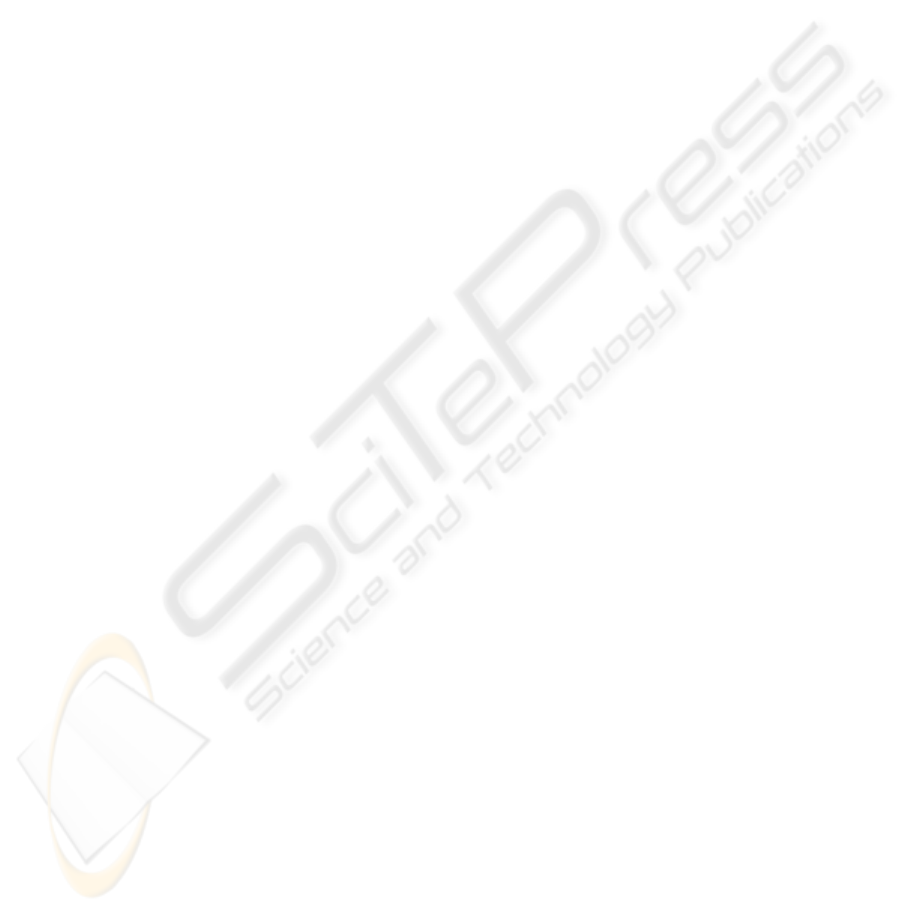
that may be applied in order to improve the accuracy
of models obtained by using one algorithm. We used
state-of-the-art methods of combining models of
analysis like bagging, boosting and stacking.
Our dataset consisted of 375 instances
represented by students that were registered as
students within e-Learning platform. For each
student the activity was represented in terms of four
parameters: the number of loggings, the number of
taken tests, the average of taken tests and the
number of sent messages.
Bagging, boosting and stacking techniques were
used with the aim of creating models of data
representation with greater accuracy.
Bagging exploited the instability that is inherent
in learning systems. Combining multiple models
helps when these models are significantly different
from one another and each one treats a reasonable
percentage of the data correctly. Ideally the models
complement one another, each being a specialist in a
part of the domain where the other models don’t
perform very well.
Boosting produced a classifier that was more
accurate than one generated by bagging. However,
unlike bagging, boosting sometimes generates a
classifier that was significantly less accurate than a
single classifier built from the same data. This
indicates that the combined classifier overfit the
data. The time sent for building the model is greater
for boosting that for bagging although the algorithm
complexity is the same. The difference comes from
computational complexity which is greater in
boosting due to the weight introduced as parameter
for each instance.
The best performance regarding accuracy was
obtained by using stacked generalization.
Combination of three different types of learning
algorithms proved to achieve better classification
accuracy than both previous ways of combining
models (bagging and boosting) that used only one
type of learner. The obtained performance was
obtained with a high cost regarding computational
time.
The obtained accuracy is the guarantee that
obtained knowledge from the analysis process is
valid and may be used together with domain
knowledge to improve the performance of the e-
Learning platform.
In future, we plan using the same platform for other
students. It is our primary concern to create analysis
models that are able to classify students according to
their accumulated knowledge. The platform,
represented by the entire infrastructure (disciplines,
course materials, and test and exam questions)
represents an invariant. On the same platform setup,
different methods of analyzing student’s activity
may be employed. Future work will take into
consideration other ways of combining different
models of analysis that have good accuracy and
produce knowledge that may be used to improve our
e-Learning system. Changes that are made at
platform’s infrastructure should be noted very
carefully and analysis process should be repeated in
order to look for correlations. An interesting thing
would be to evaluate the analysis process on data
from other e-Learning systems.
REFERENCES
Olivia Parr Rud, “Data Mining Cookbook – Modeling
Data for Marketing, Risk, and Customer Relationship
Management”, Wiley Computer Publishing, 2001.
Jiawei Han, Micheline Kamber “Data Mining – Concepts
and Techniques” Morgan Kaufmann Publishers, 2001.
Ian H. Witten, Eibe Frank “Data Mining – Practical
Machine Learning Tools and Techniques with Java
Implementations” Morgan Kaufmann Publishers,
2000.
http://www.cs.waikato.ac.nz/ml/weka
R. Agrawal and R. Srikant, “Fast algorithms for mining
association rules,” Proc. of the 20th VLDB
Conference, pp. 487-499, Santiago, Chile, 1994.
Nasraoui O., Joshi A., and Krishnapuram R., “Relational
Clustering Based on a New Robust Estimator with
Application to Web Mining,” Proc. Intl. Conf. North
American Fuzzy Info. Proc. Society (NAFIPS 99),
New York, June 1999.
B. Mobasher, N. Jain, E-H. Han, and J. Srivastava “Web
mining: Pattern discovery from World Wide Web
transactions,” Technical Report 96-050, University of
Minnesota, Sep, 1996.
R. Quinlan. C4.5: Programs for Machine Learning,
Morgan Kaufmann Publishers, San Mateo, CA, 1993.
Schapire, R.E., Y. Freund, P. Bartlet, and W.S. Lee,
“Boosting the margin: A new explanation for the
effectiveness of voting methods”, Proc. Fourteenth
International Conference on Machine Learning,
Nashville, San Francisco, 1997.
Wolpert, D.H., “Stacked generalization”, Neural
Networks, 1992.
http://stat257.central.ucv.ro/
B. Cestnik, “Estimating probabilities: A Crucial Task in
Machine Learning”, Proc. of European Conference on
Artificial Inteligence, 1990.
Ting, K.M. and Witten, I.H., “Stacked generalization:
when does it work?” Proc International Joint
Conference on Artificial Intelligence, pp. 866-871,
Japan, August, 1997.
Leo Breiman, “Stacked regression”, Machine Learning,
Vol. 24, pp. 49-64, 1996.
Michael LeBlanc, Robert Tibshirani, “Combining
Estimates in Regression and Classification”, Technical
Report 9318, Department of Statistics, University of
Toronto, Canada, 1993.
ICSOFT 2007 - International Conference on Software and Data Technologies
258