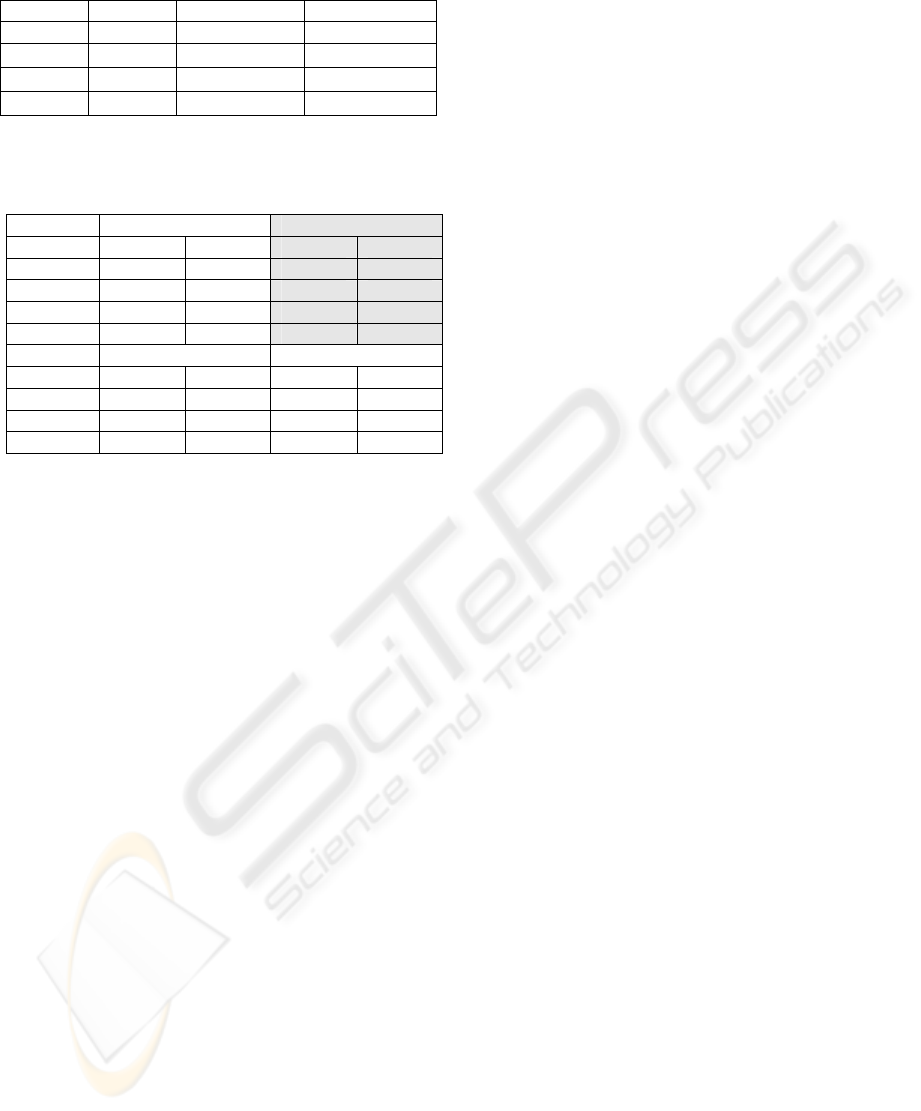
Table 1: Data Sets used for the test.
Data set Classes DIM Per Class
XOR 2 5 {400,400}
Spiral
2 2 {970,970}
Pima
2 8 {268,500}
LBC
2 9 {218,68}
Table 2: Comparison between IFPM, FPM, KNN and
FKNN, using leave-one-out technique, according to
Rejection Rate (RR) and to Misclassification Rate (MR).
Method FPM
IFPM
Criterion
MR (%) RR (%)
MR (%) RR (%)
XOR 44.12 0 0 0
Spiral 18.24 0 0 1.5
Pima 30.73 0.78 19.53 20.7
LBC 25.87 1.05 10.14 30.42
Method KNN FKNN
XOR 0 0 0 0
Spiral 0 0 0 0
Pima 25.26 0 26.43 0
LBC 23.78 0 23.43 0
6 CONCLUSIONS
In this paper, we have proposed a solution to adapt
the classification method Fuzzy Pattern Matching
(FPM) to be operant in the case of classes with
correlated attributes as well as the class importance
and its shape if this shape is not convex. The
integration of this solution in FPM is called
Improved FPM (IFPM). The performances of IFPM
are compared with the ones of FPM, K Nearest
Neighbours (KNN) and Fuzzy KNN (FKNN) using
the misclassification rate as evaluation criterion.
This comparison is realized according to four data
sets. We have also used XOR problem and Spiral
data which are widely used to study the correlation
between attributes. In addition, we have used Pima
Indians diabetes and Ljubljana Breast Cancer data
sets which are known to be strongly non Gaussian
with different a priori probabilities. The
misclassification rate obtained by IFPM is better
than the one of FPM, KNN and FKNN. However,
IFPM rejects more points than the previous methods.
Anyway, it is better to reject a point than to
misclassify it.
REFERENCES
Cadenas, J.M., M.C. Garrido and J.J. Hernandez, 2004.
Improving fuzzy pattern matching techniques to deal
with non discrimination ability features. In: IEEE
International Conference on Systems, Man and
Cybernetics, pp. 5708-5713.
Denoeux, T., M. Masson and B. Dubuisson, 1998.
Advanced pattern recognition techniques for system
monitoring and diagnosis: a survey. In: Journal
Européen des Systèmes Automatisés (RAIRO-APII-
JESA), Vol. 31(9-10), pp. 1509-1539.
Denoeux, T. and L.M. Zouhal, 2001. Handling
possibilistic labels in pattern classification using
evidential reasoning. In: Fuzzy Sets and Systems, Vol.
1(22), pp. 409-424.
Devillez, A., 2004a. Four supervised classification
methods for discriminating classes of non convex
shape. In: Fuzzy sets and systems, Vol. 141, pp. 219-
240.
Devillez, A., M.Sayed Mouchaweh and P. Billaudel,
2004b. A process monitoring module based on fuzzy
logic and Pattern Recognition. In: International
Journal of Approximate Reasoning, Vol. 37, Issue 1,
pp.43-70.
Dubois, D. and H. Prade, 1993. On possibility/probability
transformations, In: Fuzzy Logic, pp. 103-112.
Duda, R.O., P.E. Hart and D.E. Stork, 2001. Pattern
Classification second edition. Wiley, New York
Dubuisson, B., 2001. Automatique et statistiques pour le
diagnostic. Traité IC2 Information, commande,
communication. Hermes Sciences, Paris.
Dubuisson, B., 1990. Diagnostic et reconnaissance des
formes, Traité des Nouvelles Technologies, série
Diagnostic et Maintenance, Hermes Sciences, Paris.
Grabish, M. and M. Sugeno, 1992. Multi-attribute
classification using fuzzy integral. In: Proc. of fuzzy
IEEE, pp. 47-54.
Medasani, S., K. Jaeseok and R. Krishnapuram, 1998, An
overview of membership function generation
techniques for pattern recognition. In: International
Journal of Approximate Reasoning,Vol. 19,pp. 391-
417.
Newman D.J., Hettich, S., Blake C.L. and Merz, C.J,
1998. UCI Repository of machine learning databases,
http://www.ics.uci.edu/~mlearn/MLRepository.html,
Dept. of Information and Computer Science,
University of California, Irvine.
Ripley, B.D.,1996. Pattern Recognition and Neural
Networks. Cambridge University Press, Cambridge.
Sayed Mouchaweh, M., A. Devillez, G.V. Lecolier and P.
Billaudel, 2002a. Incremental learning in real time
using fuzzy pattern matching. In: Fuzzy Sets and
Systems, Vol. 132/1, pp. 49-62.
Sayed Mouchaweh, M. and P. Billaudel ,2002b. Influence
of the choice of histogram parameters at Fuzzy Pattern
Matching performance. In: WSEAS Transactions on
Systems, Vol. 1, Issue 2, pp. 260-266
Sayed Mouchaweh, M., 2004. Diagnosis in real time for
evolutionary processes in using Pattern Recognition
and Possibility theory. In: International Journal of
Computational Cognition 2, Vol. 1, pp. 79-112.
Zadeh, L. A., 1978. Fuzzy sets as a basis for a theory of
possibility. In: Fuzzy sets and systems, Vol. 1, pp. 3-2.
ICINCO 2007 - International Conference on Informatics in Control, Automation and Robotics
172