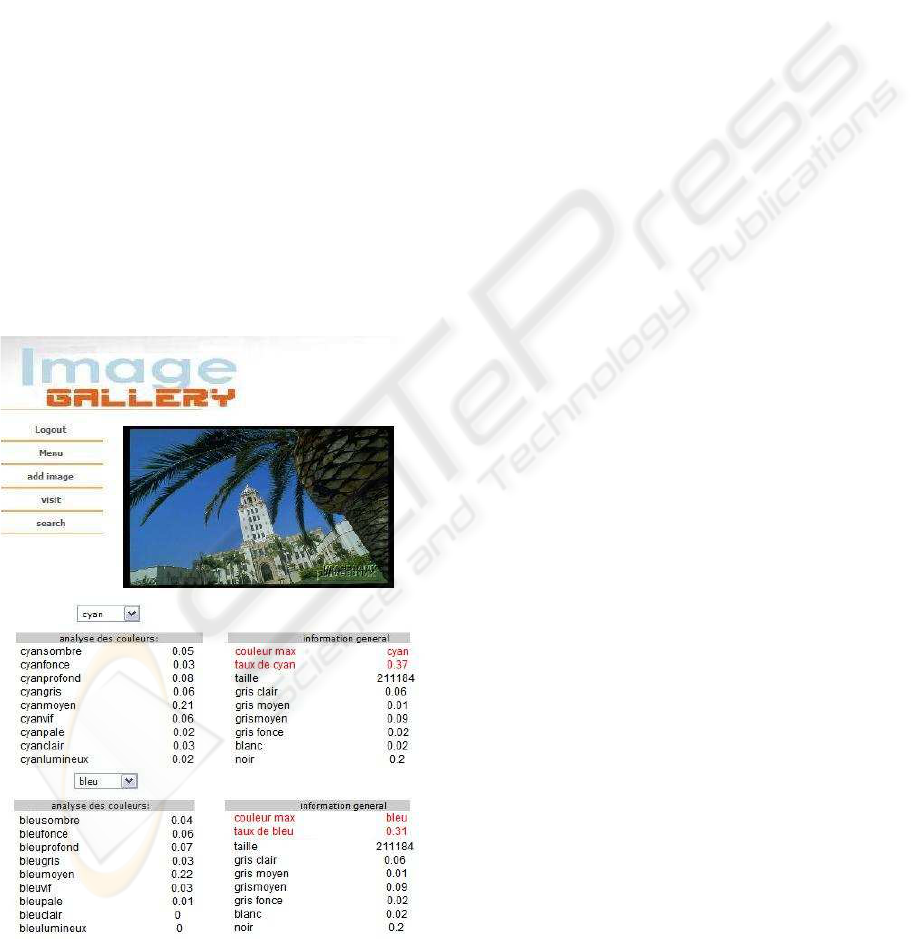
by trapezoidal fuzzy subset; all other colors are repre-
sented by triangular fuzzy subsets.
Each image inserted in the database is processed ac-
cording to the standard setting of colors; each pixel
is accessed and the RGB values are converted into the
HLS color space. By applying the functions f
i
and
˜
f
q
,
the coordinates of each pixel in HLS are transformed
into membership degree in colors and qualifiers. So
a pixel may be considered 0.7 red and 0.3 orange. At
the end, the colorimetric profile of the image is con-
structed. We store all values of the profile in the table
prof. In the application, we can see for each image in
the menu visit the detailed colorimetric parameters.
In the menu search we can retrieve images by supply-
ing an argument such as blue, red pale etc. To test our
method of comparability and compatibility we insert
in the table HLS a new row. For example we keep
all values except for the colors cyan and blue: cyan
is shifted to the right while blue is shrunk. We ap-
ply the rules of comparability and compatibility on a
image/row in table photo. Now any image containing
some blue has a new colorimetric profile: the values
for cyan increase. Figure 5 shows the results.
Figure 5: When modifying blue and cyan settings.
6 CONCLUSION
In this paper a new method is proposed to calculate
the membership degree of colors in an image. Ini-
tially we start building a colorimetric profile of the
image based on an initial set of colors and qualifiers.
Since this initial set may vary according to the user’s
perception, we developed an algorithm that shall com-
pute the new colorimetric profile. The computation of
the new profile is based on the profile constructed in
the initial phase and not on the image itself. Future
work, which has been already started, is to model the
user’s perception. The fuzziness of perception makes
its modeling a challenge and gives motivation to use
the fuzzy logic terms to do mapping between abstract
and concrete objects. We will focus on the dynamic
construction of the users’ profiles, which will increase
their satisfaction by being more personalized and ac-
commodated to their particular needs. Our work will
not affect only the image retrieving domain, but all
themes that rely on subjectivity and perceptions.
REFERENCES
A
¨
ıt Younes, A., Truck, I., and Akdag, H. (2007). Image
retrieval using fuzzy representation of colors. In Soft
Computing - A Fusion of Foundations, Methodologies
and Applications, 11 (3), pp 287-298. Springer.
Boust, C., Chahine, H., Vi
´
enot, F., Brettel, H., Chouikha,
M. B., and Alqui
´
e, G. (2003). Color correction judge-
ments of digital images by experts and naive ob-
servers. In Proceedings of PICS 2003, pp. 4-9. PICS
2003.
Chen, Y. and Wang, J. Z. (2002). A region-based fuzzy
feature matching approach to content-based image re-
trieval. In IEEE Transactions on Pattern Analysis and
Machine Intelligence 24(9), pp. 1252-1267.
CIE (1987). Cie international lighting vocabulary. In CIE
International Lighting Vocabulary. CIE Publ.
Couwenbergh, J. P. (2003). Guide complet et pratique de la
couleur. Eyrolles, Paris, 2nd edition.
Herrera, F. and Mart
´
ınez, L. (2001). A model based on lin-
gustic two-tuples for dealing with multigranularity hi-
erarchical linguistic contexts in multiexpert decision-
making. In Transactions on Systems, Man and Cyber-
netics, Part B, 31(2), pp.227-234. IEEE.
Omhover, J. F., Detyniecki, M., and Bouchon-Meunier, B.
(2004). A region-similarity-based image retrieval sys-
tem. In Proceedings of IPMU’04.
Truck, I. and Akdag, H. (2003). Supervised learning us-
ing modifiers: Application in colorimetrics. In Pro-
ceedings of the ACS/IEEE International Conference
on Computer Systems and Applications.
COLOR IMAGE PROFILE COMPARISON AND COMPUTING
231