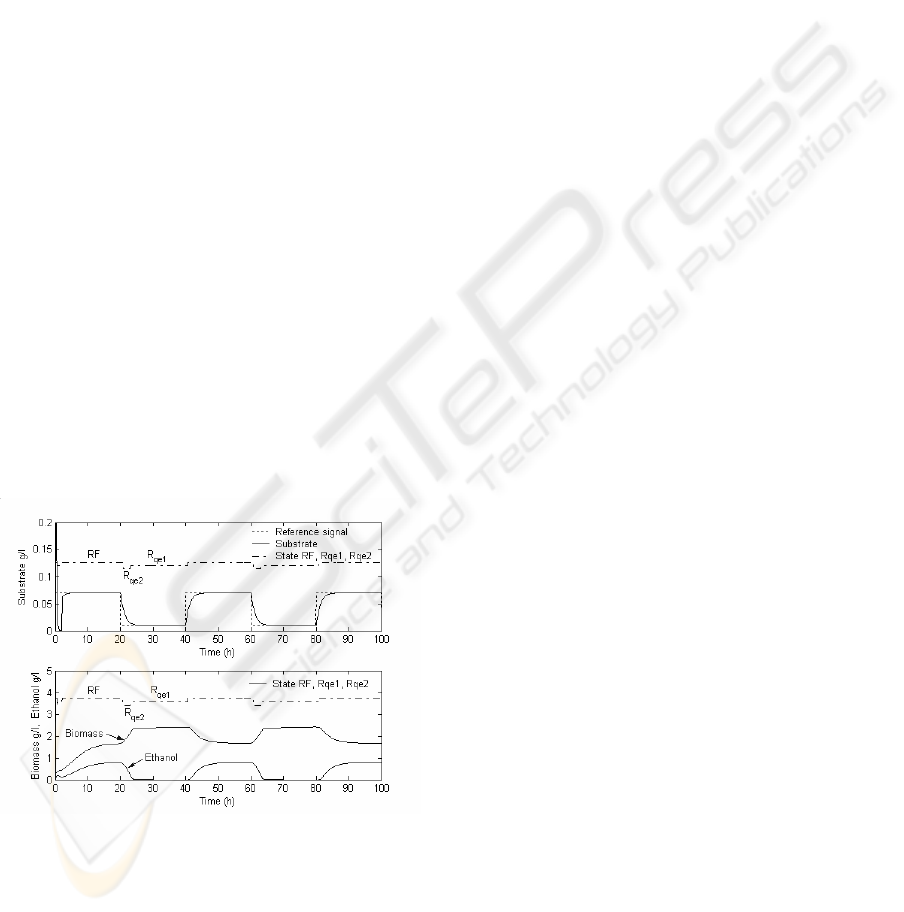
{}
64
1
() ( ()) () () ,
RF RF RF
ijklmn ijklmn ijklmn R
thztABF tytd
ψ
ξ
⎡⎤
=−++
⎣⎦
∑
(14)
where
2( 1) 4( 1) 8( 1)
16( 1) 32( 1),
nm l k
ji
=+ −+ −+ −
+−+−
1
234
11 2 2 3
45 6
( ( )) ( ( )) ( ( )) ( ( ))
( ( )) ( ( )) ( ( ))
ijkx
lx mx nx
hzt M ztM ztM zt
ztM ztM zt
ψ
=
×
(15)
It has to be noticed that x
1
, x
3
and x
4
are not
taken into account in the PDC design; this is because
these states are not intended to be stabilized but to
switch between the RF and R partial models. The
fuzzy controller for the models Rqe1 and Rqe2 were
constructed following the same procedure.
4 SIMULATION RESULTS
The application of the proposed controller scheme
was simulated using MATLAB™. In order to force
the switching between the RF and the R baking yeast
partial models, the substrate fuzzy controller was
forced to track a square reference signal, varied
between 0.01 g/l and 0.07 g/l. S
in
was set to 5 g/l.
The behavior of the substrate fuzzy tracking
controller as well as the biomass and ethanol
behavior are shown in figure 1.
Figure 1: Fuzzy controller performance and biomass and
ethanol behavior.
It can be notice that on the RF model, ethanol is
produced, limiting the biomass growth; when the
Rqe2 partial model is enable the ethanol is
consumed promoting biomass growth, and on the
Rqe1 state partial model the ethanol is consumed
and the biomass is growing just due to the substrate
uptake. To test the fuzzy integral controller
performance, it was enabled when 2 hours of
fermentation elapsed time was accomplished.
5 CONCLUSIONS
Based on the idea of splitting a continuous baking
yeast model, a TS fuzzy model was proposed using
the sector nonlinearities method, giving an exact
representation of the original nonlinear plant.
Moreover, a controller for each partial model was
constructed. It is worth noting that the controller was
capable to force the switching along the partial
models. Therefore, the approach presented here may
be considered a valid method to design a controller.
ACKNOWLEDGEMENTS
This paper has been supported by the Mexican
Consejo Nacional de Ciencia y Tecnología
(CONACyT), under grants 46538, 41148 and the
Ph.D. Schoolarship 70662.
REFERENCES
Ferreira, E., 1995. Identificação e controlo adaptivo de
processos biotecnológicos. Ph. D Thesis, Universidade
do Porto, Portugal.
Khalil, H. 1996. Nonlinear Systems, second edition.
Prentice Hall. United States of America.
Pormeleau, Y., 1990. Modelisation et controle d’un
procéde fed-batch de culture des levures á pain
(saccharomyces cerevisiae). Ph. D. Thesis. Ecole
Polytechnique de Montréal, Canada.
Takagi, T., Sugeno, M., 1985. Fuzzy identification of
systems and its applications to modelling and control.
IEEE Trans. Sys, Man Cyber, 15, 116-132.
Tanaka, K., Wang, H., 2001. Fuzzy control systems
design and analysis, a linear matrix inequality
approach. John Wiley & Sons, United States of
America.
Yamuna, R., Ramachandra, R., 1999. Control of
fermenters - a review. Bioprocess Engineering, 21, 77-
88.
TAKAGI-SUGENO MULTIPLE-MODEL CONTROLLER FOR A CONTINUOUS BAKING YEAST FERMENTATION
PROCESS
439