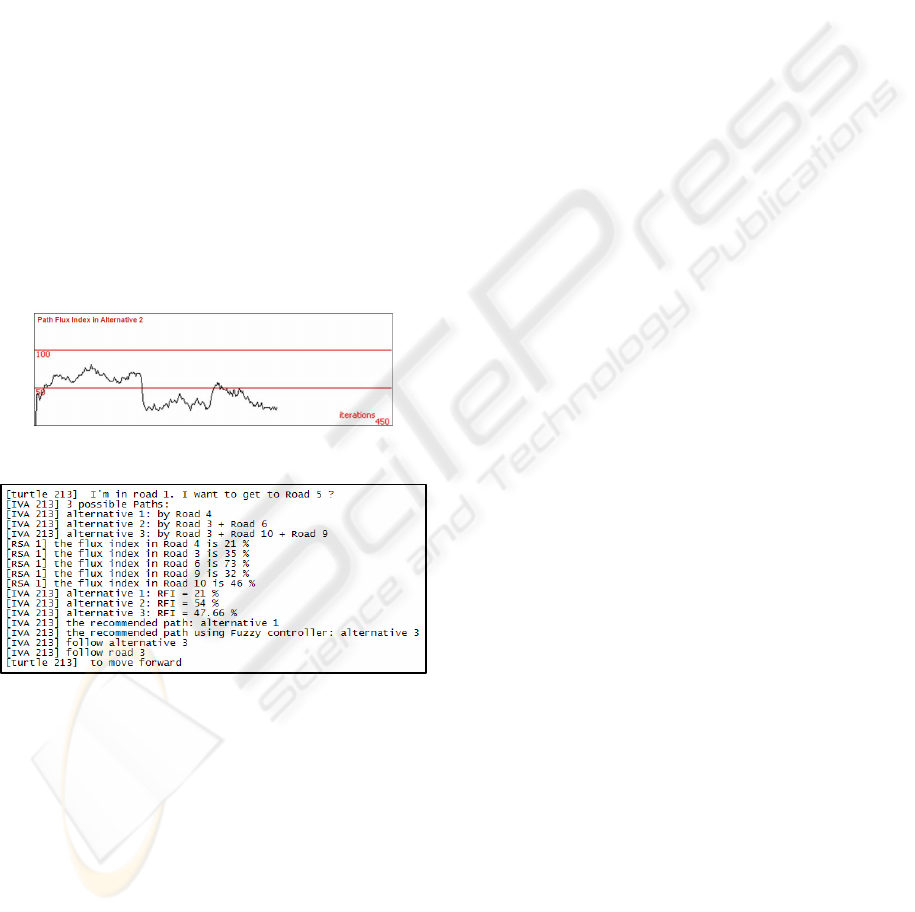
the traffic load intensity to be forwarded from road 1
to road 5. Figure 5 shows the road flux index in the
different alternatives from road number 1 to road
number 5. The IVA chooses the first alternative (by
road 4) because it has the smallest flux index
compared with second and third alternatives. The
flux index in the second alternative is high because
of jam situation in road 6. The flux index in the third
alternative is high because of the route length. The
RFI was from 0 to 100 %. The simulation has been
done every 450 seconds when updating the road flux
index table after every 60 seconds.
Second series of simulations was performed
using the fuzzy rule base with the same parameters
of the first simulation. Figure 6 confirms that after
the work information, bad weather condition, and
driver’s unfamiliarity of the road 4, the controller
proposes the third alternative to follow.
Various other simulations are applied with other
maps, other positions of clutters, and different
criteria. The results show that the fuzzy logic
application for route choosing gives a better
management of road network in all cases.
Figure 5: Viewer of Path Traffic Index.
Figure 6: Example of communication messages between
IVA and RSA.
5 CONCLUSION
In this paper, we presented a hierarchical
architecture as well as a model and a simulation of
road supervision system based on joint fuzzy logic
and multiagent approach. The route choice algorithm
developed shows acceptable results, but it become
very complex if we add other criteria for route
choice make decision.
The originality of this model resides on:
A hierarchical fuzzy controller in the
multi-route choice problem.
Generic architecture, without limit for the
number of factors to use.
A hierarchical multiagent architecture handling
fuzzy inference for the route choice problem.
As perspectives, we intend in the near future to
add other options such as the factor of variant speed
for IAV, an advancement treatment of crossroads, an
environment with double way, and the change lane
problem. Applying learning methods such as (Kallel
et al., 2006) become a necessity in order to reduce
rule numbers and adjust membership functions.
Furthermore, paths learning and multiobjective
optimization of vehicle path planning can be added.
REFERENCES
Adler, J. L., Satapathy, G., Manikonda, V., Bowles, B., &
Blue, V. J., 2005. A multi-agent approach to
cooperative traffic management and route guidance.
Transportation Research Part B, 39(4), 297-318.
Alimi, A. M., 1997. A neuro-fuzzy approach to recognize
Arabic handwritten characters. In Proc. of the I. C. on
Neural Networks, vol. 3, 1397-1400.
Ferber, J., Michel, F. & Baez, J., 2005. AGRE: Integrating
environments with organizations. In Proc. of E4MAS,
Lecture Notes in Artificial Intelligence, 3374, 48-56.
Hallé, S., Chaib-Draa, B., 2005. A collaborative driving
system based on multiagent modelling and simulations.
Transportation Research Part C, 13, 320-345.
Kallel, I., Jmaiel, M., & Alimi, A. M., 2002. A Multi-
Agent Approach for Genetic Algorithm
Implementation. In Proc. of IEEE SMC, Tunisia.
Kallel, I., Jelleli, T. & Alimi, A. M., 2005. Hierarchical
FLS Design Using Multi-Agent Genetic Approach. In
Proc. of GFS’2005, Granada, Spain, 142-147.
Kallel, I., Alimi, A. M., 2006. MAGAD-BFS: A Learning
Method for Beta Fuzzy Systems based on a Multi-Agent
Genetic Algorithm. I. J. of Soft Computing, 10(9), 757-772.
Kammoun, H. M., Kallel, I., Alimi, A. M., 2005.
RoSMAS²: Road Supervision based Multi-Agent
System Simulation. In Proc. of the I. C. on Machine
Intelligence ACIDCA-ICMI’2005, Tunisia, 203-210.
Michel, F., Beurier, G., & Ferber, J., 2005. The TurtleKit
Simulation Platform: Application to Complex
Systems. In Proc. of SITIS’05, 122-127.
Teodorovic, D., 1999. Fuzzy logic systems for
transportation engineering: the state of the art.
Transportation Research Part A, 33(5), 337-364.
Wooldridge, M., 2002. An introduction to multiagent
systems, John Wiley and Sons. February 2002.
Zadeh, L., 1965. Fuzzy sets, Information and Control 8,
338-353.
A JOINT HIERARCHICAL FUZZY-MULTIAGENT MODEL DEALING WITH ROUTE CHOICE PROBLEM -
RoSFuzMAS
397