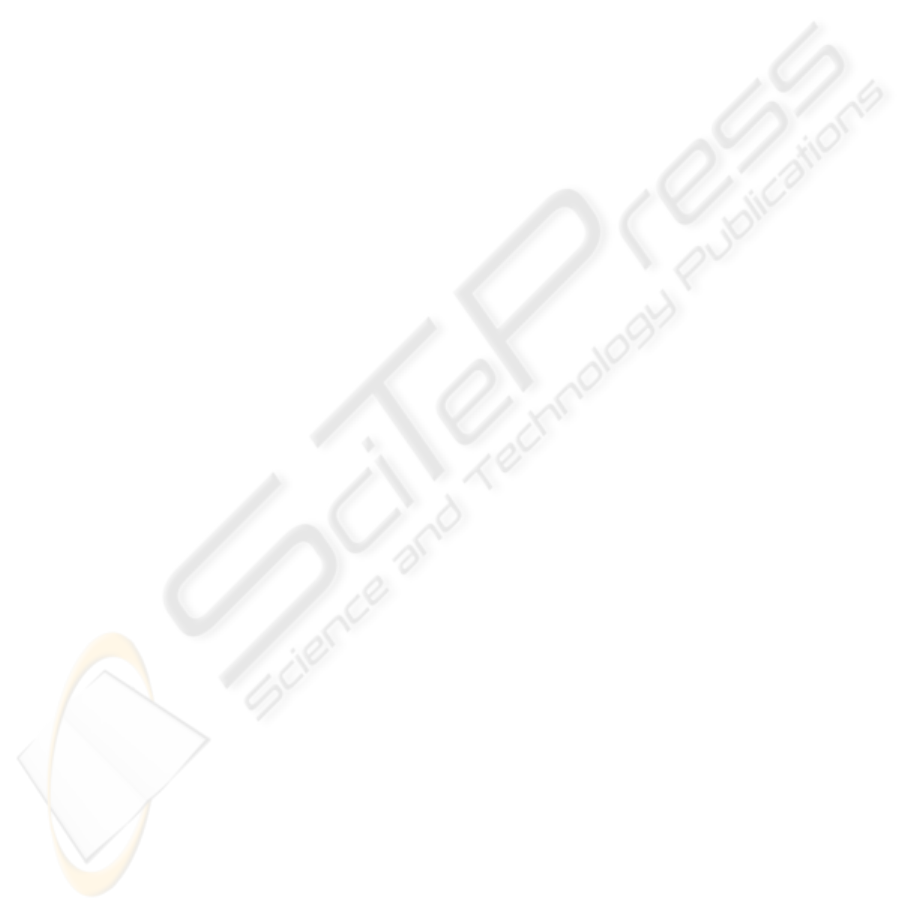
fault f
1
6
. This fault, in majority of cases, was classi-
fied as the normal operating conditions, thus it cannot
be either detected or isolated properly.
6.4 Fault Identification
In this work, the objective of fault identification was
to estimate the size of detected and isolated faults.
The sizes of faults were calculated using (23). The
results are shown in the last column of Table 2. Ana-
lyzing results, one can observe that quite large values
were obtained for the faults f
2
1
, f
2
2
and f
2
3
. That
is the reason that for these three faults true detection
rate and recognition accuracy had maximum or close
to maximum values. Another group is formed by the
faults f
1
1
, f
1
2
and f
2
4
possessing the similar values
of the fault size. The third group of fault consists of
f
1
3
, f
1
4
, and f
1
5
. The fault sizes in these cases are
distinctly smaller than in the cases already discussed.
Also the detection time t
dt
is relatively longer. In spite
of the fact that f
1
6
was not detected, the size of this
fault was also calculated. As one can see the fault size
in this case is very small, what explains the problems
with its detection and isolation.
7 FINAL REMARKS
In the paper, the neural network based method for the
fault detection and isolation of faults in a DC motor
was proposed. Using the novel structure of dynamic
neural network, quite accurate model of the motor
was obtained what rendered it possible detection, iso-
lation or even identification of faults. The approach
was successfully tested on a number of faulty scenar-
ios simulated in the real plant. The achieved results
confirm usefullness and effectiveness of neural net-
works in designing fault detection and isolation sys-
tems. It should be pointed out that presented solution
can be easily applied to on-line fault diagnosis.
ACKNOWLEDGEMENTS
This work was supported in part by the Ministry of
Science and Higher Education in Poland under the
grant Artificial Neural Networks in Robust Diagnostic
Systems
G. Głowacki is a stypendist of the Integrated Re-
gional Operational Programme (Measure 2.6: Re-
gional innovation strategies and the transfer of knowl-
edge) co-financed from the European Social Fund.
REFERENCES
Bell, A. J. and Sejnowski, T. J. (1995). An information-
maximization approach to blind separation and blind
deconvolution. Neural computation, 7:1129–1159.
Frank, P. M. and K
¨
oppen-Seliger, B. (1997). New devel-
opments using AI in fault diagnosis. Artificial Intelli-
gence, 10(1):3–14.
Fuessel, D. and Isermann, R. (2000). Hierarchical motor
diagnosis utilising structural knowledge and a self-
learning neuro-fuzzy scheme. IEEE Trans. Industrial
Electronics, 47:1070–1077.
Haykin, S. (1999). Neural Networks. A comprehensive
foundation, 2nd Edition. Prentice-Hall, New Jersey.
Korbicz, J., Ko
´
scielny, J. M., Kowalczuk, Z., and Cholewa,
W., editors (2004). Fault Diagnosis. Models, Artificial
Intelligence, Applications. Springer-Verlag, Berlin.
Moseler, O. and Isermann, R. (2000). Application of model-
based fault detection to a brushless dc motor. IEEE
Trans. Industrial Electronics, 47:1015–1020.
Patan, K. (2007). Approximation ability of a class of locally
recurrent globally feedforward neural networks. In
Proc. European Control Conference, ECC 2007, Kos,
Greece. accepted.
Patan, K. and Parisini, T. (2005). Identification of neural
dynamic models for fault detection and isolation: the
case of a real sugar evaporation process. Journal of
Process Control, 15:67–79.
Patton, R. J., Frank, P. M., and Clark, R. (2000). Issues
of Fault Diagnosis fo r Dynamic Systems. Springer-
Verlag, Berlin.
Roth, Z. and Baram, Y. (1996). Multidimensional density
shaping by sigmoids. IEEE Trans. Neural Networks,
7(5):1291–1298.
Walter, E. and Pronzato, L. (1996). Identification of Para-
metric Models from Experimental Data. Springer,
London.
Xiang-Qun, L. and Zhang, H. Y. (2000). Fault detection
and diagnosis of permanent-magnet dc motor based
on parameter estimation and neural network. IEEE
Trans. Industrial Electronics, 47:1021–1030.
Zhang, J., P. D. R. and Ellis, J. E. (1991). A self-learning
fault diagnosis system. Transactions of the Institute of
Measurements and Control, 13:29–35.
ICINCO 2007 - International Conference on Informatics in Control, Automation and Robotics
18