and suppresses the negative effect of attack traffic in-
cluded in learning data by using a statistical method
in learning phase.
We have demonstrated that our proposed system
can achieve high detection rate and high robustness
by experiments using the data set in (DARPA, 1999).
REFERENCES
Barbara, D., Jajodia, S., Wu, N., and Speegle, B. (2001).
Adam: Detecting intrusions by data mining.
Brownlee, N. (1998). Network management and realtime
trafficflow measurement. Journal of Network and Sys-
tems Management, 6(2):223–227.
DARPA (1999). Mit lincoln laboratory - darpa intrusion de-
tection evaluation. http://www.ll.mit.edu/
IST/ideval/.
Debra, A., F.Lunt, T., Tamaru, H. J. A., and Valdes, A.
(1995). Detecting unusual program behavior using the
statistical component of the nextgeneration intrusion
detection expert system(nides). Technical report.
Mahoney, M. V. and Chan, P. K. (2001). Detecting novel at-
tacks by identifying anomalousnetwork packet head-
ers. Technical report.
M.Mahoney (2003). Network traffic anomaly detection
based on packet bytes. In ACM-SAC, pages 346–350.
Neumann, P. and Porras, P. (1999). Experience with emer-
ald to date. In Proceedings of First USENIX Work-
shop on Intrusion Detection and Network Monitoring,
pages 73–80.
OIKAWA, T., WAIZUMI, Y., OHTA, K., KATO, N., and
NEMOTO, Y. (2002). Network anomaly detection us-
ing statistical clustering method. Technical report.
R., L. and et al (2000). The 1999 darpa off-line intrusion
detection evaluation. 34:579–595.
SPADE. http://www.silicondefense.com/
software/spice/.
Tyson, M., Berry, P., Williams, N., Moran, D., and Blei, D.
(2000). Derbi: Diagnosis, explanation and recovery
from computer break-ins. Technical report.
Vigna, G., Eckmann, S., and Kemmerer, R. (2000). The
stat tool suite. In Proceedings of the 2000 DARPA
Information Survivability Conference and Exposition
(DISCEX).
A NETWORK-BASED ANOMALY DETECTION SYSTEM USING MULTIPLE NETWORK FEATURES
413