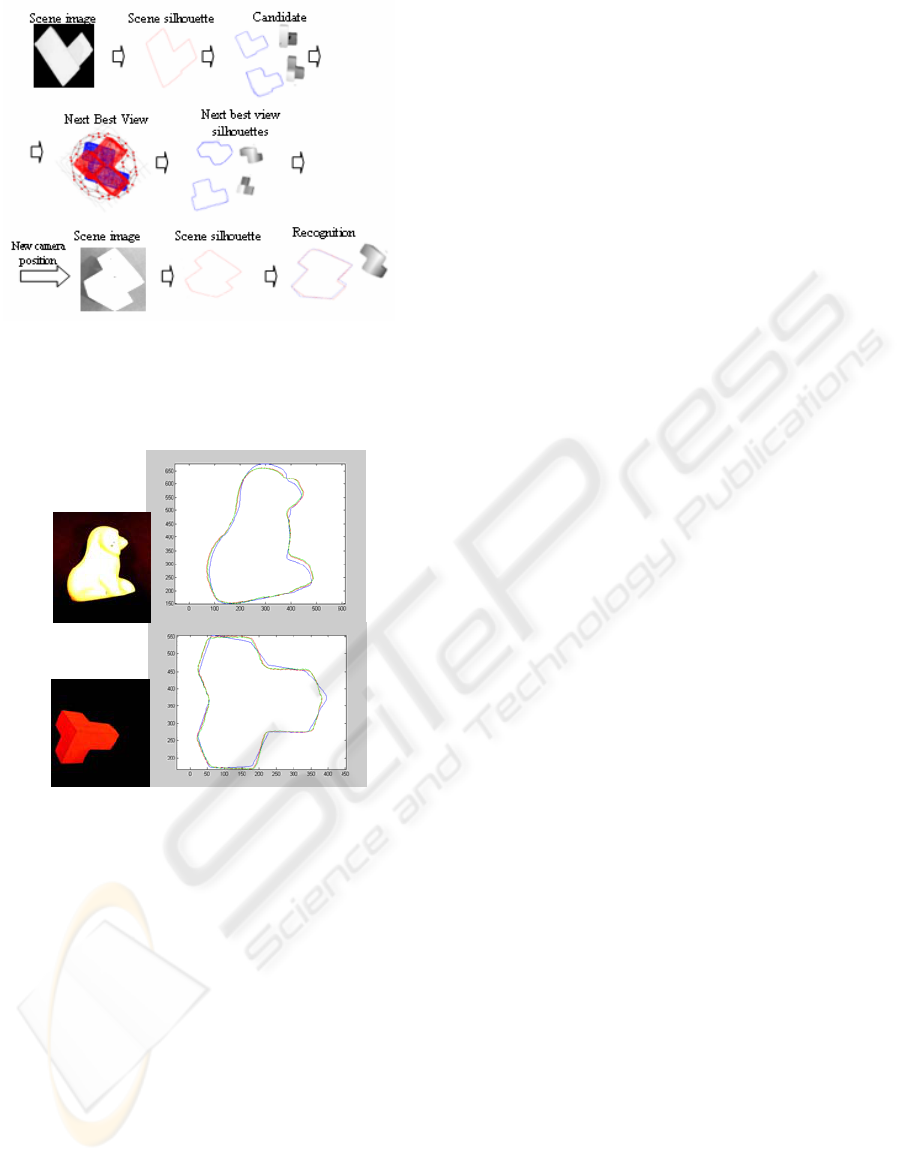
Figure 9: Solving an ambiguous case.
Figure 10 presents some matching and pose
estimation results using the proposed algorithm.
Figure 10: Some examples of 3D recognition without
ambiguity.
6 CONCLUSION
This paper has presented a new active recognition
system. The system turns a 3D object recognition
problem into a multiple silhouette recognition
problem where images of the same object from
multiple viewpoints are considered. Fourier
descriptors properties have been used to carry out
the clustering, matching and pose processes.
Our method implies the use of databases with a
very large number of stored silhouettes, but an
efficient version of the matching process with
Fourier descriptors make it possible to solve the
object recognition and pose estimation problems in a
greatly reduced computation time.
On the other hand, the next best view (NBV)
method efficiently solves the frequent ambiguity
problem in recognition systems. This method is very
robust and fast, and is able to discriminate among
very close silhouettes.
REFERENCES
Adán, A., Cerrada, C., Feliu, V., 2000. Modeling Wave
Set: Definition and Application of a New Topological
Organization For 3D Object Modeling. Computer
Vision and Image Understanding 79, pp 281-307.
Bustos, B., Kein, D.A., Saupe, D., Schreck, T., Vranic, D.,
2005. Feature-based Similarity Search in 3D Object
Databases. ACM Computing Surveys (CSUR)
37(4):345-387, Association For Computing
Machinery.
Borotschnig, H. Paletta, L., Pranti, M. and Pinz, A. H.
1999. A comparison of probabilistic, possibilistic and
evidence theoretic fusion schemes for active object
recognition. Computing, 62:293–319.
Deinzer, F., Denzler, J. and Niemann, H., 2003. Viewpoint
Selection. Planning Optimal Sequences of Views for
Object Recognition. In Computer Analysis of Images
and Patterns, pages 65-73, Groningen, Netherlands,
Springer.
Deinzer, F., Denzler, J., Derichs, C., Niemann, H., 2006:
Integrated Viewpoint Fusion and Viewpoint Selection
for Optimal Object Recognition. In: Chanteler, M.J. ;
Trucco, E. ; Fisher, R.B. (Eds.) : British Machine
Vision Conference
Helmer S. and Lowe D. G, 2004. Object Class
Recognition with Many Local Features. In Workshop
on Generative Model Based Vision 2004 (GMBV),
July.
Hutchinson S.A. and Kak. A.C., 1992. Multisensor
Strategies Using Dempster-Shafer Belief
Accumulation. In M.A. Abidi and R.C. Gonzalez,
editors, Data Fusion in Robotics and Machine
Intelligence, chapter 4, pages 165–209. Academic
Press,.
Netanyahu, N. S., Piatko, C., Silverman, R., Kanungo, T.,
Mount, D. M. and Wu, Y., 2002. An efficient k-means
clustering algorithm: Analysis and implementation.
vol 24, pages 881–892, july.
Niku S. B., 2001. Introduction to Robotics, Analysis,
Systems, Applications. Prentice Hall.
Poppe, R.W. and Poel, M., 2005. Example-based pose
estimation in monocular images using compact fourier
descriptors. CTIT Technical Report series TR-CTIT-
05-49 Centre for Telematics and Information
Technology, University of Twente, Enschede. ISSN
1381-3625
Roy, S.D., Chaudhury, S. and Banerjee. S., 2004. Active
recognition through next view planning: a survey.
Pattern Recognition 37(3):429–446, March.
Sipe M. and Casasent, D., 2002. Feature space trajectory
methods for active computer vision. IEEE Trans
PAMI, Vol. 24, pp. 1634-1643, December.
ACTIVE 3D RECOGNITION SYSTEM BASED ON FOURIER DESCRIPTORS
325