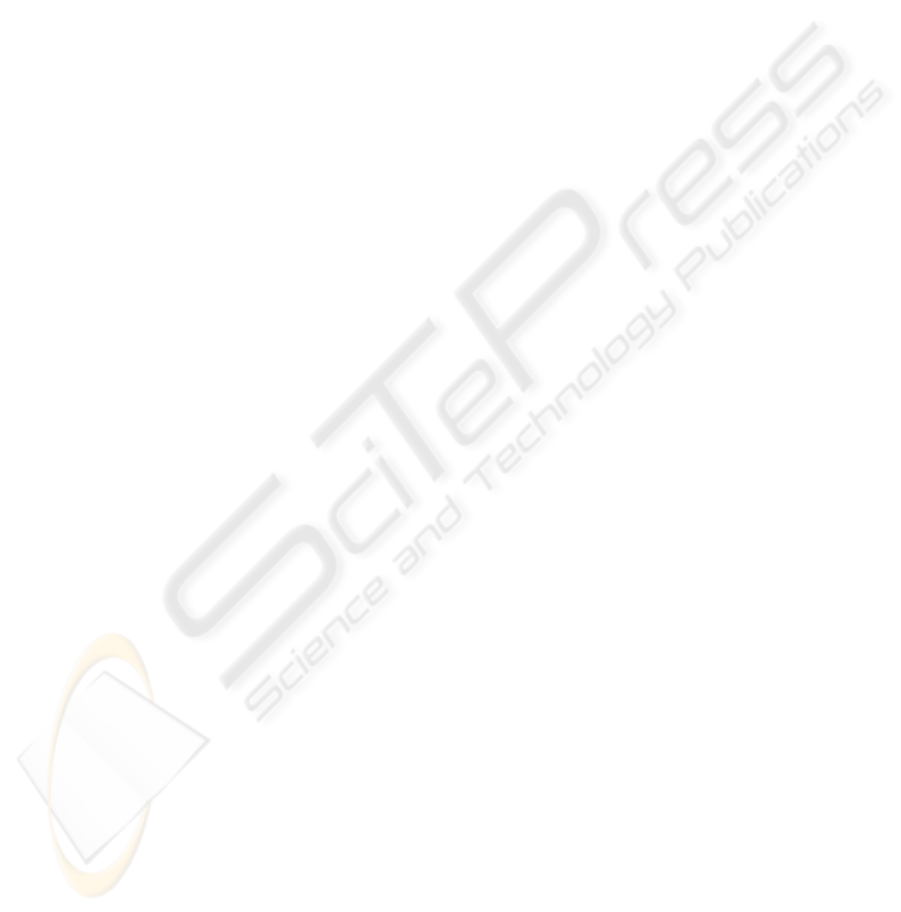
performance. Transfer functions of different units can be different and decided
automatically by an evolutionary process, instead of assigned by human experts. In
general, nodes within a group, like layer, in an artificial neural network tend to have
the same type of transfer function with possible difference in some parameters, while
different groups of nodes might have different types of transfer function.
References
1. Liu, Y. and Yao, X. “Evolutionary design of artificial neural networks with different
nodes”. In Proc. 1996 IEEE Int. Conf. Evolutionary Computation (ICEC’96), Nagoya,
Japan, pp. 670–675.
2. Fogel, D. B., Evolutionary Computation: Toward a New Philosophy of Machine
Intelligence. New York: IEEE Press, 1995.
3. Bäck, T. Hammel, U.and Schwefel, H.-P.“Evolutionary computation: Comments on the
history and current state,” IEEE Trans. Evolutionary Computation, vol. 1, pp. 3–17, Apr.
1997.
4. Mani, G. “Learning by gradient descent in function space”. In Proc. IEEE Int. Conf.
System, Man, and Cybernetics, Los Angeles, CA, 1990, pp. 242–247.
5. Lovell D. R. and Tsoi, A. C. The Performance of the Neocognitron with Various S-Cell and
C-Cell Transfer Functions, Intell. Machines Lab., Dep. Elect. Eng., Univ. Queensland,
Tech. Rep., Apr. 1992.
6. Stork, D. G. Walker, S. Burns, M. and Jackson, B. “Preadaptation in neural circuits”. In
Proc. Int. Joint Conf. Neural Networks, vol. I, Washington, DC, 1990, pp. 202–205.
7. White D. and Ligomenides, P. “GANNet: A genetic algorithm for optimizing topology and
weights in neural network design”. In Proc. Int. Workshop Artificial Neural Networks
(IWANN’93), Lecture Notes in Computer Science, vol. 686. Berlin, Germany: Springer-
Verlag, 1993, pp. 322–327.
8. Hwang, M. W. Choi, J. Y. and Park, J. “Evolutionary projection neural networks”. In Proc.
1997 IEEE Int. Conf. Evolutionary Computation, ICEC’97, pp. 667–671.
9. Sebald, A. V. and Chellapilla, K. “On making problems evolutionarily friendly, part I:
Evolving the most convenient representations”. In Porto, V. W., Saravanan, N., Waagen, D.
and Eiben, A. E. (Eds.): Evolutionary Programming VII: Proc. 7th Annu Conf.
Evolutionary Programming, vol. 1447 of Lecture Notes in Computer Science, Berlin,
Germany: Springer-Verlag, 1998, pp. 271–280.
10. Volná E. “Evolution design of an artificial neural network with fixed topology”, In R.
Matoušek, P. Ošmera (eds.): Proceedings of the 12th International Conference on Soft
Computing, Mendel'06, Brno, Czech Republic, 2006, pp. 1-6.
11. Lawrence, D. Handbook of genetic algorithms. Van Nostrand Reinhold, New York 1991.
12. Volná, E. „Using Neural network in cryptography“. In P. Sinčák, J. Vaščák, V. Kvasnička,
R. Mesiar (eds.): The State of the Art in Computational Intelligence. Physica-Verlag
Heidelberg 2000. pp.262-267.
13.
Garfinger, S. PGP: Pretty Good Privanci. Computer Press, Praha 1998.
14. Volná, E. „Learning algorithm which learns both architectures and weights of feedforward
neural networks“. Neural Network World. Int. Journal on Neural & Mass-Parallel Comp.
and Inf. Systems. 8 (6): 653-664, 1998.
20