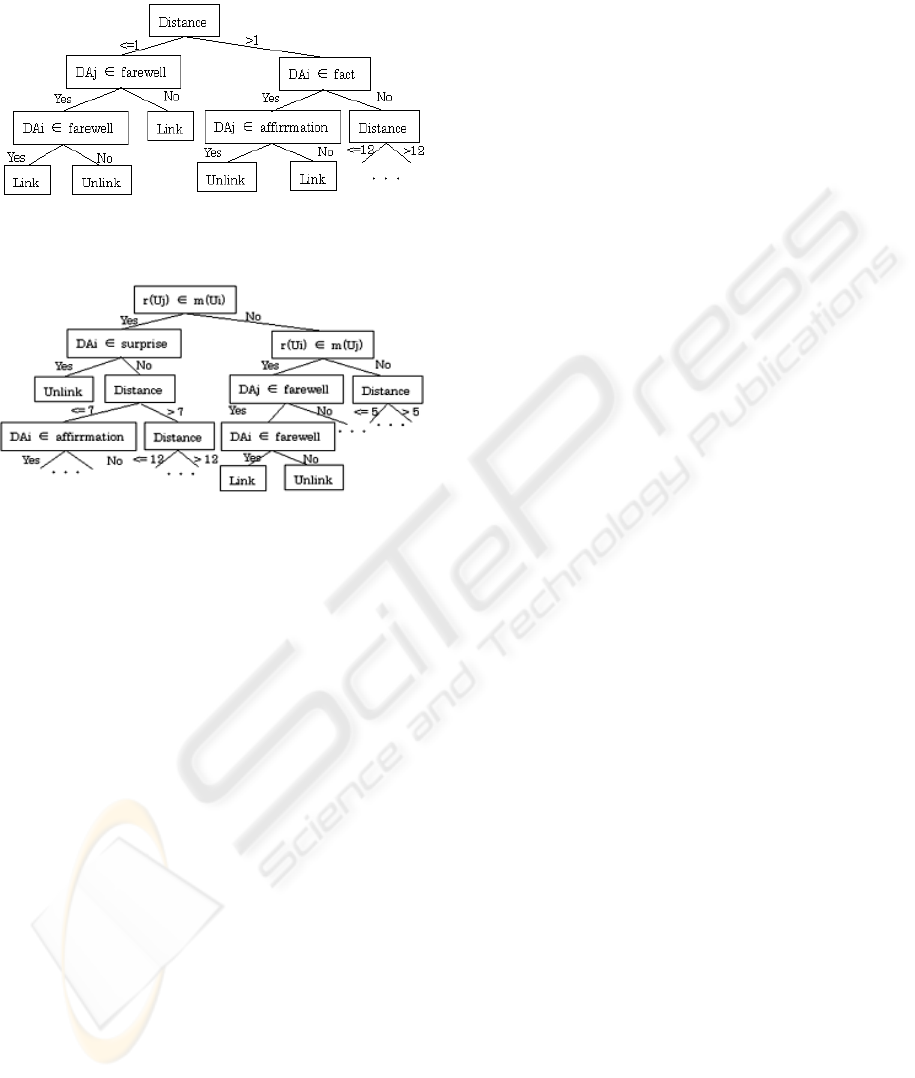
Tree when the attribute pattern is (8) in Table4, and
Figure 11 shows a sub-set when the attribute pattern
is (1) in Table 5.
Figure 10: Sub-set of Decision Tree when attribute pattern
is (8) in Table 4.
Figure 11: Sub-set of Decision Tree when attribute pattern
is (1) in Table 5.
4 CONSIDERATION
In the matter of detecting the links between same
person’s utterances, the result of Decision Tree
clearly shows that the information of DIS is an
important element to detect the links. The
information of recipients is not useful element
because the speakers do not specify themselves as
recipients. Therefore, the linguistic elements are
especially important, it is necessary improve the
estimation accuracy from the linguistic elements. In
the matter of detecting the links between others’
utterances, the result of Decision Tree clearly shows
that the information of DIS greatly influences the
links with the information of NUM.From these
viewpoints, one may say that our system could find
the new links that it was difficult to find from only
linguistic information by using meta-information.
We can recognize from Figure10 that an attribute
(A-2-b) is top of Decision Tree. Hence, we may say
that our assumption that shown in Figure 7 is
significant.
A further direction of this study will be to
improve the estimation accuracy, the precision, and
the recall from the linguistic elements. For example,
it is considered that an effective method is to use the
information of Rhetorical Relation. In addition to
this, it may also be effective method to add other
meta-information.
5 CONCLUSIONS
We proposed the method of learning the links
between two utterances with Decision Tree using
meta-information. Our system could find the new
links that it was difficult to find from only linguistic
information by using meta-information.
The result of the examination of Decision Tree
learning showed the accuracy is 84.1%, the precision
is 87.1% and the recall is 66.9% for link between
same person’s utterances, and the accuracy is 77.5%,
the precision is 68.5% and the recall is 56.8% for
link between others’ utterances. The result of the
examination without meta-information showed the
accuracy is 62.7%, the precision is 86.7%, the recall
is 64.5% for same person’s utterances, and the
accuracy is 77.9%, the precision is 73.8% and the
recall is 49.9% for others’.
REFERENCES
Tokunaga Y., Inui K., and Matsumoto Y., 2006,
Identifying Continuation and Response Relations
between Utterances in Computer-Mediated Chat
Dialogues, The Association for Natural Language
Processing, Journal of Natural Language Processing,
Vol.12, No.1, pp. 79-105.
Ogura K., Ishizaki M., 2003, The Analysis of Surface
Information to Identifying Relevant Utterances in Chat
Conversations, The Japanese Society for Artificial
Intelligence, SIG-SLUD-A203-P05, pp.75-80.
J.R. Quinlan, 1993, C4.5 Programs for Machine Learning,
Morgan Publishers San Mated, California, pp.302.
Andress Stolcke, Elizabeth Shriberg, et al., 1998,
Dialogue Act Modeling for Conversational Speech,
AAAI Spring Symposium, AAAI Press, pp.98-105.
Inui N., Ebe T., Indurkhya B., and Kotani Y., 2001, A
Case-Based Natural Language Dialogue System Using
Dialogue Acts, IEEE International Conference on
Systems, Man and Cybernetics, pp.193-198.
Edward Ivanovic, 2005, Dialogue Act Tagging for Instant
Messaging Chat Sessions, Proceeding of the ACL
Student Research Workshop, pp.79-84.
Nakamura J., Inui N., and Kotani Y., 2005, A method to
extract dialogue relations in the polylogue, IPSJ
Programing symposium, Vol. 46, pp.155-163.
Nakamura J., Inui N., and Kotani Y., 2006, A system of
Neural Network detecting link of utterances in the
polylogue, IPSJ SIG Technical Reports, 2006-NL-173,
pp.53-58.
WEBIST 2007 - International Conference on Web Information Systems and Technologies
424