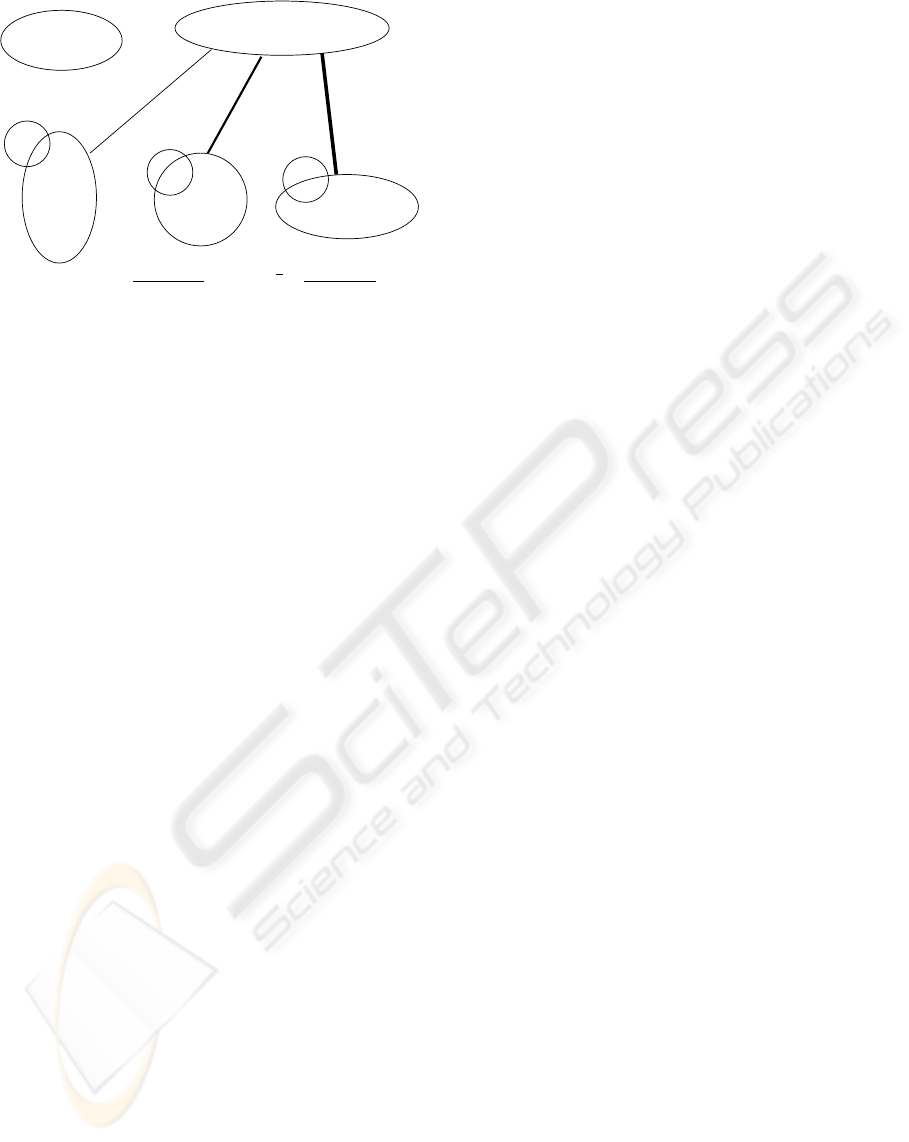
The target page
cache 1
cache 2
cache 3
W
u1
u2
u1
u1
u2
u1
δ1
δ2
δ3
similarity
similarity
similarity
P(u | Z) =
δ1+δ2+δ3
δ1+δ2
δ1 +δ2 + δ3
keywords
keywords
keywords
P(u | Z) =
δ3
Figure 2: The overview of our system.
3 EXPERIMENT
We evaluate our method by an experiment as follows.
cache page number 1886 pages.
Every page in this set can be reachable from
http://www.ipsj.or.jp by following the links. Also,
every page in this set has metadata named
“keywords”. Thus, the crawler for our ex-
periment searches all link paths from the page
http://www.ipsj.or.jp, and takes keyword assigned
pages into the cache set.
test page number 178 pages(set1) and 100
pages(set2).
From this set of pages, a target page is given to the
system as input. The selection of these test set is
random but we take care of variety of sites in the
test set.
In Fig.3, we show the result of experiment of our
method for the test data (set1). Here, n in these fig-
ures represents the size of words of keyword candi-
date. When n = 1, i.e. the output of our system
contains just one word, then the precision is 1 and the
recall is 0 .1 for this test data. For the test data (set2),
we have obtained that the precision is 0.8 and the re-
call is 0.08 with just one candidate.
The average number of keywords in the set of
cache pages is 10.5 for the test data (set1). When
n = 10, we can see that the precision is 0.78 and
the recall is 0.72 for the test data (set1). On the other
hand, for the test data (set2), we haveobtained that the
precision is 0.21 and the recall is 0.20 when n = 10.
In Fig.4, we showthe result of experiment with the
approximation in equation (4). From these graphs, we
can read that if n = 5, i.e. the output of our system
contains five words, then precision is 0.5 and recall
is 0.3 for test data (set1). For the test data (set2), we
have obtained that the precision is 0.2 and the recall
is 0.1 when n = 5.
In both data set, (set1) or (set2), we can read that
the approximation in the equation (4) is worse than
another approximation.
4 CONCLUSIONS
We have shown a metadata finding method for a web
page by selecting suitable item from web cache. In
the evaluation, the result is 74% precision and 76%
recall with data (set1) and n = 10.
For future works, inference of other metadata
item, “subject” for example, is an interesting prob-
lem.
REFERENCES
Heiner Stuckenschmidt, F. v. H. (2001). Ontology-based
metadata generation from semi-structured informa-
tion. In Proceedings of the First Conference on
Knowledge Capture (K-CAP’01), pages 440–444.
Jane Greenberg, Kristina Spurgin, A. C. (2005). Final re-
port for the amega (automatic metadata generation ap-
plications) project. In University of North Carolina at
Chapel Hill.
Jihie Kim, Yolanda Gil, V. R. (2006). Semantic metadata
generation for large scientific workflows. In Proceed-
ings of the 5th International Semantic Web Conference
2006 (ISWC2006), pages 357–370.
J¨urgen Belizki, Stefania Costache, W. N. (2006). Appli-
cation independent metadata generation. In Proceed-
ings of the 1st international workshop on Contextu-
alized attention metadata: collecting, managing and
exploiting of rich usage information(CAMA06), pages
33–36.
Paynter, G. W. (2005). Developing practical automatic
metadata assignment and evaluation tools for internet
resources. In Proceedings of the 5th ACM/IEEE-CS
joint conference on Digital libraries, pages 291–300.
Solomon Atnafu, Richard Chbeir, L. B. (2002). Effi-
cient content-based and metadata retrieval in image
database. In Journal of Universal Computer Science,
volume 8, pages 613–622.
WEBIST 2007 - International Conference on Web Information Systems and Technologies
476