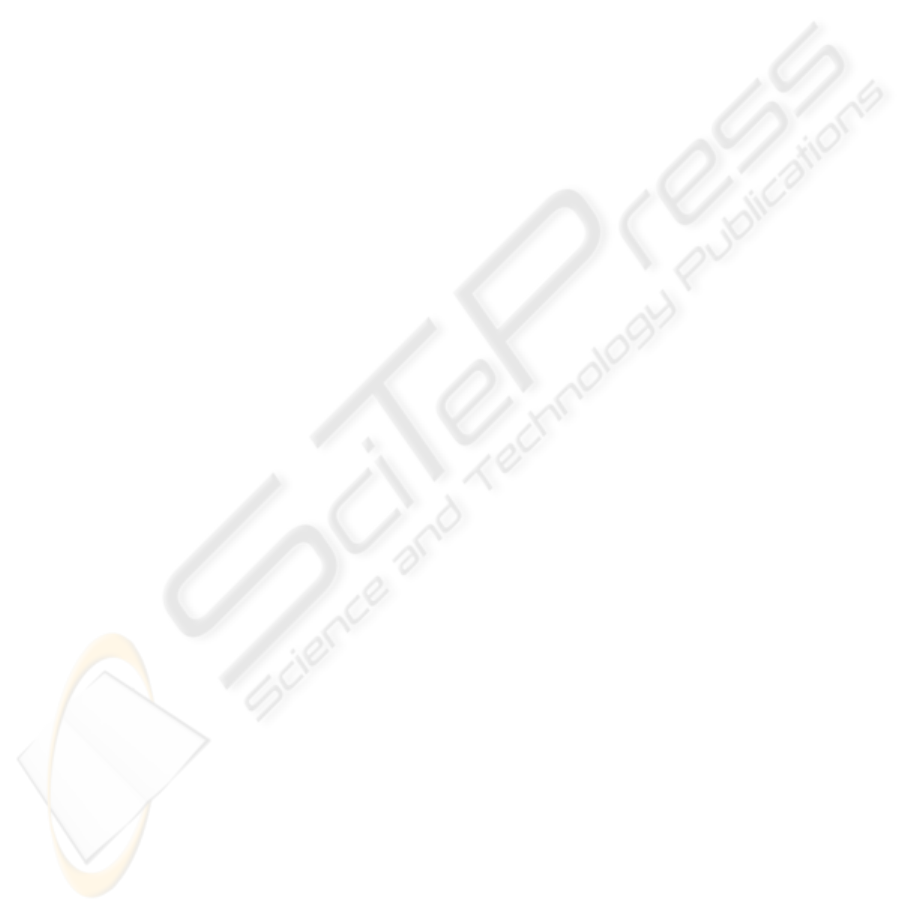
test, B3, the implementation again backlogged and
grew at a linear rate. The SPT had similar behavior,
and therefore was considered accurate. The CPN
predicted no backlog on this test.
4 CONCLUSION
In conclusion, the experimental results showed that
the SPT combined with a statistical simulation was
accurate the majority of the time. With respect to
predicting maximum queue size, the SPT was
accurate on 78 percent of the tests making it more
accurate than the CPN, which was accurate on 11
percent of the tests. When the SPT approach was
inaccurate, it consistently overestimated problems.
On the CPN’s inaccurate tests, it consistently
underestimated performance problems.
The next metric examined was throughput. The
SPT approach produced accurate values on 56
percent of the tests, while the CPN was accurate on
33 percent of the tests. Since the SPT approach was
accurate on the majority of the tests, it was
considered the more accurate technique. On the
SPT’s inaccurate tests, it constantly overestimated
performance problems. On the CPN’s inaccurate
tests, it again underestimated the performance
problems.
The last metric studied was the queue behavior
over time. The SPT was accurate on 56 percent of
the tests and the CPN was accurate on 11 percent of
the tests. Therefore the SPT approach was
considered the more accurate for predicting queue
size over time. On its inaccurate tests, SPT
consistently overestimated the problem or identified
non-existent problems. Again, the CPN consistently
underestimated or missed the performance problems.
In summary, the data suggests that the SPT
Profile combined with statistical simulation is more
accurate than CPNs, which supports the hypothesis.
However, as discussed in section 3.4, the data is
from a single experiment, and may not have broad
applicability. It was also observed that the SPT
consistently overestimated problems and identified
non-existent problems. This would lead to
unnecessary changes in design; however it
guarantees that all existing problems would be fixed.
On the other hand, the CPN consistently
underestimated or missed performance problems.
This would avoid unnecessary change in design, but
not all problems would be fixed. Therefore it is
better to err on the side of overestimation to ensure
that all problems are fixed. This further suggests the
use of SPT with statistical simulation over CPNs
REFERENCES
Bennett, A. J. & Field, A. J. (2004) Performance
Engineering with the UML Profile for Schedulability,
Performance and Time: a Case Study. IN IEEE (Ed.)
12th International Workshop on Modeling, Analysis,
and Simulation of Computer and Telecommunication
Systems (MASCOTS 2004). Vollendam, The
Netherlands.
CPN Group At The University of Aarhus, D. (2004)
Design/CPN. 4.0 ed., CPN group at the University of
Aarhus, Denmark.
GERONESOFT Code Counter Pro. 1.27 ed., Geronesoft.
Gomaa, H. (2000) Designing Concurrent, Distributed, and
Real-Time Applications with UML, Boston, Addison-
Wesley Object Technology Series.
Graf, S., Ober, I. & Ober, I. A real-time profile for UML
Software Tools for Technology Transfer manuscript.
Hakansson, J., Mokrushin, L., Pettersson, P. & YI, W.
(2004) An Analysis Tool for UML Models with SPT
Annotations. Workshop on SVERTS: Specification and
Validation of UML models for Real Time and
Embedded Systems. Lisbon, Portugal.
Hooman, J., Mulyar, N. & Posta, L. (2004) Validating
UML models of Embedded Systems by Coupling
Tools. Workshop on SVERTS: Specification and
Validation of UML models for Real Time and
Embedded Systems. Lisbon, Portugal.
Minh, D. L. (2001) Applied Probability Models, Pacific
Grove, CA, Brooks/Cole.
Woodside, M., Petriu, D. C., Petriu, D. B., Shen, H., Israr,
T. & Merseguer, J. (2005) Performance by Unified
Model Analysis (PUMA). Fifth International
Workshop on Software and Performance (WOSP 05).
Palma, Illes Balears, Spain.
ICSOFT 2007 - International Conference on Software and Data Technologies
420