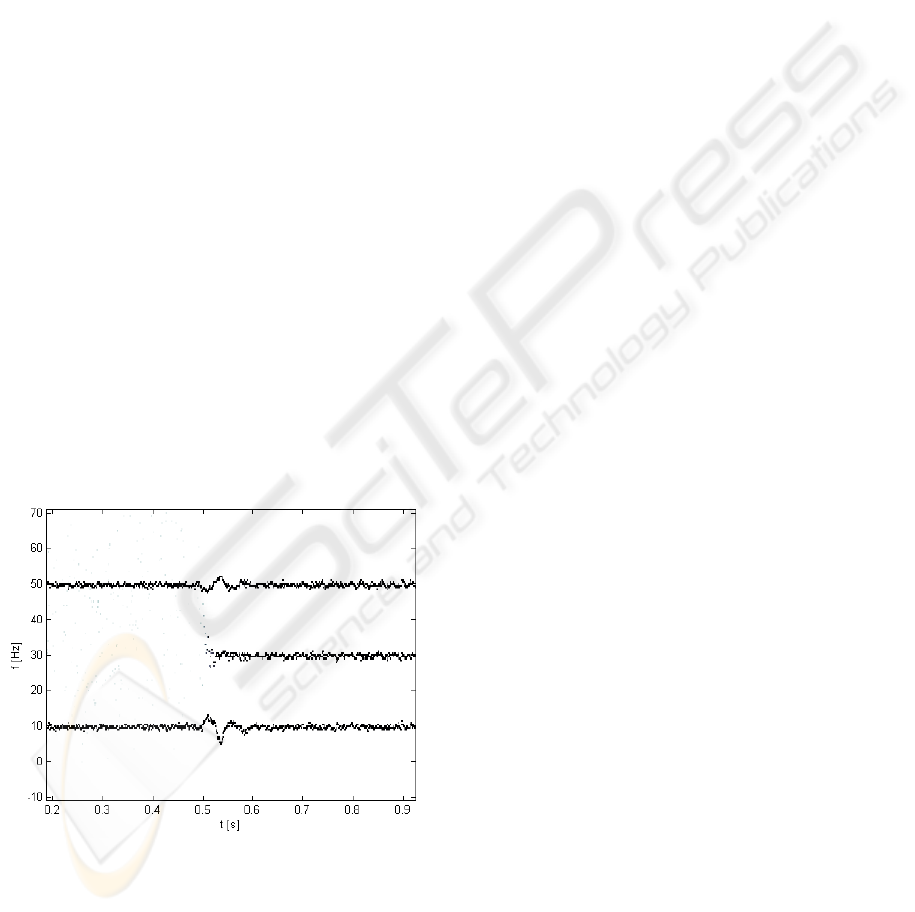
The additive noise in simulation signal is the cause
of the instantaneous frequency oscillating.
The algorithm based on Kalman estimation is
also illustrated on another two non-stationary test
signals s
2
(t) and s
3
(t). The initial conditions of
Kalman estimator were set up as mentioned above
and initial frequencies of the model were obtained
through Burg’s AR linear prediction filter of order
25 as maxima in estimated power spectrum (n=10).
The test signal s
2
(t) consists of two components
in time-frequency domain - stationary harmonic
signal with constant frequency and concave
parabolic chirp signal. Both components exist in
time between t = 100 and t = 900. The results of the
Kalman estimation is compared with the methods
mentioned in Section 2 and the results are shown in
Figure 4. The output of Kalman estimation in time-
frequency domain has relatively better time-
frequency resolution in both components than the
other methods.
The test signal s
3
(t) consists of four harmonic
components and the accuracy of the method to
identify the frequency and also the time of the origin
and end of the components is tested. The signal
begins again in time t = 100 and ends in t = 900. The
frequency changes in t = 300 and t = 600. There are
two components simultaneously in time between
t = 300 and t = 600. The ability of methods to
distinguish between these two frequencies is visible
in Figure 5. The smoothed pseudo Wigner-Ville
distribution and Kalman filter have better time-
frequency resolution compared to short-time Fourier
transform and to Morlet wavelet.
Figure 2: Estimation of instantaneous frequency of test
signal components.
The last example is the transform of the acoustic
signal from the real equipment where the
instantaneous event took place. The signal was
measured with 80 kHz sampling rate. For
comparison, in figure 3, the time-frequency-
amplitude responses of the short-time Fourier
transform (STFT) and of the Kalman estimator
approach are compared. The black column at first 4
milliseconds in the left spectrogram is the adaptation
phase of Kalman filter. This example was obtained
with following initial conditions: Burg’s filter of
order 400 was used to identify the power spectral
density and all local maxima (n=148), which satisfy
the inequality
>ma
10
-7
were appointed as
monitored frequencies. All resonance frequencies (5,
6, 14 and 27kHz) in the STFT spectrogram are also
presented in the left one (Kalman). An event which
occurs at time 0.022 seconds is displayed also in
both spectrograms (see the frequency band 2 -
15 kHz). It is visible that the Kalman version of
spectrogram offers a better resolution in time and
frequency than the spectrogram obtained through
STFT.
6 CONCLUSIONS
The new method for obtaining the time-frequency
representation of instantaneous frequency has been
introduced in this work. The procedure is based on
the Kalman estimation and shares its advantages
regarding the suppression of measurement noise. In
this method the Kalman filter serves for dissociation
of signal into modes with well defined instantaneous
frequency. Simultaneously the time progression of
signal components is estimated. This procedure
utilizes the adaptive feature of the Kalman filter.
In cases where the short–time Fourier transform
cannot offer sufficient resolution in frequency-time
domain, there can be taken advantage of this method
despite of higher computational severity. In
vibrodiagnostic methods, where frequency-time
information is used for localizing of non-stationary
events, the sharpness of the introduced method can
be helpful for the improvement of the event
localization.
ACKNOWLEDGEMENTS
This work was supported by AREVA NP GmbH,
Department SD-G in Erlangen (Germany) and from
the specific research of Department of Cybernetics
at the University of West Bohemia in Pilsen.
ICINCO 2007 - International Conference on Informatics in Control, Automation and Robotics
44