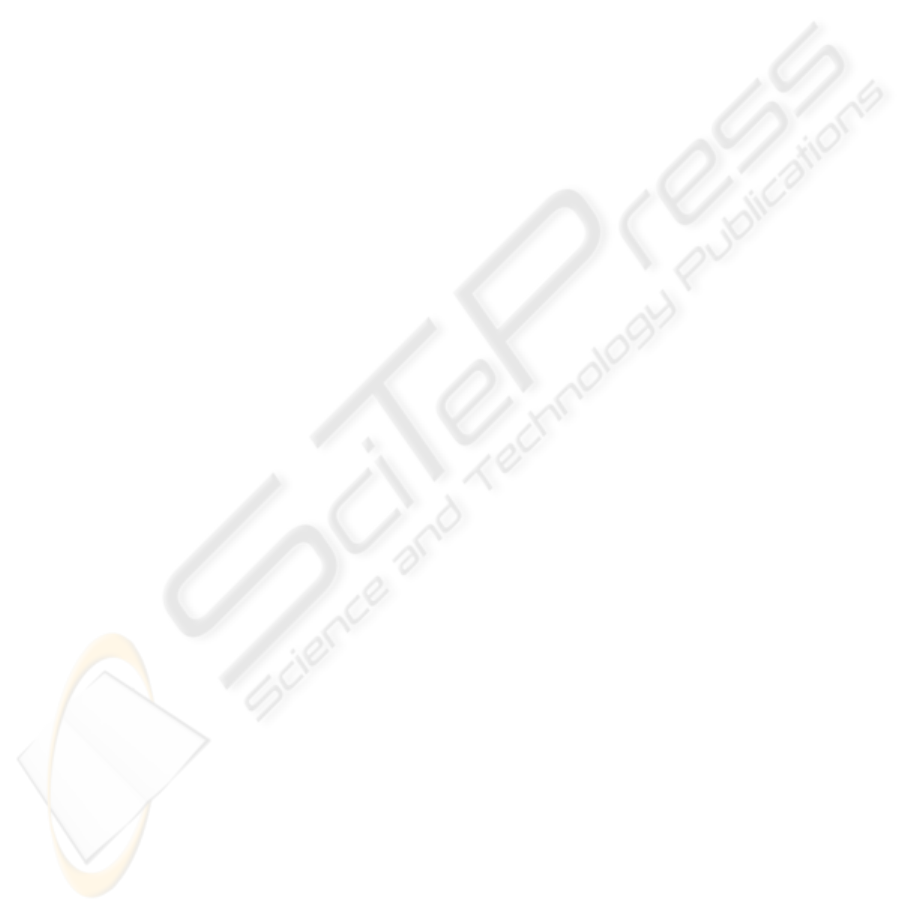
Only relevant links between nodes have been drawn.
A link is relevant when the percentage of students that
follow such link is at least a 5% for both nodes. No-
tice that this graph includes all the possible paths, that
is, not only the first action taken from the initial page.
5 CONCLUSION
In this paper a proposal for embedding hidden marks
in web pages with the aim of user action identifica-
tion has been proposed. Two complementary mark-
ing strategies have been described: the first one, ac-
tion based, consists in adding special parameters in
the calls to applications in the business logics layer of
a web site, in order to capture the specific clicks in
the relevant elements for analysis purposes. The sec-
ond one, content based, consists in hiding invisible
pieces of content that, combined with simple scripts,
generate log entries for both user actions and navi-
gation paths. Preliminary results show that this ap-
proach is useful to face both well known problems
when analyzing large and complex web sites. First,
users’s intentions are better captured as only the rele-
vant actions (for analysis purposes) are logged. Sec-
ond, generated data are only a small fraction of the
original data stored in the web server log files, thus re-
ducing both time and space requirements for off-line
processing. A simple experiment showing user be-
havior the first day of the academic semester has been
described. This experiment reveals interesting infor-
mation, for example, students start sessions reading
all the welcome messages from the teacher and other
students, and replying such messages. This fact could
be used to design a specific initial page for the first
session during first day, when all the other available
services are not requested. Similar ideas for improv-
ing the e-learning environment can be extracted from
the results of each activity analysis, depending on the
educational context.
Current and future research in this topic should in-
clude the use of large scale web usage mining tech-
niques for clustering purposes. Analyzing the evolu-
tion of users’ behavior along a period of time is also
an interesting issue. Finally, as web services are be-
coming more “semantic”, it will be important to es-
tablish the appropriate relationships between the new
services available in the virtual campus and real user
intentions when searching or browsing such services.
ACKNOWLEDGEMENTS
This work has been partially supported by a Span-
ish government grant under the project PERSONAL
(TIN2006-15107-C02-01).
REFERENCES
Chi, E. H., Pirolli, P., and Pitkow, J. (2000). The scent of
a site: a system for analyzing and predicting informa-
tion scent, usage, and usability of a web site. In Pro-
ceedings of the SIGCHI conference on Human factors
in computing systems, pages 161–168, New York, NY,
USA. ACM Press.
Cooley, R., Mobasher, B., and Srivastava, J. (1999). Data
preparation for mining world wide web browsing pat-
terns. Knowledge and Information Systems, 1(1):5–
32.
Hilbert, D. M. and Redmiles, D. F. (2000). Extracting us-
ability information from user interface events. ACM
Computing Surveys, 32(4):384–421.
Kay, J. and Lum, A. (2004). Creating user models from
web logs. In Proceedings of the Intelligent User Inter-
faces Workshop: Behavior-Based User Interface Cus-
tomization.
Marsico, M. D. and Levialdi, S. (2004). Evaluating web
sites: exploiting user’s expectations. International
Journal of Human-Computer Studies, 60(3):381–416.
Mor, E. and Minguill´on, J. (2004). E-learning personaliza-
tion based on itineraries and long-term navigational
behavior. In Proceedings of the Thirteenth Interna-
tional World Wide Web Conference, volume 2, pages
264–265, New York, NY, USA. ACM Press.
Mor, E., Minguill´on, J., and Carb´o, J. M. (2006). Data Min-
ing in E-learning, chapter Analysis of User Naviga-
tional Behavior for E-Learning Personalization, pages
227–246. WIT Press.
Paramythis, A. and Loidl-Reisinger, S. (2004). Adaptive
learning environments and e-learning standards. Elec-
tronic Journal of e-Learning, 2(2).
Sangr`a, A. (2002). A new learning model for the informa-
tion and knowledge society: The case of the UOC. In-
ternational Review of Research in Open and Distance
Learning, 2(2).
Spiliopoulou, M. (2000). Web usage mining for web site
evaluation. Communications of the ACM, 43(8):127–
134.
Thomas, R., Kennedy, G., Draper, S., Mancy, R., Crease,
M., Evans, H., and Gray, P. (2003). Generic usage
monitoring of programming students. In Proceedings
of the ASCILITE 2003 Conference, pages 715–719,
Adelaide, Australia.
Wharton, C., Rieman, J., Lewis, C., and Polson, P. (1994).
Usability inspection methods, chapter The cognitive
walkthrough method: a practitioner’s guide, pages
105–140. John Wiley & Sons, Inc.
CAPTURING USER BEHAVIOR IN E-LEARNING ENVIRONMENTS
469