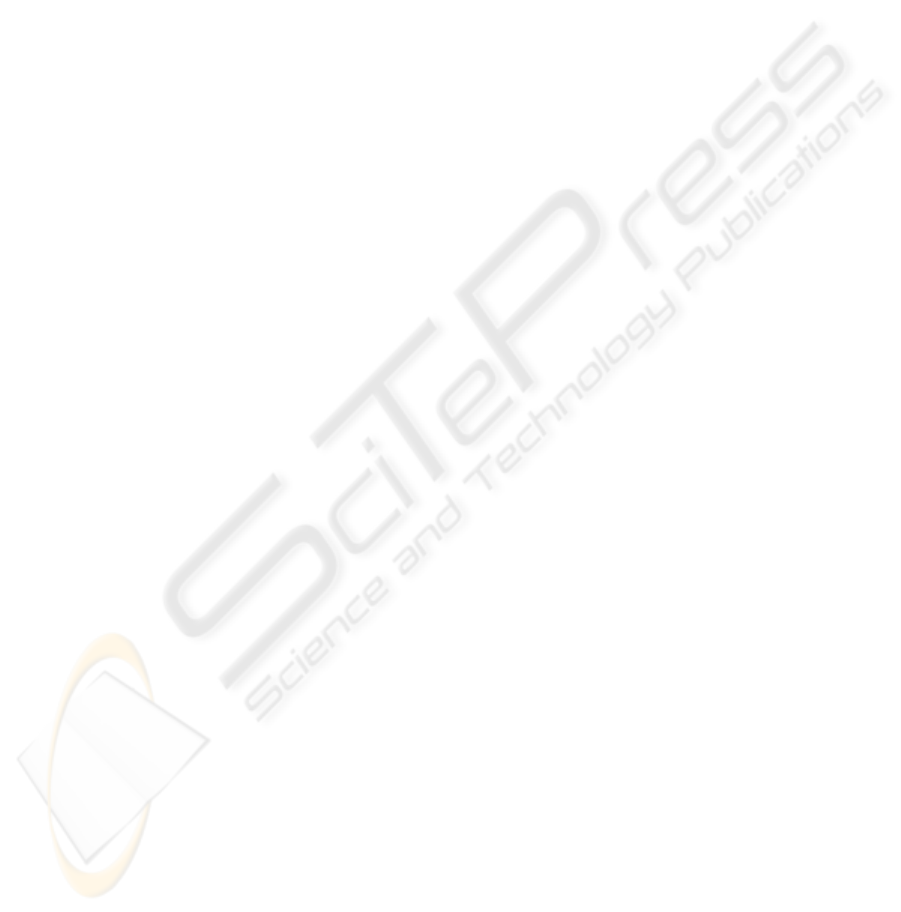
experiment going on, more and more evidences are
gained, so the number of suspicious alarms is
descending.
Table 2 reports the result of classification on the
second level. During the first days, all the numbers
of the different type alarms are very low, which is
caused by the first level, and it could not
discriminate the anomaly alarms from suspicious
ones widely. However, the following days, all of the
numbers ascend greatly, until reaching stable states.
6 CONCLUSIONS
Conventional approaches of alarm classification are
always caused alarms going into the wrong classes
in the early time especially when the evidences used
to classify are in short. To overcome this
shortcoming, this paper proposes a multiple-level
classification model based on the Demper-Shafer
theory. Experiment on DARPA1999 dataset
demonstrates the superiority of our new approach in
handling this problem.
Although the proposed approach of alarms
classification looks promising, more work needs to
be done such as: 1. how to react the intrusions
relating to the classified alarms automatically? 2.
There are still some indistinguishable alarms and
how to handle them?
ACKNOWLEDGEMENTS
This work was supported by Specialized Research
Fund for Doctoral Program of Higher Education of
China (NO.20050217007). In the meanwhile, we
thank the anonymous reviewers for their very
instructive suggestions.
REFERENCES
Chen, T. M. and Venkataramanan, V., 2005. Dempster-
Shafer Theory for Intrusion Detection in Ad Hoc
Networks. Ad Hoc and P2P Security: 35-41.
Cuppens, F., 2001. Managing Alerts in a Multi-Intrusion
Detection Environment. In 17th Annual Computer
Security Applications Conference(ACSAC'01) , New
Orleans, LA. IEEE Press.
Cuppens, F. and Miege, A., 2002. Alert Correlation in a
Cooperative Intrusion Detection Framework. In IEEE
Symposium on Security and Privacy, Oakland, USA,
IEEE Press.
Debar, H., Dacier,M., et al., 1999. Towards a Taxonomy
of Intrusion-Detection Systems. Computer Networks
31: 805-822.
Jian-Wei, Z., Da-Wei, W., et al., 2006. A Network
Anomaly Detector Based on the D-S Evidence
Theory. Journal of Software 17(3): 463-471.
Kruegel, C., Mutz, D., et al., 2003. Bayesian Event
Classification for Intrusion Detection. In Proceedings
of the 19th Annual Computer Security Applications
Conference, Los Alamitos, USA. IEEE Press.
Lee, W. and Stolfo, S. J., 2000. A Framework for
Constructing Features and Models for Intrusion
Detection Systems. In ACM Transactions on
Information and System Security, ACM Press.
Lee, W., Stolfo, S. J., et al., 1999. Data Mining
Framework for Building Intrusion Detection Models.
In 1999 IEEE Symposium on Security and Privacy.
IEEE Press.
Lippmann, R., Haines, J. W., et al., 2000. The 1999
DARPA Off-line Intrusion Detection Evaluation.
Computer Networks 34(4): 579-595.
Mehta, M., Agrawal, R., et al., 1996. SLIQ: A Fast
Scalable Classifier for Data Mining. In Conference on
Extending Database Technology (EDBT'96), Avignon,
France, 1996: 18-33.
Ouali, A., Cherif, A. R., et al., 2006. Data Mining Based
Bayesian Networks for Best Classification.
Computational Statistics & Data Analysis 51(2): 1278-
1292.
Perdisci, R., Giacinto, G., et al., 2006. Alarm Clustering
for Intrusion Detection Systems in Computer
Networks. Engineering Applications of Artificial
Intelligence 19(4): 429-438.
Valdes, A. and Skinner, K., 2001. Probabilistic Alert
Correlation. Recent Advances in Intrusion Detection.
In 4th International Symposium, RAID 2001,Lecture
Notes in Computer Science. Berlin,German. Springer
Press. 2001: 54-68.
Xiang, C. and Lim, S. M., 2005. Design of Multiple-Level
Hybrid Classifier for Intrusion Detection System.
Machine Learning for Signal Processing, IEEE Press.
A NOVEL APPROACH OF ALARM CLASSIFICATION FOR INTRUSION DETECTION BASED UPON
DEMPSTER-SHAFER THEORY
239