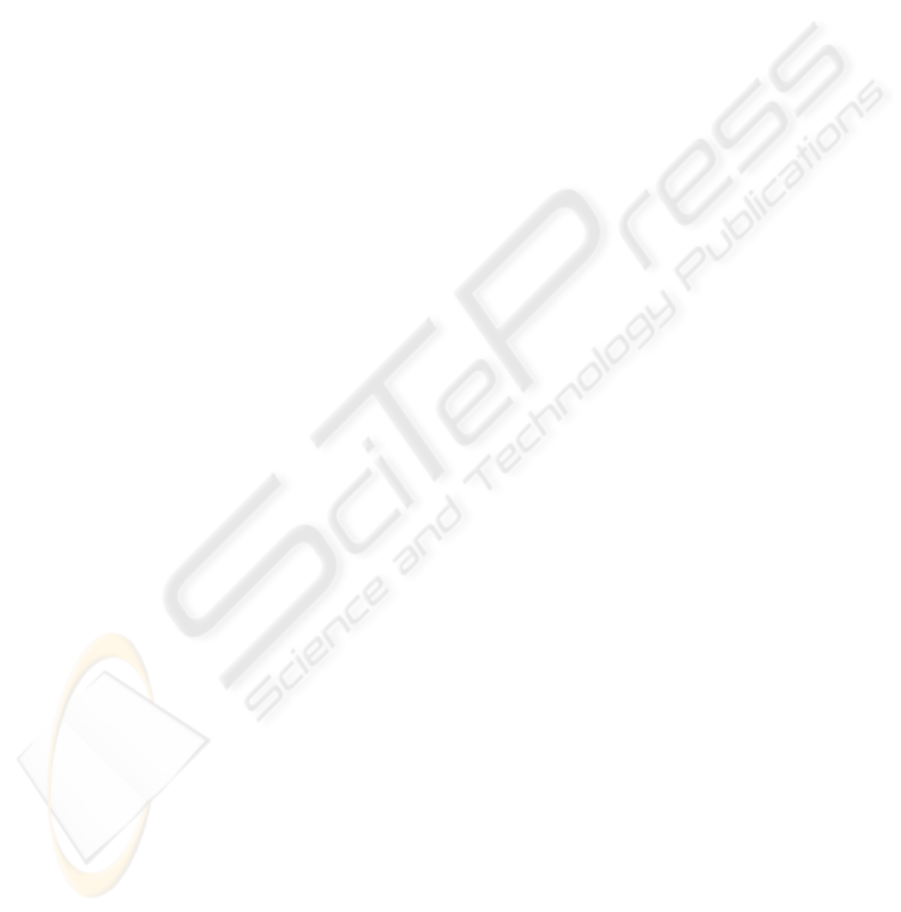
(Le Goc and al, 2005). This is the reason why one of
the main requirements for our modelling approach is
to be compatible with this approach of learning.
6 CONCLUSION
This paper presents the basis of a multimodeling
methodology that uses a CommonKADS conceptual
model to interpret the knowledge source with the
aim of representing the system with three models: a
structural model describing the relations between the
components of the system, a functional model
describing the relations between the values the
variables of the system can take (i.e. the functions)
and a behavioral model describing the states of the
system and the discrete events firing the state
transitions. The relation between these models is
made with the notion of variable: a variable used in
a function of the functional model is associated with
an element of the structural model and a discrete
event is defined as the affectation of a value to a
variable.
This methodology is presented in this paper with
a toy but pedagogic problem: the technical diagnosis
of a car with a given knowledge base (Schreiber and
al, 2000). This example shows that the resulting
models are compatible with Reiter’s theory of
diagnosis and that a specific reasoning is required to
take advantage of the behavioural model of the
dynamic system to diagnose. Such reasoning must
take into account the time of the observations. This
example illustrates clearly our goal: making explicit
the models used by experts to formulate their
knowledge. The idea is that using the same level of
abstraction that the expert can facilitate the problem
solving reasoning. This method has been applied to a
real world dynamic system, the Cubblize dam,
confirming the conclusions presented in this paper
and validating the method (Masse and Le Goc,
2007). It is to note finally that the resulting models
can be used either fore the design or the simulation
phases.
Our current work aims at formalizing the global
methodology and to design of a diagnosis algorithm
able to use a behavioural model that can be built
according to the timed relation the Stochastic
Approach of knowledge learning discovers.
REFERENCES
Basseville, M. and Cordier, M-O., 1996. Surveillance et
diagnostic de systèmes dynamiques : approches
complémentaires du traitement de signal et de
l’intelligence artificielle, office publication.
Bouché, P., Le Goc, M., and Giambiasi, N., 2005.
Modeling Discrete Event Sequences for Discovering
Diagnosis Signatures, Summer Computer Simulation
Conference, SCSC'05, Philadelphia, USA.
Clancey, W., 1985. Heuristic classification, Artificial
Intelligence Journal 25(3) 289-350.
Cordier, M.O., Dousson, C., 2000. « Alarm driven
monitoring based on chronicles », Proceedings of
SafeProcess 2000, pp.286-291, Budapest, Hungary.
Chittaro, L., Guida, G., Tasso, C., and Toppano, E., 1993.
Functional and teleological knowledge in the
multimodeling approach for reasoning about physical
systems: a case study in diagnosis. IEEE transactions
on systems.
Dagues, P., 2001. « Théorie logique du diagnostic à base
de modèles », in Diagnostic, intelligence artificielle et
reconnaissance des formes, Hermes, p. 17-105.
Le Goc, M., Bouché, P. and Giambiasi, N., 2005.
Stochastic modeling of continuous time discrete event
sequence for diagnosis. Proceedings of the 16th
International Workshop on Principles of Diagnosis,
DX-05, Pacific Grove, California, USA.
Le Goc, M., Bouché, P., Giambiasi, N., 2006. DEVS, a
formalism to operationnalize chronicle models in the
ELP Laboratory. Proceedings of DEVS’06, DEVS
Integrative M&S Symposium, Part of the 2006 Spring
Simulation Multiconference (SpringSim'06), pp. 143-
150, Van Braun Convention, Huntsville, Alabama,
USA, April 2-6 2006.
Masse, E., and Le Goc, M., 2007. Modeling Dynamic
Systems from their Behavior for a Multi Model Based
Diagnosis. To appear in the proceedings of the 18th
International Workshop on the Principles of Diagnosis
(DX‘07), Nashville, USA, Mai 29th to 31th 2007.
Reiter, R., 1987. A theory for Diagnosis from First
Principles, Artificial Intelligence 32, P 57-95.
Schreiber, A. Th., Akkermans, J. M., Anjewierden, A. A.,
de Hoog, R., Shadbolt, N. R., Van de Velde W.,
Wielinga B. J., 2000. Publication, Knowledge
Engineering and Management, The CommonKADS
methodology, MIT Press.
Thetiot, R., 1999. PhD in Sciences, Utilisation de
l’approche multi-modèles pour l’aide au diagnostic
d’installations industrielles, Université d’Evry Val d’
Essonne.
Zanni, C., Le Goc, M., Frydman, C., 2005. Publication, A
conceptual framework for the analysis, Classification
and choice of knowledge-based diagnosis systems,
International Journal of Knowledge-Based &
Intelligent Engineering Systems (KES Journal), IOS
Press Eds., 41 p.
Zouaoui, F., 1998. PhD in Sciences, Aide à
l’interprétation du fonctionnement des systèmes phy-
siques en utilisant une approche multi-modèles.
Application au circuit primaire d’une centrale à eau
pressurisée, Université de Paris XI – Orsay.
ICSOFT 2007 - International Conference on Software and Data Technologies
282