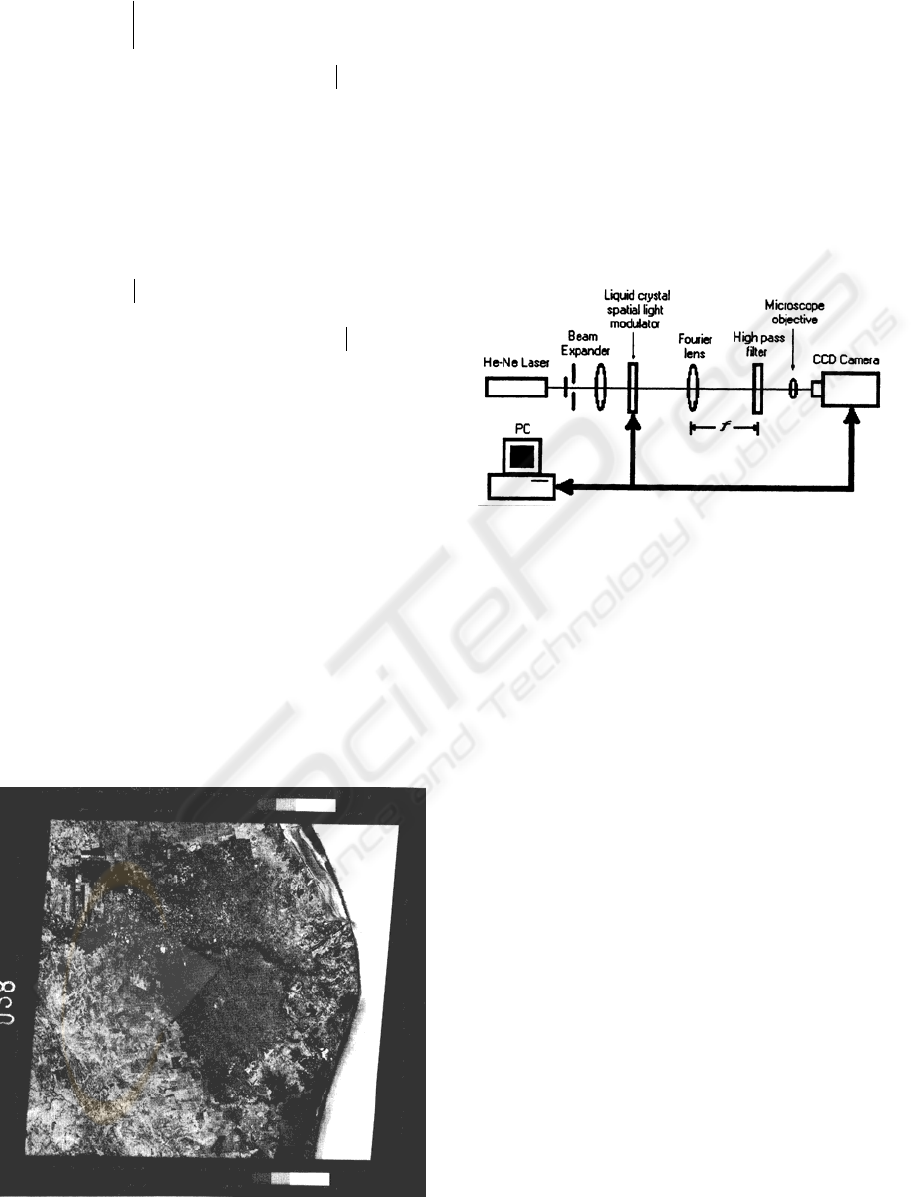
() ()
∫∫
=
)(
,,
D
yxfqpI
()
[]
2
dd2exp yxyqxpi +−×
π
, (11)
where
fxp
/
′
= , fyq
/
= ,
is the wavelength
of illumination, and f is the focal length of the lens.
Using the polar coordinates for input and output
planes of the Fourier processor, Eq. (11) may be
rewritten as follows:
() ()
ϕθρ
π
,,
2
00
rfI
R
∫∫
=
()
[]
2
dd2exp
ϕθϕρπ
rrri −−× . (12)
Comparing the latter equation with Eq. (2) and (5),
we come to conclusion that the sample power
spectrum
()
RS
nk
;
may be easily calculated in any
PC-compatible system connected with CCD array.
4 EXPERIMENTAL RESULTS
We performed a physical simulation experiment on
segmentation of true satellite (Landsat) images into
homogeneous regions that correspond to four
different classes of terrestrial surface, to wit, “sea”,
“mountains”, “crops” and “settlement”. An example
of such an image is shown in Fig. 2. Each class of
texture images to be classified was considered a
spatially stationary and isotropic random field.
Figure 2: True satellite image used in the experiment.
The experimental setup is sketched schematically
in Fig. 3. The images to be processed in this setup
were previously converted into numerical files using
a standard scanning technique. To provide the
capture of the digitalized images into the optical
Fourier processor, the liquid crystal spatial light
modulator HoloEye-LC2002 (800
× 600 pixels)
controlled by PC was used. The detection of the
light distribution in the output plane of the Fourier
processor was realized by means of the CCD camera
SONY-SSC-M374 (768
494 pixels).
Figure 3: Experimental setup.
In our experiment, at the stage of SSDF
synthesis, we used 50 images of 10
× 10 mm for
each of four classes. At the stage of segmentation we
used the composed full-scale image of 100
100
mm and realized its 2-D scanning by an aperture of
5
5 mm with a discrete step of 5 mm. At every step
of image scanning the corresponding texture image
was classified in accordance with the SSDF method.
Calculation of SSDFs and values
mk
u
0
(see Eq. (8))
was realized in a PC-system using specially
designed software. Decision on image class at every
step of scanning was made on the basis of
thresholding the output data. If none or more than
one of
mk
u
0
values had exceeded the threshold, the
corresponding image region was considered to be
unclassified. The result of four-class segmentation
that corresponds to the satellite image in Fig. 2 is
shown in Fig. 4. The regions of the segmented image
labeled by “
0” correspond to unclassified images.
As can be seen, in the main these regions correctly
repeat the true shape of the boundaries between
terrestrial surface images of different classes, but, in
certain cases, they occupy a rather large area of the
image. The latter can be explained by the fact that,
in reality, our satellite image contains textures of
more than four classes mentioned above (e.g.,
regions of the terrestrial surface covered by clouds),
ICINCO 2007 - International Conference on Informatics in Control, Automation and Robotics
410